From Game Theory to Visual Recognition: Advancing DNN Robustness.
IEEE International Conference on Acoustics, Speech, and Signal Processing(2024)
摘要
In many real-world applications of Deep Neural Networks (DNNs) in visual recognition, data augmentation stands out as a premier tool for enhancing model robustness. Stemming from the understanding of the common mechanisms of data augmentation methods, we introduce the mask-based "data augmentation boost" (DaBoost) method, a strategic approach that exploits the control of game interaction strength. Our empirical results are telling: DaBoost not only consistently surpasses the state-of-the-art PixMix method but also achieves impressive robustness metrics, with a vanilla WideResNet registering a mere 6.5% mCE and a 2.3% RMS calibration error on CIFAR-10 data. An intriguing observation from our study is the Long-Rope Effect. We discerned that penalizing high-order interactions inadvertently leads to a boost in mid-order interactions, mirroring patterns inherent to human cognitive processes. This interplay hints at the potential avenues for optimizing DNNs’ performance further.
更多查看译文
关键词
Data augmentation,Game theory,Game interactions,Model robustness,Computer vision
AI 理解论文
溯源树
样例
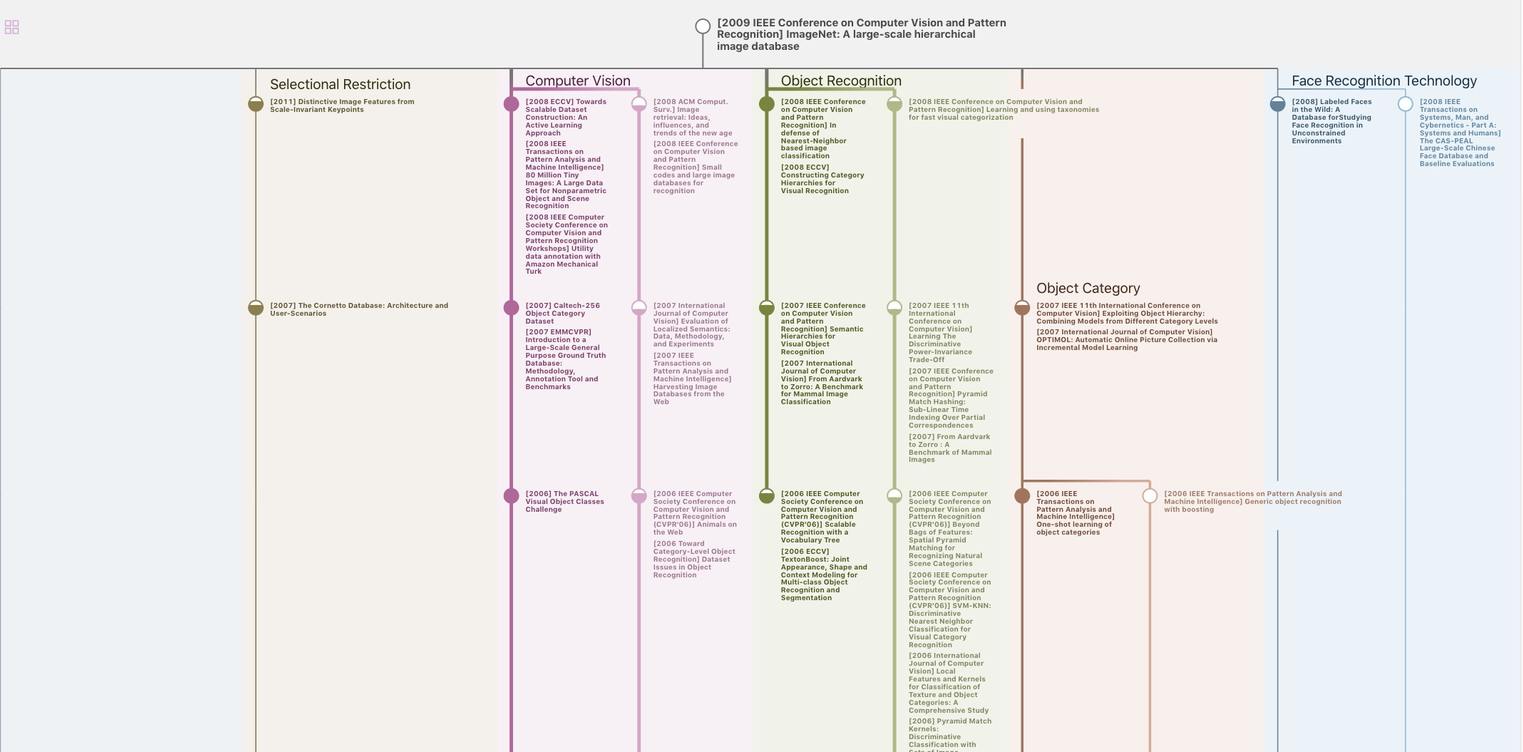
生成溯源树,研究论文发展脉络
Chat Paper
正在生成论文摘要