GR0: Self-Supervised Global Representation Learning for Zero-Shot Voice Conversion
ICASSP 2024 - 2024 IEEE International Conference on Acoustics, Speech and Signal Processing (ICASSP)(2024)
摘要
Research in generative self-supervised learning (SSL) has largely focused on local embeddings for tokenized sequences. We introduce a generative SSL framework that learns a global representation that is disentangled from local embeddings. We apply this technique to jointly learn a global speaker embedding and a zero-shot voice converter. The converter modifies recorded speech to sound as if it were spoken by a different person while preserving the content, using only a short reference clip unavailable to the model during training. Listening experiments conducted on an unseen dataset show that our models significantly outperform SOTA baselines in both quality and speaker similarity for various datasets and unseen languages.
更多查看译文
关键词
generative self-supervised global representation learning,cross-lingual zero-shot voice conversion
AI 理解论文
溯源树
样例
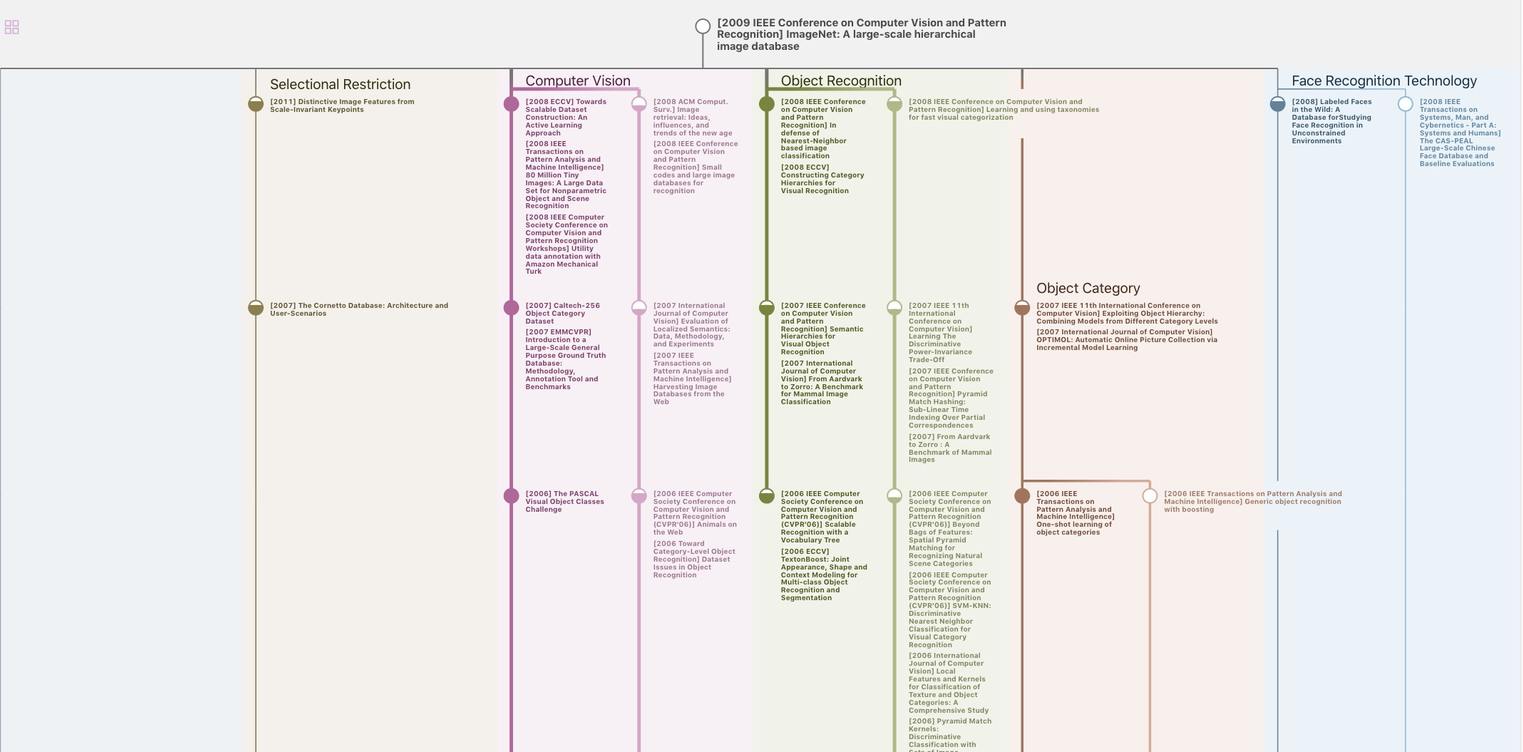
生成溯源树,研究论文发展脉络
Chat Paper
正在生成论文摘要