An Enhanced Network Intrusion Detection Method Using Auxiliary Classifier Generative Adversarial Networks
2023 China Automation Congress (CAC)(2023)
摘要
With the development of communication technology and the emerging concept of Internet of everything, the network is at risk of expanding the attack surface, and the associated network security issues have raised widespread concern. Although the intrusion detection system (IDS) based on machine learning has achieved great progress in anomaly detection, they are weak in processing imbalanced data. Machine learning models are unable to learn minority classes of cyber-attacks in imbalanced data, and thus cannot accurately distinguish malicious attacks. In this study, we propose a novel auxiliary classifier generative adversarial networks (ACGAN) that oversamples the raw data to solve the imbalance problem. we introduce an attention mechanism to extract important features in the attack traffic and add an auxiliary classifier to the original generative adversarial networks (GAN) structure, generating attack samples of the specified class. To verify the effectiveness of the proposed model, we perform a comprehensive evaluation on two datasets include NSL-KDD and UNSW-NB15, and compare ACGAN with synthetic minority oversampling technique (SMOTE). The experimental results demonstrate that our method can effectively generate minority class samples and improve the performance of classification.
更多查看译文
关键词
network security,machine learning,imbalanced data,intrusion detection system (IDS),auxiliary classifier gener-ative adversarial networks (ACGAN),oversampling
AI 理解论文
溯源树
样例
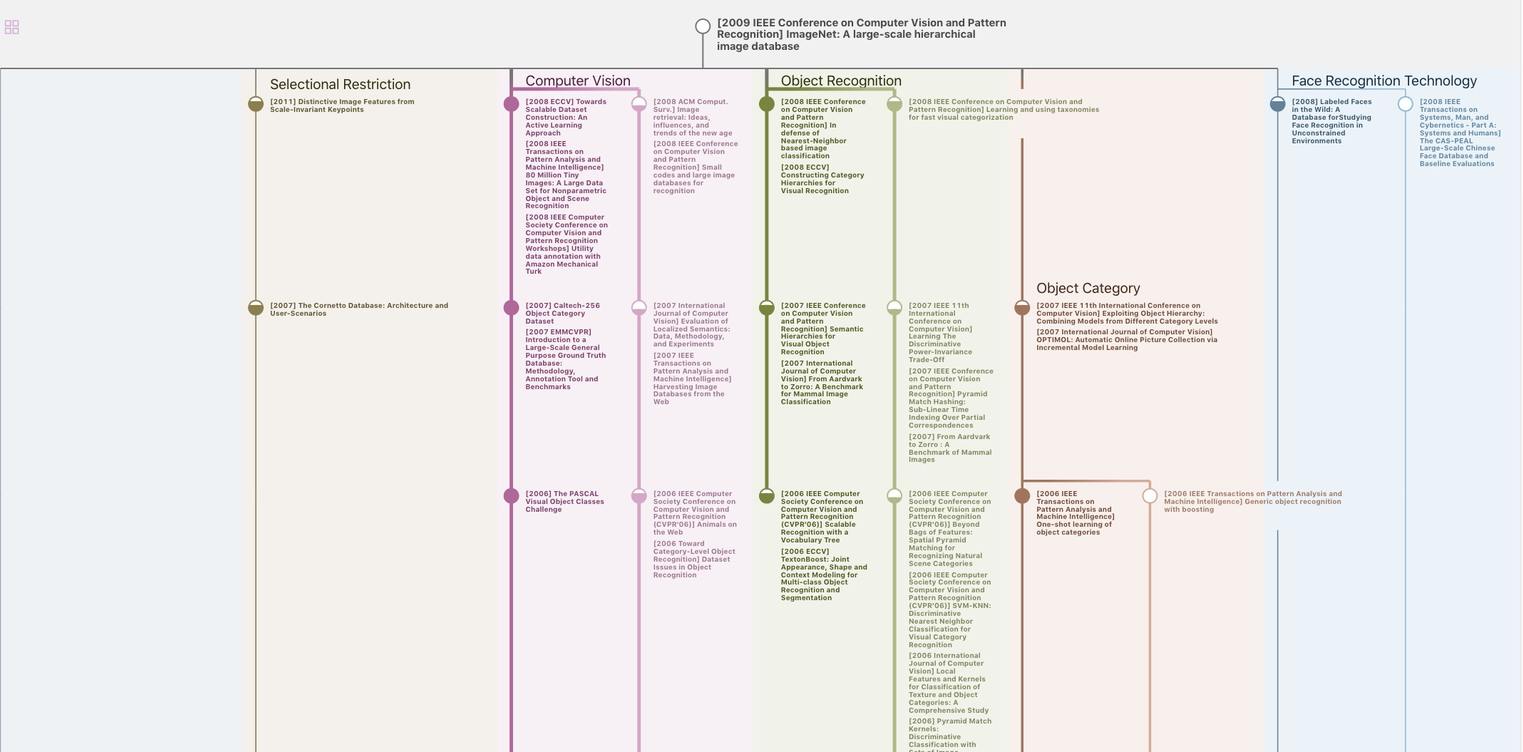
生成溯源树,研究论文发展脉络
Chat Paper
正在生成论文摘要