Using Machine Learning to Evaluate the Value of Genetic Liabilities in the Classification of Hypertension within the UK Biobank
JOURNAL OF CLINICAL MEDICINE(2024)
摘要
Background and Objective: Hypertension increases the risk of cardiovascular diseases (CVD) such as stroke, heart attack, heart failure, and kidney disease, contributing to global disease burden and premature mortality. Previous studies have utilized statistical and machine learning techniques to develop hypertension prediction models. Only a few have included genetic liabilities and evaluated their predictive values. This study aimed to develop an effective hypertension classification model and investigate the potential influence of genetic liability for multiple risk factors linked to CVD on hypertension risk using the random forest and the neural network. Materials and Methods: The study involved 244,718 European participants, who were divided into training and testing sets. Genetic liabilities were constructed using genetic variants associated with CVD risk factors obtained from genome-wide association studies (GWAS). Various combinations of machine learning models before and after feature selection were tested to develop the best classification model. The models were evaluated using area under the curve (AUC), calibration, and net reclassification improvement in the testing set. Results: The models without genetic liabilities achieved AUCs of 0.70 and 0.72 using the random forest and the neural network methods, respectively. Adding genetic liabilities improved the AUC for the random forest but not for the neural network. The best classification model was achieved when feature selection and classification were performed using random forest (AUC = 0.71, Spiegelhalter z score = 0.10, p-value = 0.92, calibration slope = 0.99). This model included genetic liabilities for total cholesterol and low-density lipoprotein (LDL). Conclusions: The study highlighted that incorporating genetic liabilities for lipids in a machine learning model may provide incremental value for hypertension classification beyond baseline characteristics.
更多查看译文
关键词
the receiver operation characteristic (ROC),area under the curve (AUC)
AI 理解论文
溯源树
样例
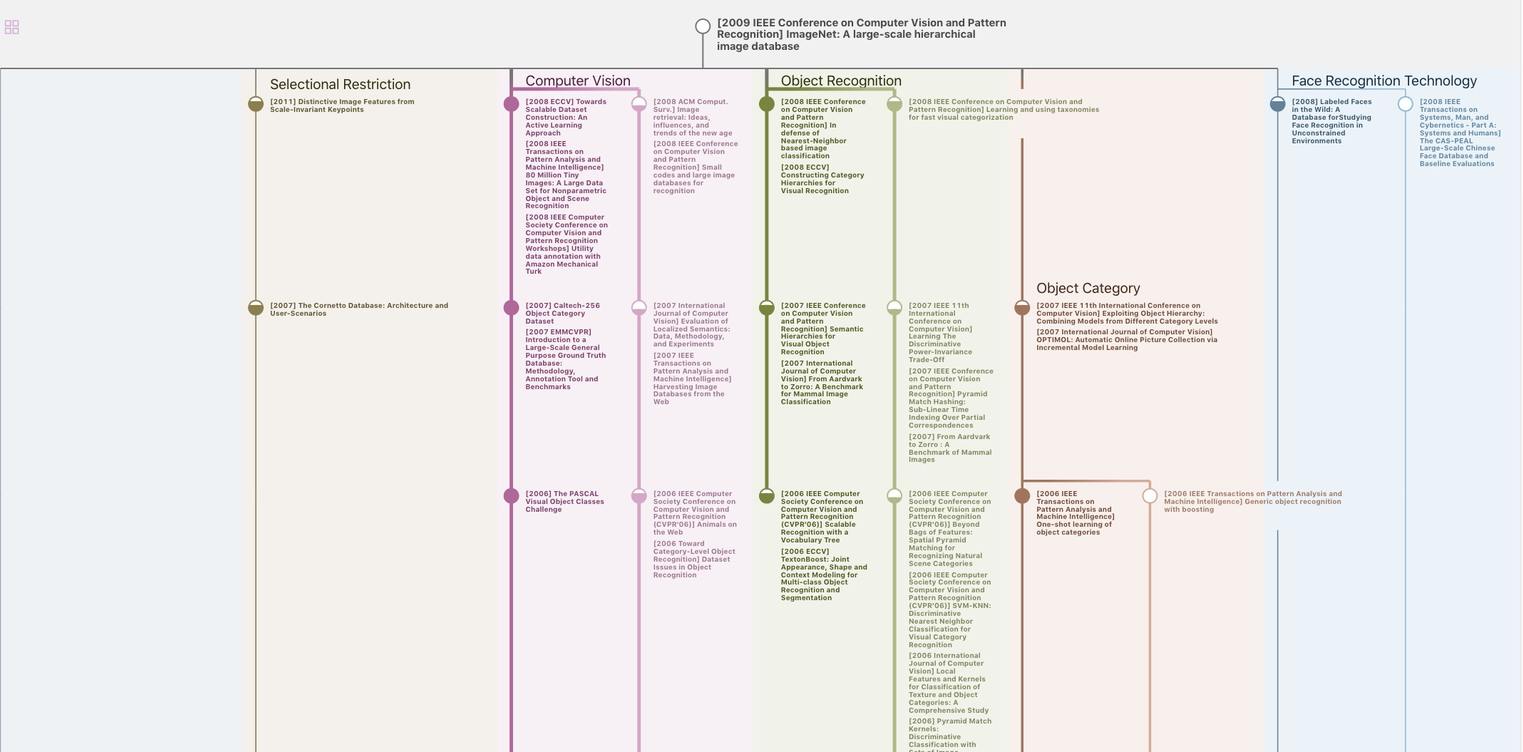
生成溯源树,研究论文发展脉络
Chat Paper
正在生成论文摘要