Enhancing Anomaly Detection Performance in Manufacturing Industry through Improved Alignment Algorithms.
International Conference on Electronics, Information and Communications(2024)
Abstract
In the realm of industrial manufacturing, particularly within the domain of anomaly detection tasks, one can discern a notable and pragmatic approach. These tasks exhibit exceptional prowess in surmounting challenges, including the burden of high labeling costs and the presence of substantial data imbalances. They furnish an efficacious means for identifying irregularities and defects in the production process without necessitating an abundance of annotated data. As such, they constitute a fiscally responsible and efficient solution for ensuring product quality and operational reliability. Notably, PaDiM stands as a widely embraced method, characterized by a pivotal advantage: it can be expeditiously deployed through the utilization of pretrained models derived from ImageNet, obviating the requirement for supplementary training on individual datasets. Nonetheless, it is imperative to underscore that the proper alignment of objects within images serves as a prerequisite for attaining heightened performance with this methodology. In pursuit of the refinement of alignment and the subsequent achievement of superior results in anomaly detection, we present a redefined alignment neural network architecture. We have implemented this architecture for the purpose of training and evaluation on a genuine industrial manufacturing dataset.
MoreTranslated text
Key words
industrial environment,anomaly detection,vision inspection,alignment
AI Read Science
Must-Reading Tree
Example
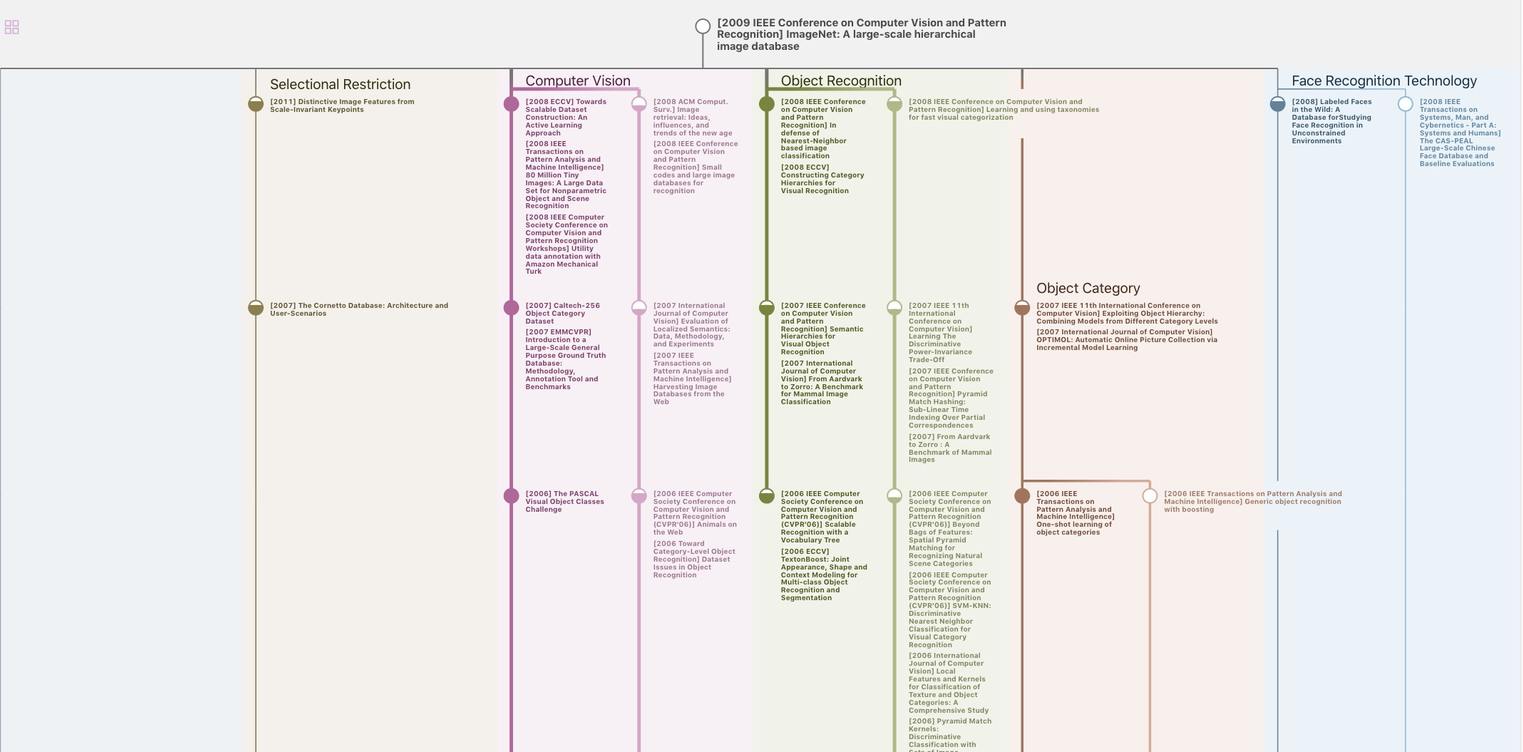
Generate MRT to find the research sequence of this paper
Chat Paper
Summary is being generated by the instructions you defined