Towards an Interpretable AI Framework for Advanced Classification of Unmanned Aerial Vehicles (UAVs)
2024 IEEE 21ST CONSUMER COMMUNICATIONS & NETWORKING CONFERENCE, CCNC(2024)
摘要
With UAVs on the rise, accurate detection and identification are crucial. Traditional unmanned aerial vehicle (UAV) identification systems involve opaque decision-making, restricting their usability. This research introduces an RF-based Deep Learning (DL) framework for drone recognition and identification. We use cutting-edge eXplainable Artificial Intelligence (XAI) tools, SHapley Additive Explanations (SHAP), and Local Interpretable Model-agnostic Explanations(LIME). Our deep learning model uses these methods for accurate, transparent, and interpretable airspace security. With 84.59% accuracy, our deep-learning algorithms detect drone signals from RF noise. Most crucially, SHAP and LIME improve UAV detection. Detailed explanations show the model's identification decision-making process. This transparency and interpretability set our system apart. The accurate, transparent, and user-trustworthy model improves airspace security.
更多查看译文
关键词
Drone Detection,RF Signals,Deep Learning,SHAP,LIME,Explainable AI,Airspace Security
AI 理解论文
溯源树
样例
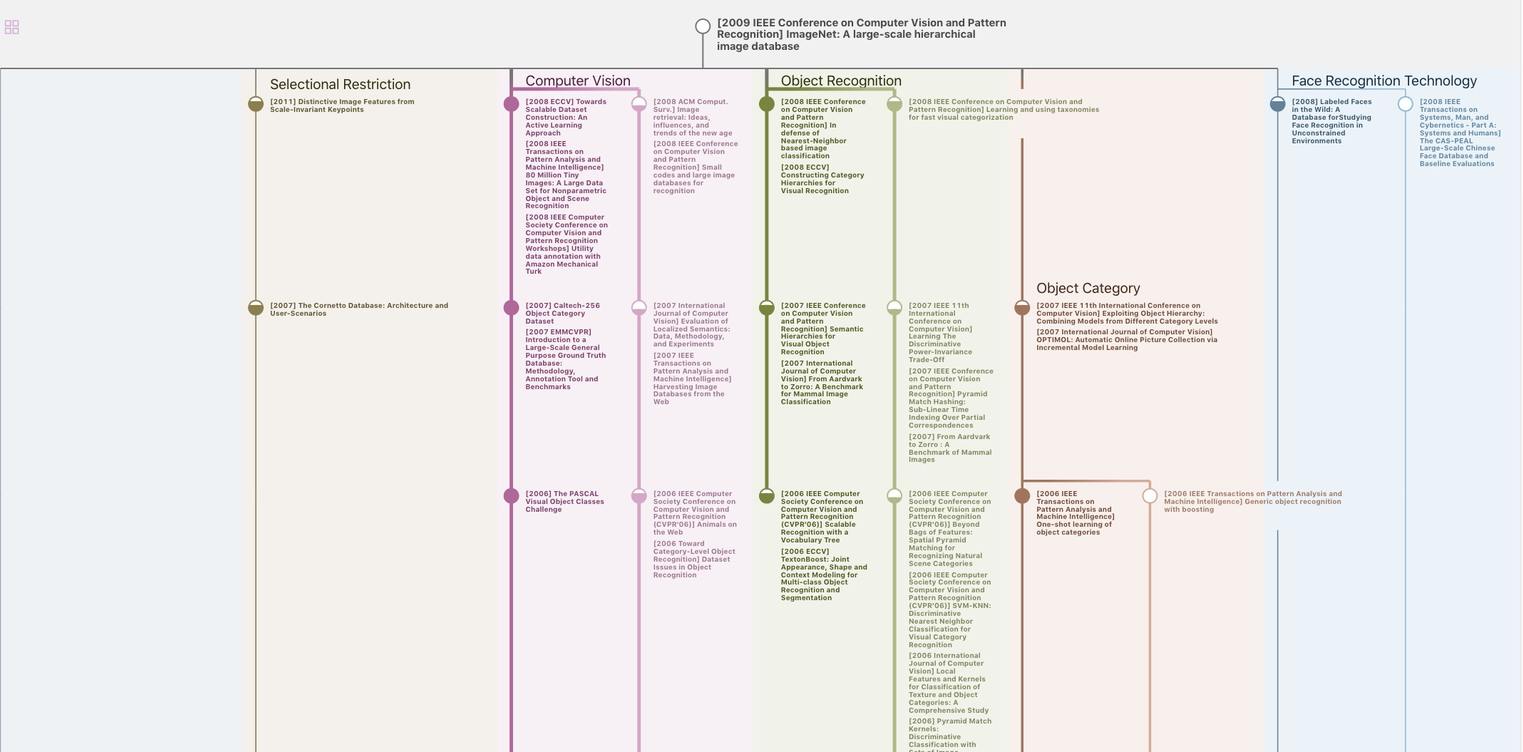
生成溯源树,研究论文发展脉络
Chat Paper
正在生成论文摘要