Link prediction using deep autoencoder-like non-negative matrix factorization with L21-norm
Applied Intelligence(2024)
摘要
Link prediction aims to predict missing links or eliminate spurious links and anticipate new links by analyzing observed network topological structure information. Non-negative matrix factorization(NMF) is widely used in solving the issue of link prediction due to its good interpretability and scalability. However, most existing NMF-based approaches involve shallow decoder models, which are incapable of capturing complex hierarchical information hidden in networks, and seldom consider random noise. To address these issues, a novel deep autoencoder-like nonnegative matrix factorization method with L_2,1 norm for link prediction models(DANMFL) is proposed. Unlike conventional NMF-based approaches, our model contains a decoder component and an encoder component, which capture complex hierarchical information effectively, leading to more accurate prediction results. In addition, we use the L_2,1 norm to remove random noise in each layer and the convergence of our model is strictly proven. We conduct extensive experiments on twelve real-world networks and the experimental results show that DANMFL is superior to existing state-of-the-art baseline approaches in terms of prediction accuracy. Codes are available at https://github.com/litongf/DANMFL .
更多查看译文
关键词
Link prediction,NMF,Deep autoencoder-like NMF,norm
AI 理解论文
溯源树
样例
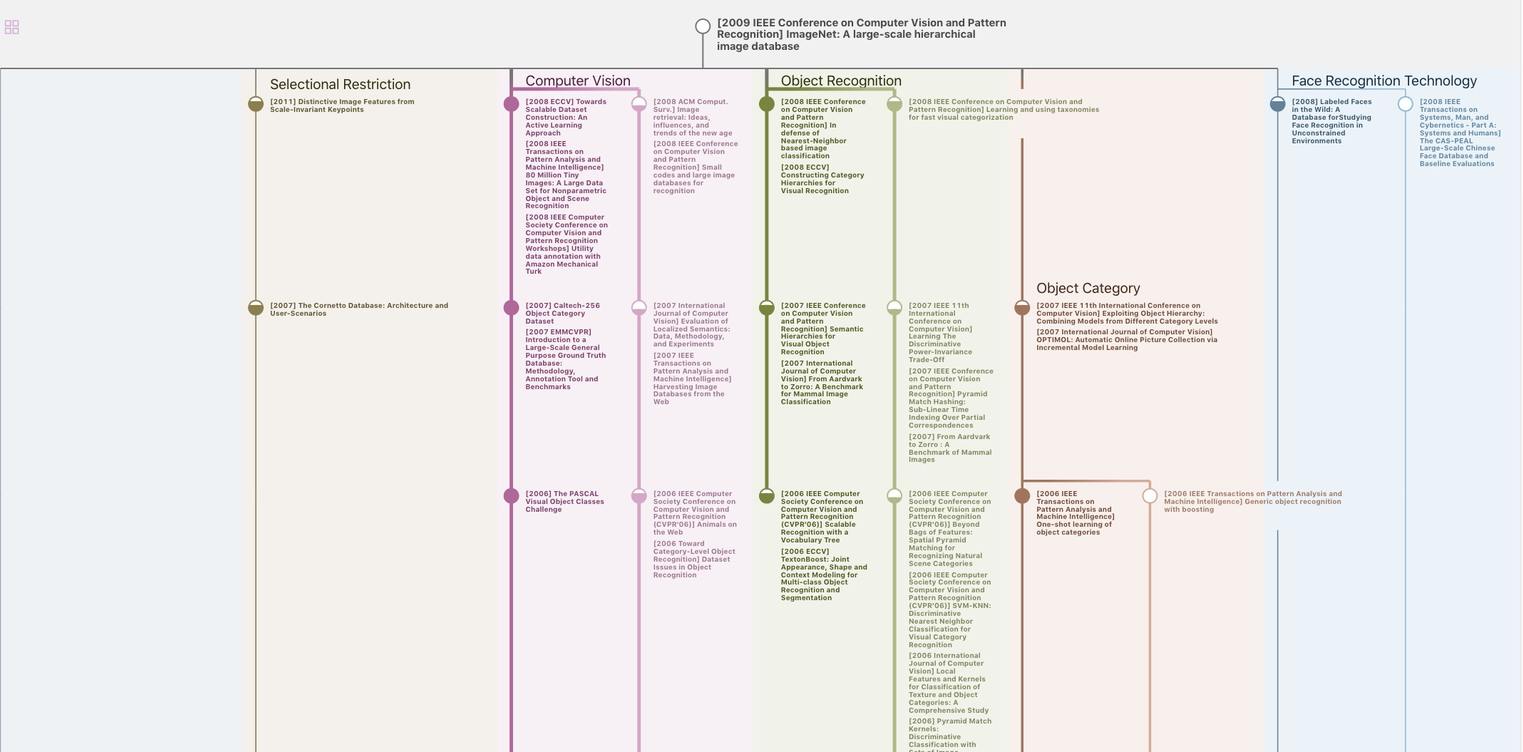
生成溯源树,研究论文发展脉络
Chat Paper
正在生成论文摘要