A Bayesian Hierarchical Analysis on the Disparity of Emergency Department Visits for COVID-19: A Cohort Study Using National COVID Cohort Collaborative (N3C) Data.
International Conference on Machine Learning and Applications(2023)
摘要
Since the COVID-19 pandemic in 2020, there are numerous studies and researches on the long term effect of COVID-19 on both patient level and social level with disparities noted in infection rates and outcomes. However, differences in the COVID related healthcare decisions after patients present for care (e.g. hospitalization after emergency department visit) has not been studied much at a national level. The National COVID Cohort Collaborative (N3C) provides researchers with abundant data collected from different clinical sites, making it suitable for Bayesian hierarchical modeling while analyzing the disparity in hospitalization after emergency department visit, where prior information or belief could be easily included in the modeling process by adjusting the prior distribution of parameters. In this analysis, we select demographic information (age, sex, race and ethnicity) and the Charlson Comorbidity Index (CCI) as features and study the relationship between these features and whether a patient would be hospitalized after having a COVID related visit to an emergency department (ED).
更多查看译文
关键词
Bayesian analysis,hierarchical modeling,generalized linear model,disparity,COVID-19 related healthcare
AI 理解论文
溯源树
样例
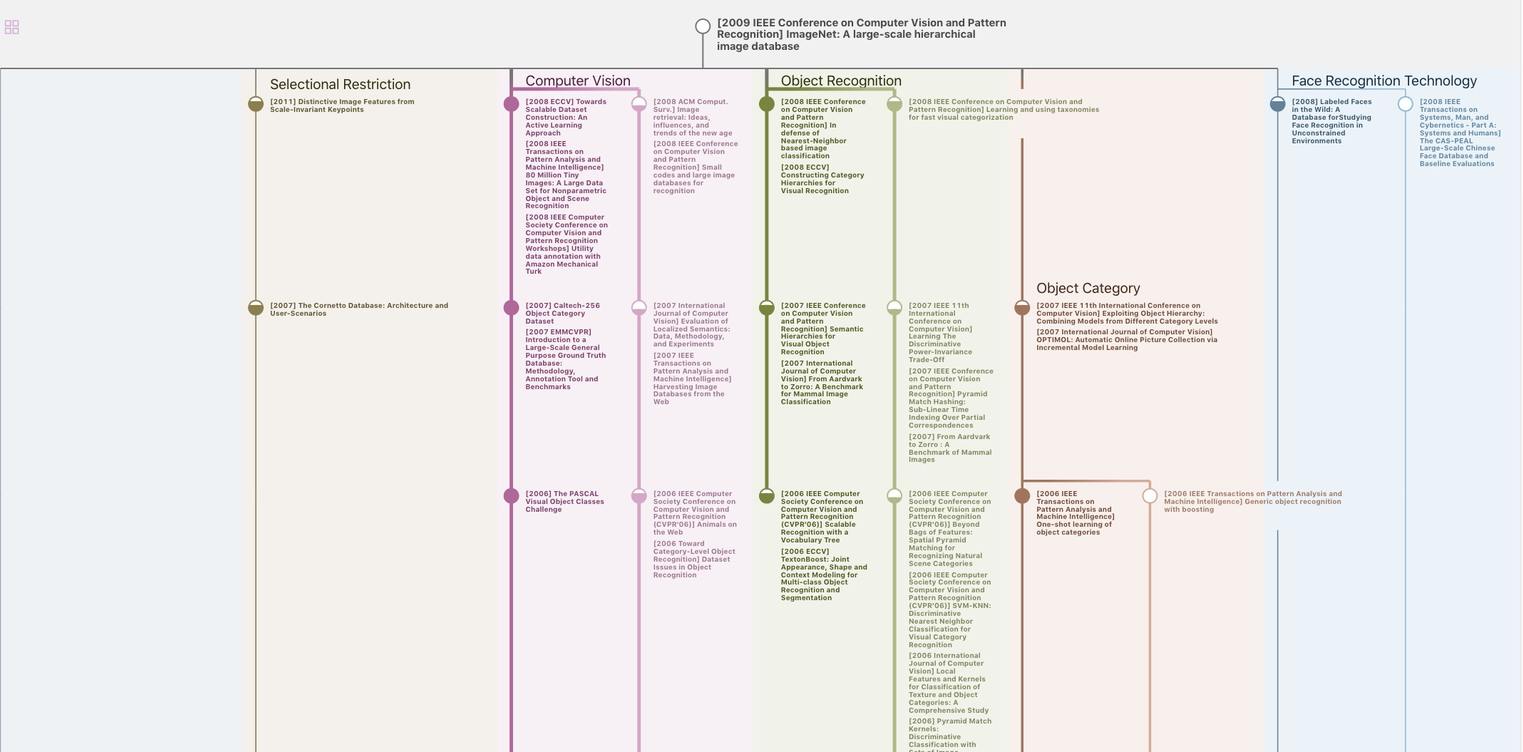
生成溯源树,研究论文发展脉络
Chat Paper
正在生成论文摘要