Fixed Inter-Neuron Covariability Induces Adversarial Robustness
ICASSP 2024 - 2024 IEEE International Conference on Acoustics, Speech and Signal Processing (ICASSP)(2024)
摘要
Vulnerability to adversarial perturbations is a major flaw of Deep Neural Networks (DNNs) that brings their reliability in real-world scenarios into question. However, biological perception, which DNNs emulate, is highly robust to such perturbations. This suggests that DNNs diverge from biological perception and develop vulnerability to adversarial perturbations. One point of divergence is that the activity of biological neurons is correlated and the structure of this correlation tends to be static across stimuli and over time, even if it hampers performance and learning. Conversely, we observe that small perturbations of the inputs can significantly change the inter-neuron correlations. We hypothesize that constraining the DNN’s neurons to respect a fixed inter-neuron correlation structure would improve its robustness. To test this hypothesis, we develop a Self-Consistent Activation (SCA) layer, which consists of neurons whose activations are optimized to conform to a fixed, but learned, correlation pattern. We train models with our SCA layer on image and audio recognition tasks and evaluate their accuracy under AutoAttack, an ensemble of white and black box adversarial attacks. Models with an SCA layer achieved up to 6% (abs) higher accuracy than traditional MLPs, without being trained on adversarially perturbed data. The code is available at https://github.com/ahmedshah1494/SCA-Layer.
更多查看译文
关键词
Adversarial Robustness,Deep Learning,Biologically inspired
AI 理解论文
溯源树
样例
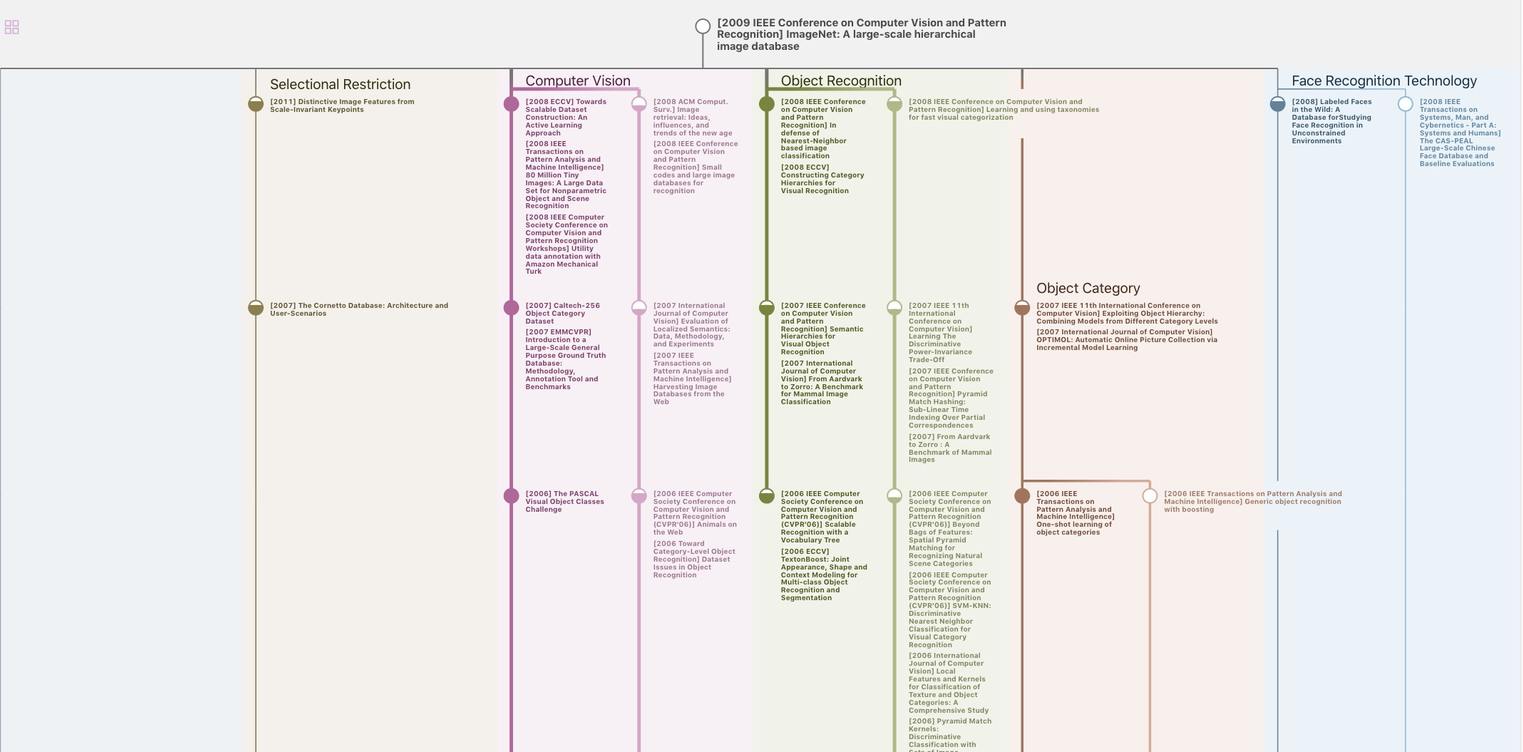
生成溯源树,研究论文发展脉络
Chat Paper
正在生成论文摘要