Learning Discriminative Feature Representations via Metric Learning for Early Operation of Wind Turbine Anomaly Detection Systems.
International Conference on Machine Learning and Applications(2023)
摘要
To achieve robust wind turbine anomaly detection, we attempted to incorporate metric learning into the process of learning discriminative feature representations. In the context of anomaly detection based on inlier modeling, where anomalies are identified as inputs deviating from the normal state distribution, the key to building a high-performance and robust system, even with limited training data, lies in eliminating the influence of environmental differences from the inputs and obtaining a compact normal state distribution. To address this, we designed a deep neural network-based feature extractor to mitigate the impact of environmental changes. Additionally, we integrated metric learning into its learning process to obtain a more compact distribution by embedding inputs with similar properties close to one another. We validated the effectiveness of the developed network as a feature extractor for constructing a normal model with limited data through experimental comparisons using vibration data collected from sensors. Specifically, we utilized MobileNet as a discriminative feature extractor to identify 37 wind turbines. By introducing metric learning based on t-SNE into its training process, we achieved a notable 50 % improvement in the AUC. Remarkably, this significant improvement was accomplished using only a small amount of data, approximately 13 minutes, obtained from the monitored turbine.
更多查看译文
关键词
Feature Representation,Discriminative Features,Wind Turbine,Anomaly Detection,Metric Learning,Anomaly Detection Systems,Normal Distribution,Environmental Changes,Normal Conditions,Training Data,Small Amount Of Data,Limited Training Data,Vibration Data,Impact Of Environmental Changes,Compact Distribution,Data Normalization,Latent Variables,Feature Space,Conditional Probability,Gaussian Mixture Model,Input Space,Variational Autoencoder,Vibration Signals,Feature Extraction Process,Extract Discriminative Features,Anomaly Score,Abnormal Data,Perplexity
AI 理解论文
溯源树
样例
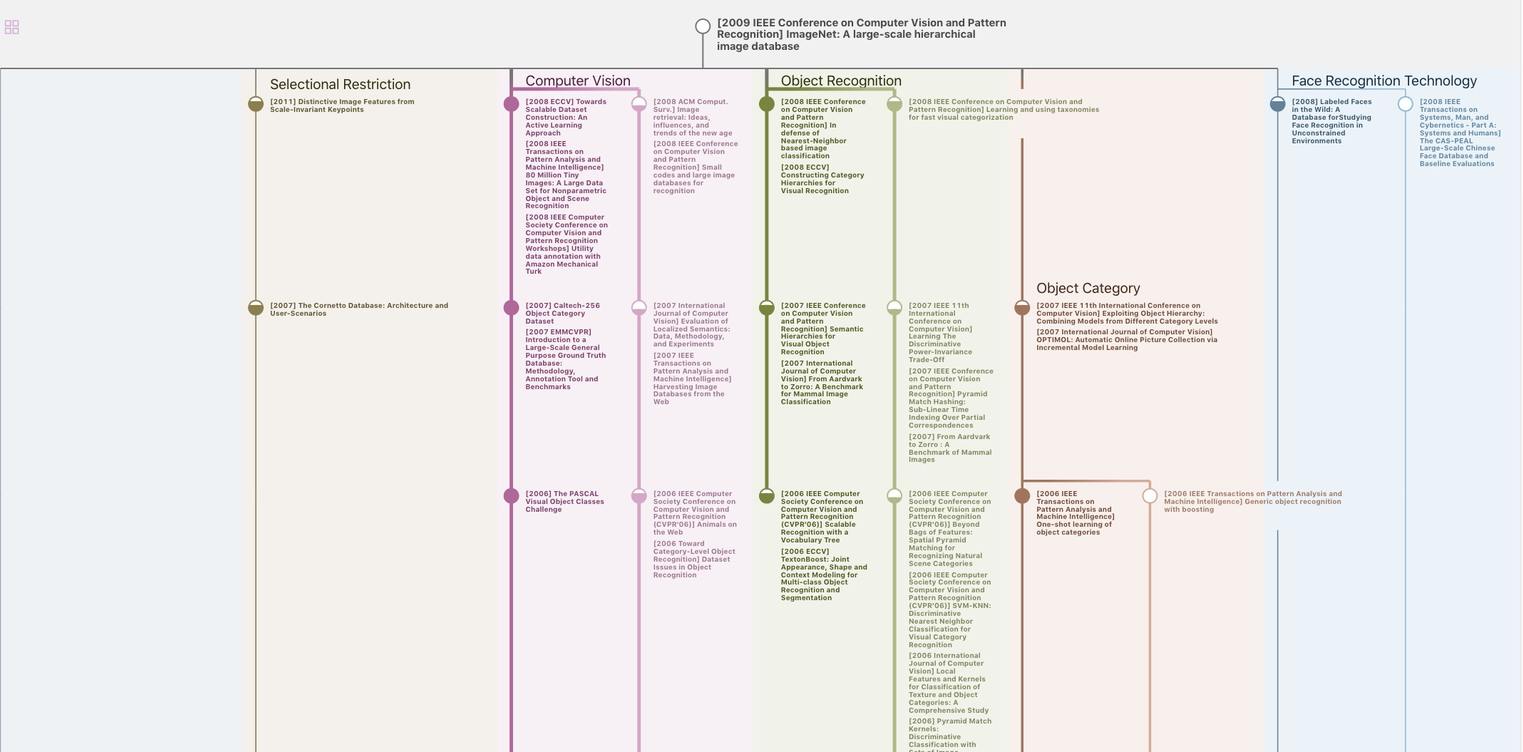
生成溯源树,研究论文发展脉络
Chat Paper
正在生成论文摘要