Edge-Computing FogFlow Framework For Solar Generation Prediction Exploiting Federated Learning
2023 International Conference on Modeling & E-Information Research, Artificial Learning and Digital Applications (ICMERALDA)(2023)
摘要
The integration of renewable energy sources into residential power systems, accompanied by the growth of edge computing, presents a dynamic landscape for energy management. In this paper, an innovative solution for solar generation forecasting is introduced, effectively addressing issues of data privacy, operational efficiency, scalability, and network optimization. A Federated Learning Gradient Boosting (FedXGB) framework integrated with edge computing is applied, significantly amplifying energy efficiency and sustainability in residential environments. Powered by FogFlow, the orchestration and optimization of IoT ecosystems, particularly within smart homes, are advanced, and data privacy is ensured. Remarkable results are observed, especially in mid-term and long-term solar forecasting, highlighting the framework's transformative potential in reshaping energy management. This innovation holds the promise of revolutionizing how renewable energy is harnessed within homes and communities, paving the way for a more sustainable and efficient future. With this comprehensive approach, the intersection of renewable energy and edge computing takes a significant step forward in enhancing energy management.
更多查看译文
关键词
FogFlow,Federated Learning,edge computing,Generation prediction
AI 理解论文
溯源树
样例
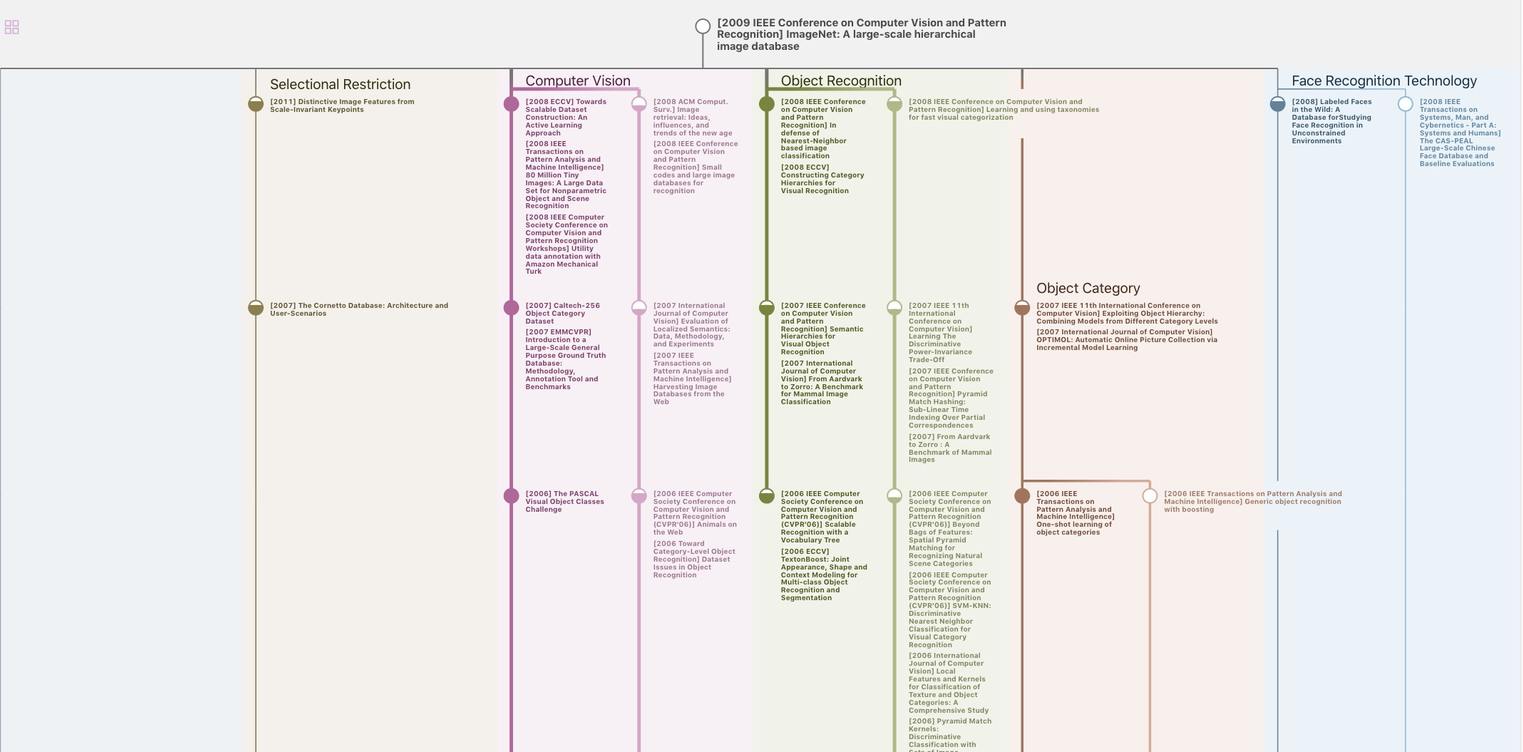
生成溯源树,研究论文发展脉络
Chat Paper
正在生成论文摘要