Towards Generic Deepfake Detection with Dynamic Curriculum
ICASSP 2024 - 2024 IEEE International Conference on Acoustics, Speech and Signal Processing (ICASSP)(2024)
摘要
Most previous deepfake detection methods bent their efforts to discriminate artifacts by end-to-end training. However, the learned networks often fail to mine the generic face forgery information efficiently due to ignoring data diversity. In this work, we propose to introduce sample hardness into the training of deepfake detectors via a curriculum learning paradigm. Specifically, we present a novel simple yet effective strategy, named Dynamic Facial Forensic Curriculum (DFFC), which makes the model gradually focus on hard samples during the training. To this end, we propose Dynamic Forensic Hardness (DFH) which integrates the facial quality score and instantaneous instance loss to dynamically measure sample hardness during training. Besides, we present a pacing function to construct data subsets from easy to hard throughout the training process based on DFH. Comprehensive experiments show that DFFC can improve both within- and cross-dataset performance of various kinds of end-to-end deepfake detectors in a plug-and-play manner. It indicates that DFFC can help deepfake detectors learn generic forgery discriminative features more efficiently by exploiting the information from hard samples.
更多查看译文
关键词
Deepfake detection,Curriculum learning,Face image quality
AI 理解论文
溯源树
样例
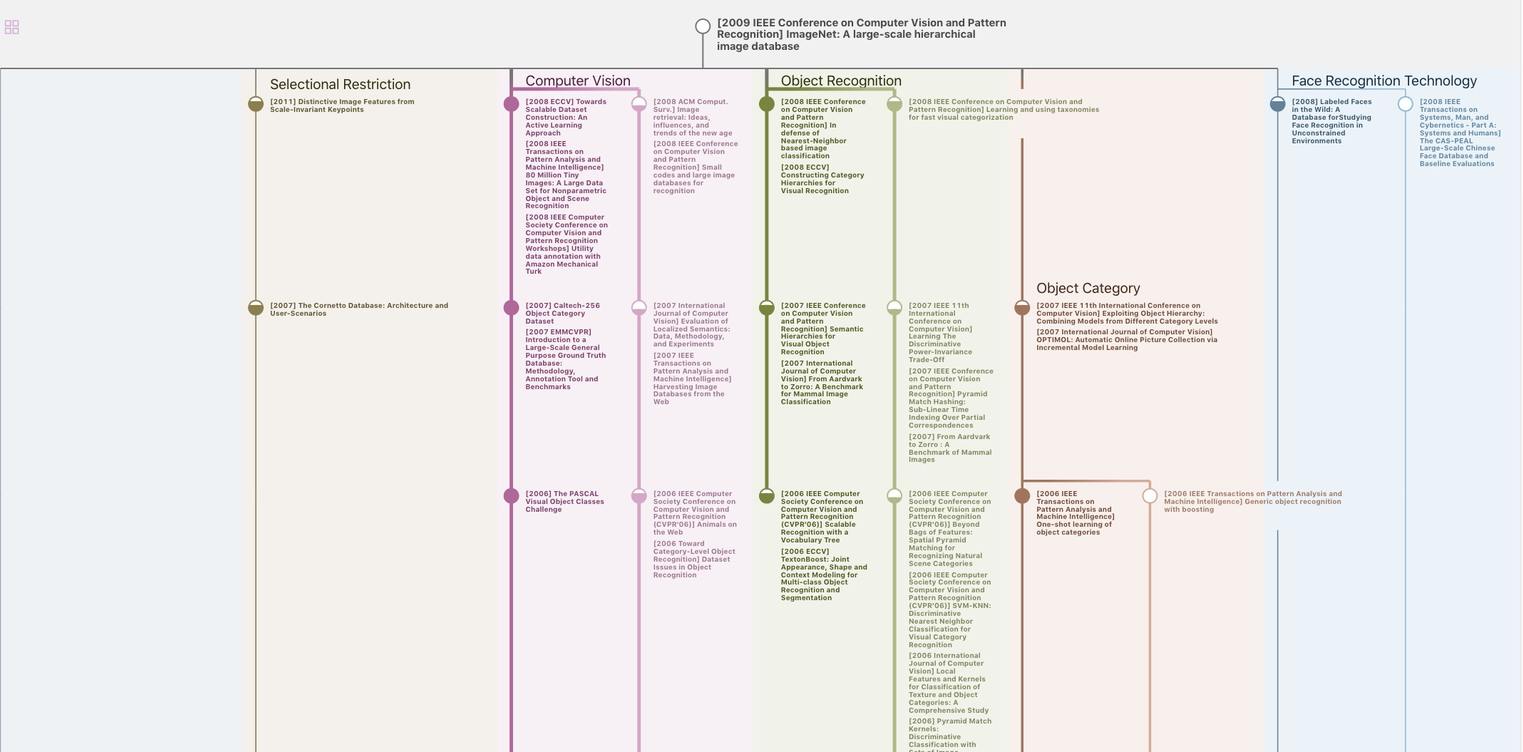
生成溯源树,研究论文发展脉络
Chat Paper
正在生成论文摘要