Probability-Aware Word-Confusion-Network-To-Text Alignment Approach for Intent Classification
ICASSP 2024 - 2024 IEEE International Conference on Acoustics, Speech and Signal Processing (ICASSP)(2024)
摘要
Spoken Language Understanding (SLU) technologies have greatly improved due to the effective pretraining of speech representations. A common requirement of industry-based solutions is the portability to deploy SLU models in voice-assistant devices. Thus, distilling knowledge from large text-based language models has become an attractive solution for achieving good performance and guaranteeing portability. In this paper, we introduce a novel architecture that uses a cross-modal attention mechanism to extract bin-level contextual embeddings from a word-confusion network (WNC) encoding such that these can be directly compared and aligned with traditional text-based contextual embeddings. This alignment is achieved using a recently proposed tokenwise constrastive loss function. We validate our architecture’s effectiveness by fine-tuning our WCN-based pretrained model to do intent classification (IC) on the well-known SLURP dataset. Obtained accuracy on the IC task (81%), depicts a 9.4% relative improvement compared to a recent/equivalent E2E method.
更多查看译文
关键词
Word-Confusion-Networks,Cross-modal Alignment,Knowledge Distillation,Intent Classification
AI 理解论文
溯源树
样例
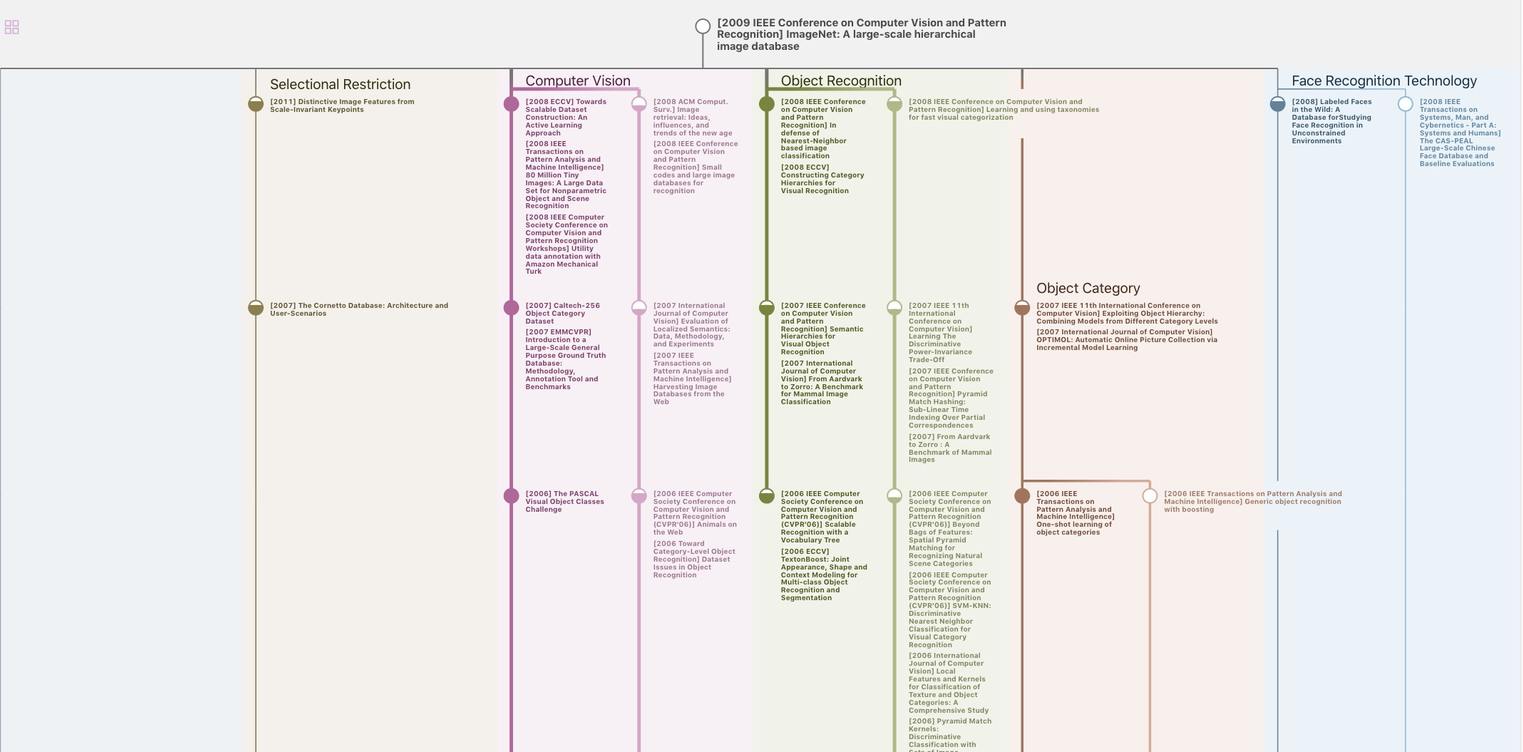
生成溯源树,研究论文发展脉络
Chat Paper
正在生成论文摘要