Representing Network Frontiers based on Spatio-temporal Graph Transformations
crossref(2024)
摘要
Abstract Purpose-Spatio-temporal prediction in cyber elements can be enhanced by incorporating geographic features into the estimation process for cyber elements. It highlights a deficiency in existing studies that do not incorporate geographic characteristics when estimating network security factors. Methodology-This methodology is then assessed through benchmark experiments using ARIMA and LSTM models, with the spatiotemporal graph convolution prediction model demonstrating superior forecasting performance based on evaluation criteria such as Mean Absolute Error (MAE), Root Mean Squared Error (RMSE), and Mean Absolute Percentage Error (MAPE). Findings-This leads to the creation of a spatiotemporal network vulnerability dataset, demonstrating the feasibility of integrating spatial and temporal dimensions into network vulnerability analysis. Research Limitations -The identified gap in studies integrating geographic characteristics in estimating network security factors suggests a need for further research in this area. Future studies could explore different approaches, datasets, and applications to build upon the current findings and contribute to the evolving field of spatio-temporal prediction in cyberspace. Practical Implications-The practical implications of incorporating geographic features and utilizing the proposed spatiotemporal graph convolution model include improved network security planning, enhanced predictive capabilities, informed data-driven decision-making, benchmarking against traditional models, adaptation to evolving threats, and resource optimization. These implications highlight the potential practical benefits for organizations seeking advanced and effective approaches to cybersecurity. Originality-The originality of incorporating geographic features, addressing a gap in the literature, introducing an innovative methodology, creating a unique spatiotemporal dataset, and proposing a sophisticated spatiotemporal graph convolution model with demonstrated superior forecasting performance. These contributions collectively advance the understanding and application of spatio-temporal prediction in the field of cyberspace security.
更多查看译文
AI 理解论文
溯源树
样例
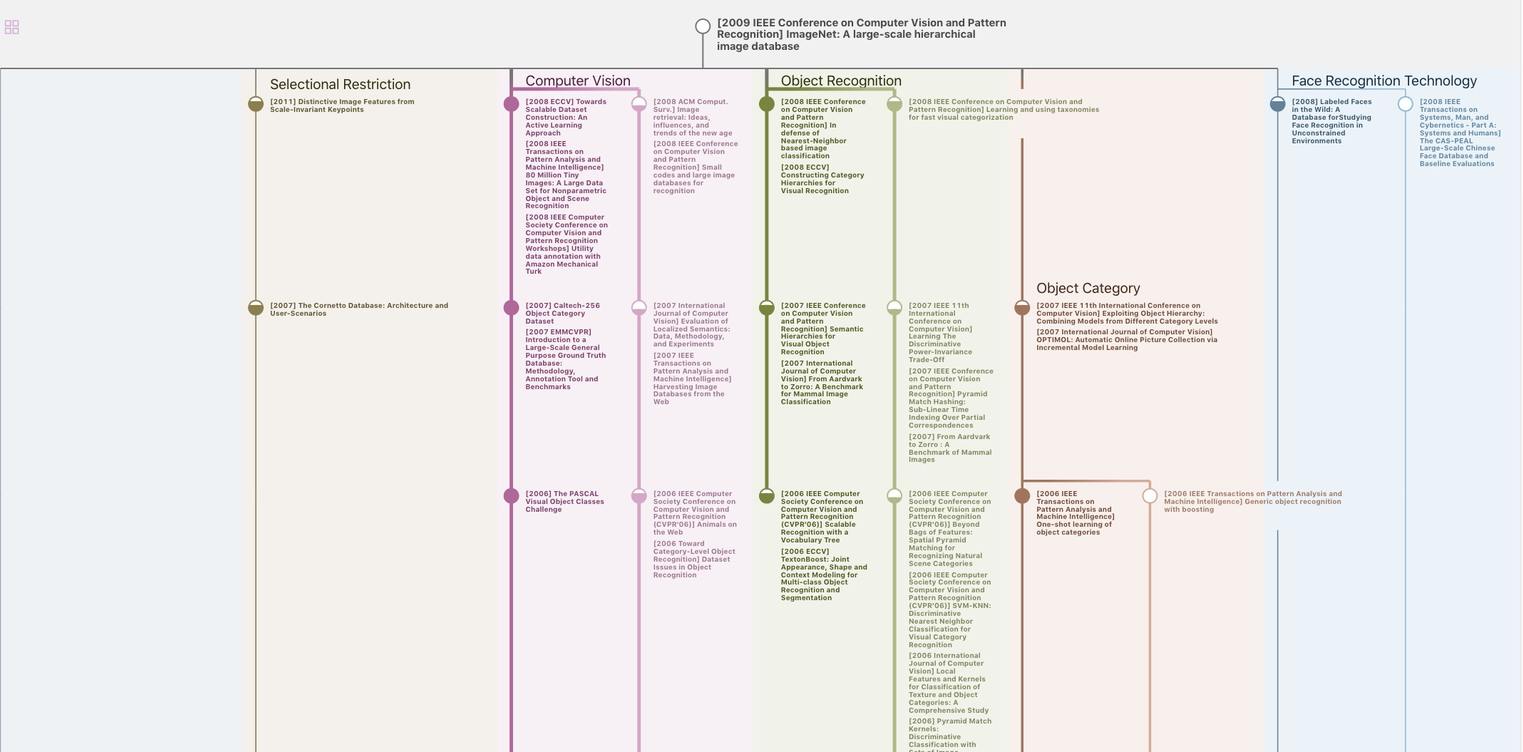
生成溯源树,研究论文发展脉络
Chat Paper
正在生成论文摘要