Deep Convolutional Neural Networks for Predominant Instrument Recognition in Polyphonic Music Using Discrete Wavelet Transform
Circuits, Systems, and Signal Processing(2024)
摘要
In this article, a new multi-input deep convolutional neural networks (deep-CNNs) model architecture is addressed for the recognition of predominant instruments in polyphonic music using discrete wavelet transform (DWT). The proposed deep-CNNs model employs a fusion of Mel-spectrogram and Mel-frequency cepstral coefficient (MFCC) features as its first input and a concatenation of statistical features extracted from decomposed signals obtained through DWT as its second input. Particle swarm optimization (PSO), a feature selection algorithm, is employed to minimize the feature dimensionality by excluding the irrelevant features. The proposed model is experimentally tested on the IRMAS dataset using fixed-length single-labeled train data for model training and variable-length multi-labeled test data for model evaluation. The proposed model is evaluated using several DWT feature dimensions, and a feature dimension of 250 yields the best outcomes. The model performance is assessed by averaging the precision, recall, and F1 measures on a micro- and macro-level. For a set of optimal model hyperparameter values, our proposed model can reach micro and macro F1 measures of 0.695 and 0.631, which are 12.28 https://doi.org/10.1109/taslp.2016.2632307 ) CNN model, respectively.
更多查看译文
关键词
Predominant instrument recognition,Deep convolutional neural networks,Mel-spectrogram,MFCC,DWT,PSO
AI 理解论文
溯源树
样例
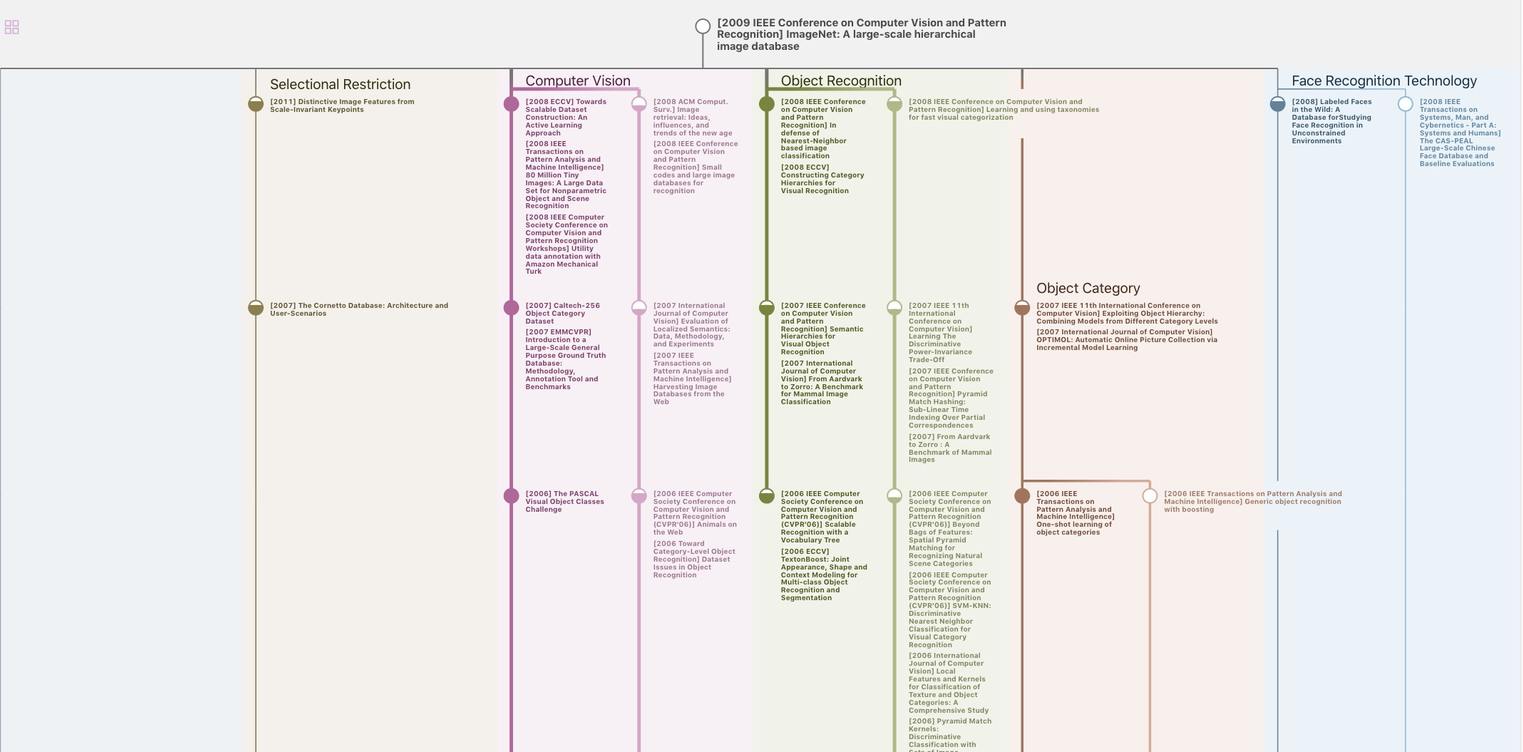
生成溯源树,研究论文发展脉络
Chat Paper
正在生成论文摘要