Event detection algorithm based on label semantic encoding
Discover Applied Sciences(2024)
摘要
One major challenge in event detection tasks is the lack of a large amount of annotated data. In a low-sample learning environment, effectively utilizing label semantic information can mitigate the impact of limited samples on model training. Therefore, this chapter proposes the SALM-Net (Semantic Attention Labeling Matching Network) model. Firstly, a Label Semantic Encoding (LSE) module is designed to obtain semantic encodings for labels. Next, a contrastive learning fine-tuning module is introduced to fine-tune the label semantic encodings produced by the LSE module. Finally, an attention module is used to match text encodings with label semantic encodings of events and arguments, thus obtaining event detection results. Experiments are conducted on the publicly available ACE2004 dataset, and the algorithm’s effectiveness is validated through an analysis of experimental results, comparing them with state-of-the-art algorithms.
更多查看译文
关键词
Event detection,Label semantic encoding,Natural language processing,Attention mechanism
AI 理解论文
溯源树
样例
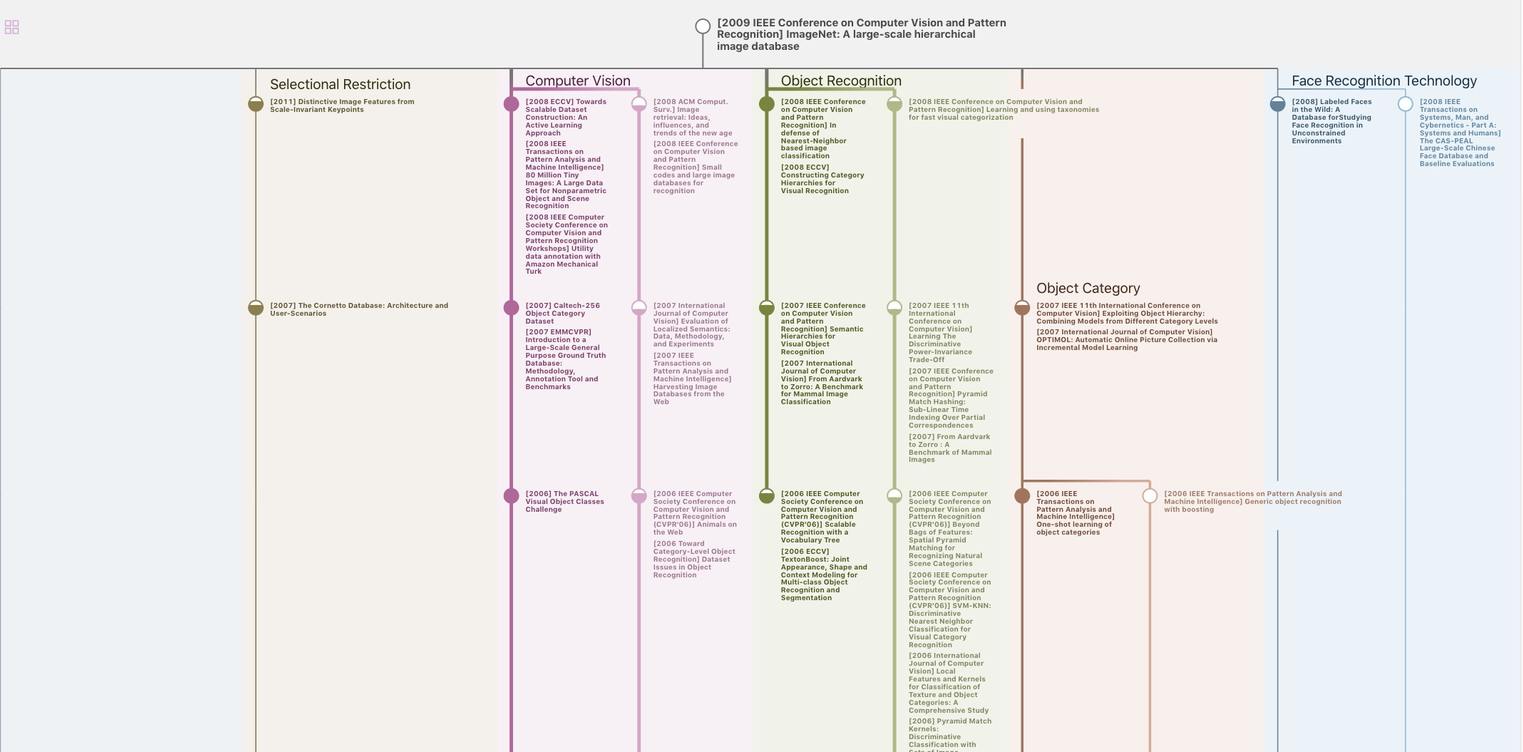
生成溯源树,研究论文发展脉络
Chat Paper
正在生成论文摘要