Outlier-Robust Feature Selection with ℓ2,1 -Norm Minimization and Group Row-Sparsity Induced Constraints
ICASSP 2024 - 2024 IEEE International Conference on Acoustics, Speech and Signal Processing (ICASSP)(2024)
摘要
In the realm of high-dimensional data analysis, the existence of outliers presents a substantial hurdle to the efficacy of feature selection methods that rely on the assumption of Gaussian distribution. To tackle this issue, we propose an outlier-robust feature selection method, ORFS, which combines robust ℓ
2,1
-norm minimization with group row-sparsity induced constrains to achieve both robustness and discriminative prediction capabilities. Moreover, the group row-sparsity constraints subspace learning based on ℓ
2,0
-norm can directly select features without parameter tuning. Finally, we introduce an iterative optimization strategy to solve NP-hard problem, and extensive experiments demonstrate the efficacy of ORFS in effectively eliminating the impact of outliers and significantly improving classification performance.
更多查看译文
关键词
Feature selection,outlier-robust,ℓ2,1-norm minimization,ℓ2,0-norm
AI 理解论文
溯源树
样例
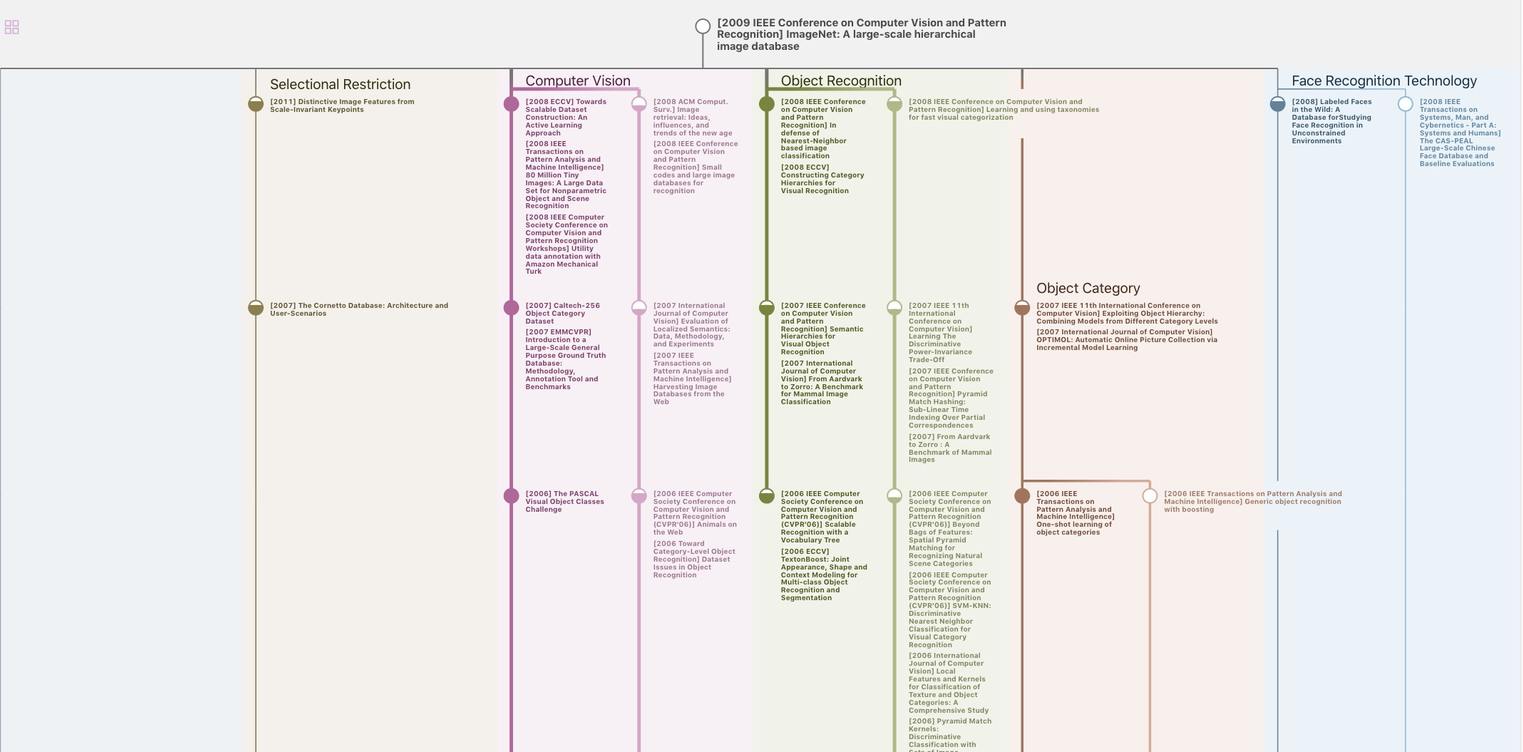
生成溯源树,研究论文发展脉络
Chat Paper
正在生成论文摘要