FedSafe-No KDC Needed: Decentralized Federated Learning With Enhanced Security and Efficiency
2024 IEEE 21ST CONSUMER COMMUNICATIONS & NETWORKING CONFERENCE, CCNC(2024)
摘要
Cloud-based federated learning (FL) services have received increasing attention due to their ability to enable collaborative global model training without the need to collect local data from participants. To generate a global model, local models are trained on participants' local data and only model parameters are sent to an aggregator server. Nonetheless, revealing model parameters can still reveal training data via launching attacks, e.g., inference and membership. Hence, to protect model parameters, a secure global model aggregation scheme is needed to protect these parameters from unauthorized access. Existing solutions to this issue, which are based on homomorphic encryption and secure multi-party computation, tend to have large overheads and slow down training times. Functional encryption (FE) has been proposed as a solution for resolving privacy-preservation issues in FL, but current solutions suffer from high overhead and lack of security such as leaking master private key. To address these issues, this paper proposes a privacy-protecting, efficient, and decentralized FL framework, called FedSafe, based on FE without the need for a trusted key distribution center (KDC). The proposed scheme allows the participants to communicate with an aggregator to construct a global model without disclosing or learning their local models' parameters or the training data, thereby safeguarding their privacy. Through rigorous testing with real-world data, it is demonstrated that FedSafe outperforms the state-of-the-art privacy-protecting FL schemes in terms of security, scalability, and communication and computation overhead. Unlike existing approaches, this is accomplished without depending on any trusted KDC.
更多查看译文
关键词
Federated learning,privacy preservation,functional encryption,decentralization
AI 理解论文
溯源树
样例
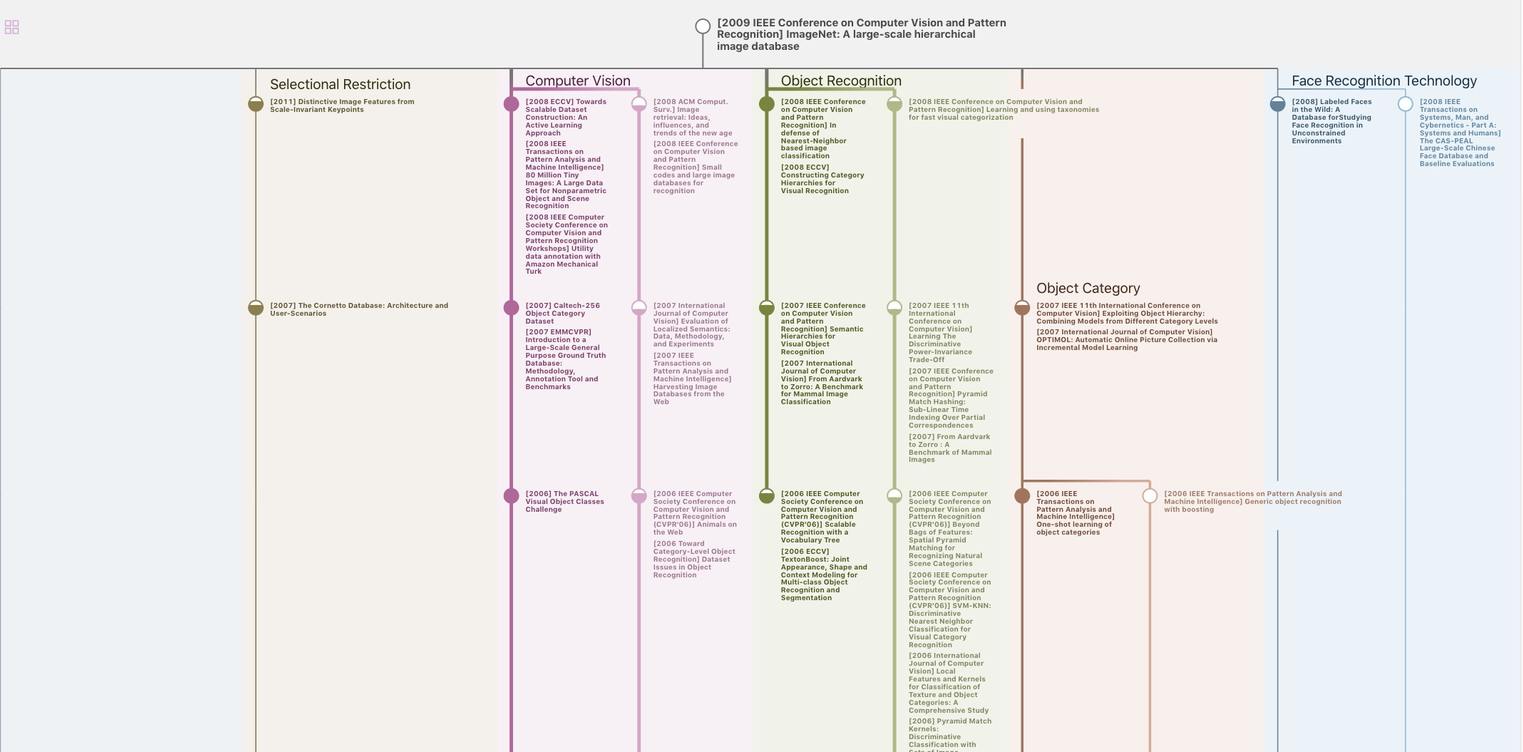
生成溯源树,研究论文发展脉络
Chat Paper
正在生成论文摘要