Contrastive Learning Based Fault Diagnosis of Motor with Multi-Modal Feature Fusion
2023 China Automation Congress (CAC)(2023)
摘要
In practical industrial environments, various types of data are generated by rotating motor faults, including bearing vibration signals, three-phase current signals, temperature signals, and more. These data possess different characteristics, making it impossible to comprehensively reflect the fault information solely relying on a single signal source. To address this issue, this paper proposes a multimodal feature fusion(MMF) method that integrates self-fusion and cross-fusion techniques, along with Simple Contrastive Learning(SIMCLR), for fault diagnosis of rotating motors. This method is referred to as SIMCLR-MMF. Firstly, different convolutional autoencoders with distinct structures are employed to extract features of different modalities from each signal source, facilitating the self-fusion of features. Additionally, contrastive learning techniques are incorporated to enhance the feature representation capability of each signal source. Finally, a Multi-head attention network framework is utilized to fuse the multimodal data and assign higher weights to important features. This framework enables the fusion of multiple modalities and improves the performance of fault diagnosis for rotating motors. The effectiveness of this method are demonstrated through validation on real-world rotating motor datasets.
更多查看译文
关键词
Multi-Modal Feature Fusion,Contrastive learning,Multi-head attention mechanism,Fault diagnosis,Motor
AI 理解论文
溯源树
样例
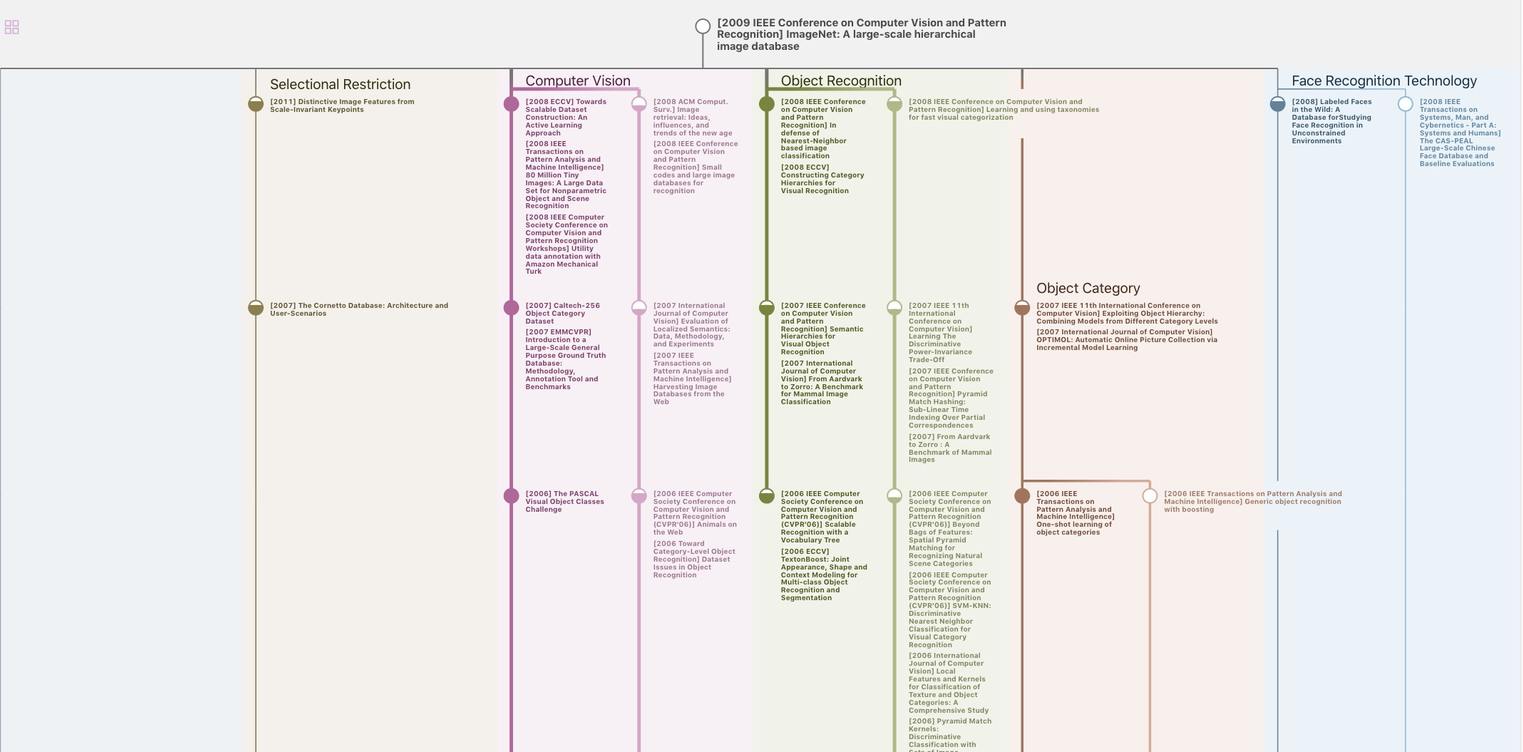
生成溯源树,研究论文发展脉络
Chat Paper
正在生成论文摘要