RSKD: Enhanced medical image segmentation via multi-layer, rank-sensitive knowledge distillation in Vision Transformer models
Knowledge-Based Systems(2024)
摘要
Medical image segmentation is crucial for enhancing diagnostic accuracy through pixel labeling. State-of-the-art networks, despite their performance, have high computational demands, limiting real-time use on constrained devices. Lightweight networks face challenges in balancing detail processing with precision. Vision Transformer models, while promising, also have computational concerns. This study presents a novel method that merges Vision Transformer strengths with a unique knowledge distillation technique. A pivotal element of our approach is the Token Importance Ranking Distillation, which facilitates the meticulous transfer of top-k token importance rankings between a complex teacher model and a simplified student model, guided by a specialized ranking loss function. This method is essential for optimizing the student model to effectively emulate the teacher model’s ability to encapsulate vital semantic and spatial information. Additionally, we introduce an innovative methodology in structural texture knowledge, utilizing a Contourlet Decomposition Module (CDM), which enriches the models with nuanced texture representation, crucial for extracting directional features and capturing intricate global and local contexts in medical imaging. Complementing this, we deploy a unique multi-stage distillation strategy, the Space Channel Cascade Fusion (SCCF), to refine both spatial and channel information concurrently, mitigating redundancy and enhancing representational effectiveness in feature maps. Experimental results demonstrate the effectiveness of our approach in elevating the performance of student models while diminishing computational demands, thereby enabling efficient, real-time medical image segmentation on resource-constrained devices.
更多查看译文
关键词
Knowledge distillation,Vision Transformer Models,Token Importance Ranking Distillation,Structural Texture Distillation,Multi-layer feature map distillation
AI 理解论文
溯源树
样例
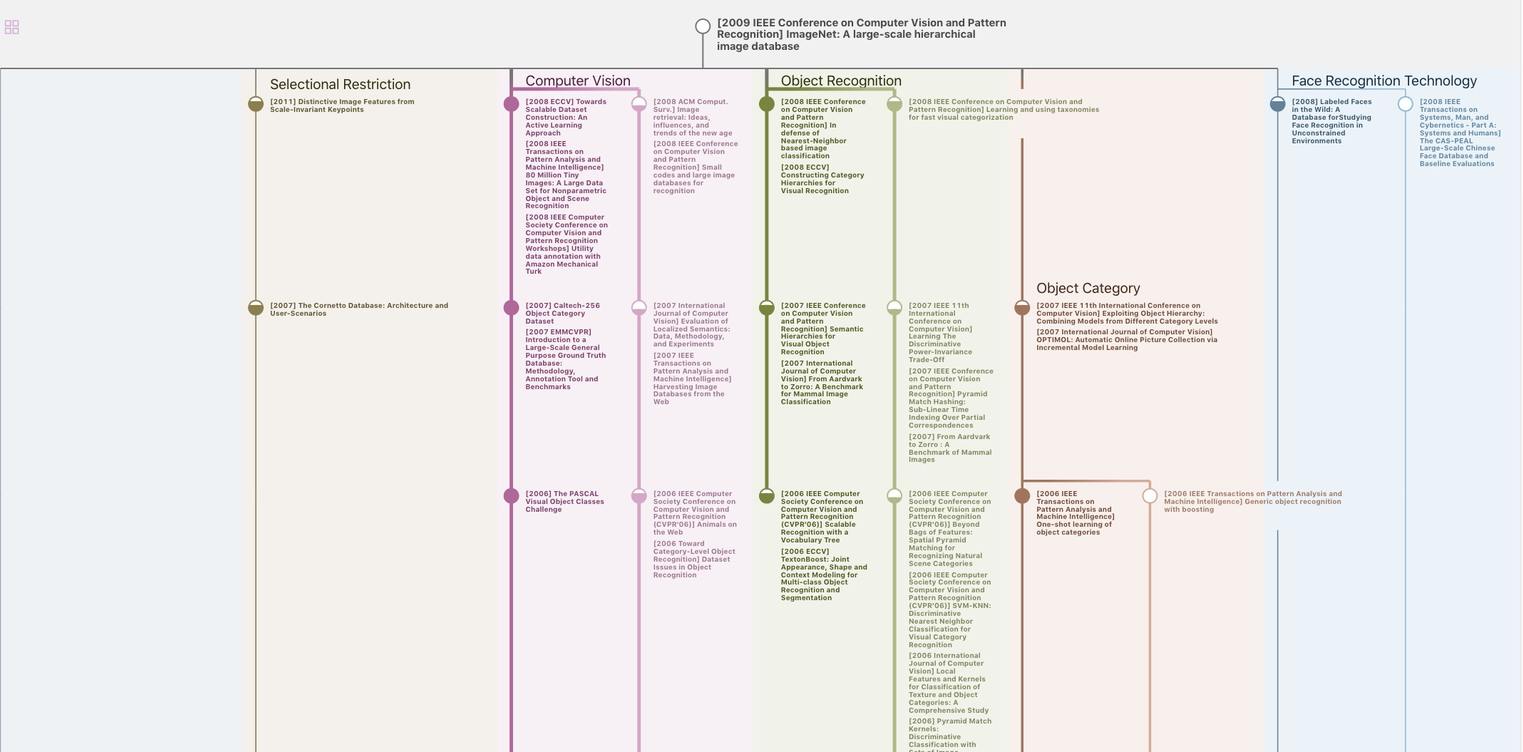
生成溯源树,研究论文发展脉络
Chat Paper
正在生成论文摘要