Rapid classification of epidemiologically relevant age categories of the malaria vector, Anopheles funestus
Parasites & Vectors(2024)
摘要
Accurately determining the age and survival probabilities of adult mosquitoes is crucial for understanding parasite transmission, evaluating the effectiveness of control interventions and assessing disease risk in communities. This study was aimed at demonstrating the rapid identification of epidemiologically relevant age categories of Anopheles funestus, a major Afro-tropical malaria vector, through the innovative combination of infrared spectroscopy and machine learning, instead of the cumbersome practice of dissecting mosquito ovaries to estimate age based on parity status. Anopheles funestus larvae were collected in rural south-eastern Tanzania and reared in an insectary. Emerging adult females were sorted by age (1–16 days old) and preserved using silica gel. Polymerase chain reaction (PCR) confirmation was conducted using DNA extracted from mosquito legs to verify the presence of An. funestus and to eliminate undesired mosquitoes. Mid-infrared spectra were obtained by scanning the heads and thoraces of the mosquitoes using an attenuated total reflection–Fourier transform infrared (ATR–FT-IR) spectrometer. The spectra (N = 2084) were divided into two epidemiologically relevant age groups: 1–9 days (young, non-infectious) and 10–16 days (old, potentially infectious). The dimensionality of the spectra was reduced using principal component analysis, and then a set of machine learning and multi-layer perceptron (MLP) models were trained using the spectra to predict the mosquito age categories. The best-performing model, XGBoost, achieved overall accuracy of 87
更多查看译文
关键词
Malaria,Anopheles funestus,Deep learning,Machine learning,Ifakara Health Institute,Mid-infrared spectroscopy
AI 理解论文
溯源树
样例
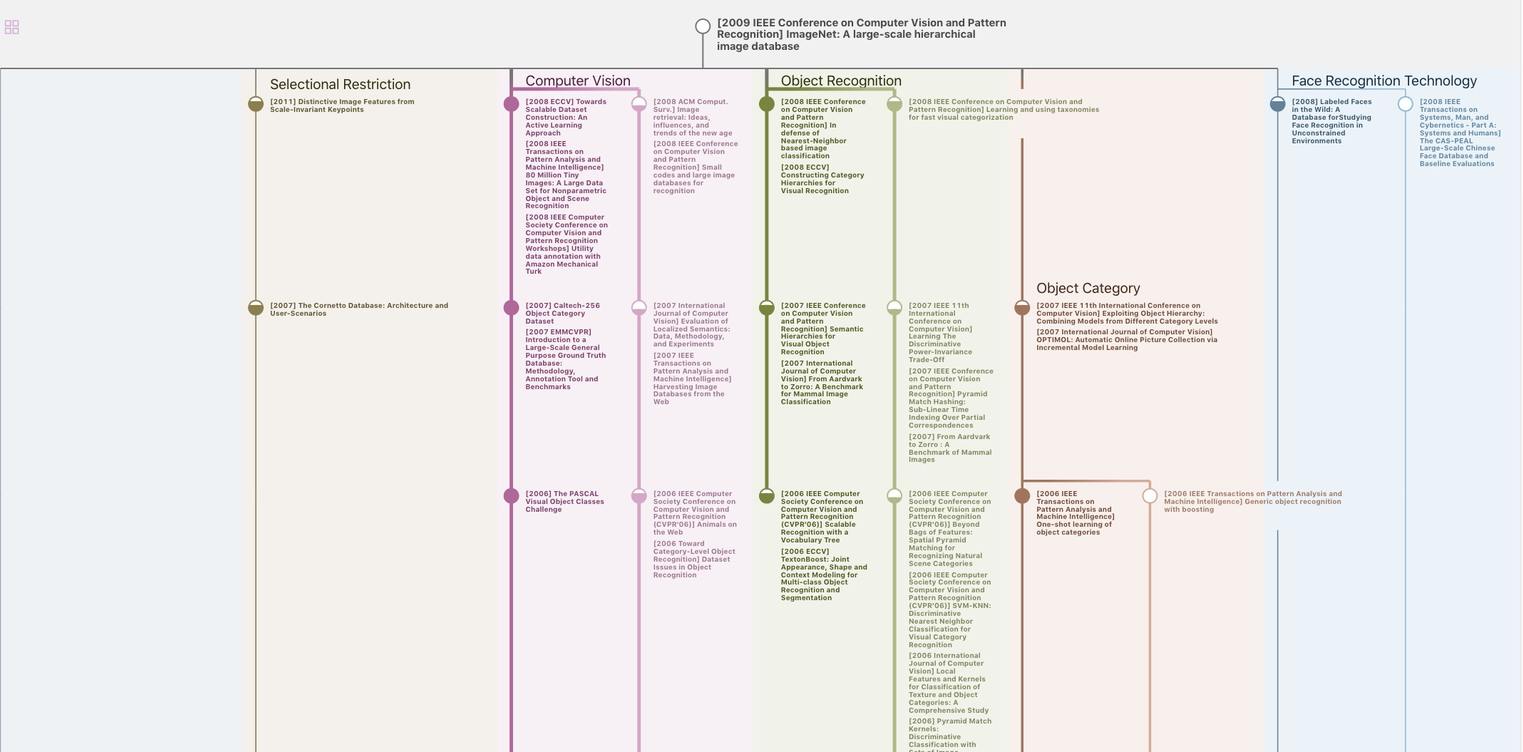
生成溯源树,研究论文发展脉络
Chat Paper
正在生成论文摘要