AI-Powered Network Intrusion Detection: A New Frontier in Cybersecurity.
Arab Conference on Information Technology(2023)
摘要
Intrusion Detection Systems (IDS) constitute a critical line of defense in contemporary cybersecurity efforts, designed to identify and counteract unauthorized access and malicious activities within computer networks. Leveraging the capabilities of Machine Learning (ML) algorithms, IDS endeavors to distinguish potentially harmful alterations and security breaches. This study delves into the pivotal question of algorithm selection for optimal performance. Machine Learning-based Intrusion Detection Systems (ML-IDS) are designed not only to enhance overall system security but also to strike a balance between minimizing false alarms and maximizing true alarm rates. To address this, we empirically evaluate five ML algorithms and present their performance in the context of network intrusion detection. Those algorithms are as follows: Random Forest achieves an impressive accuracy rate of 99.88%, Gradient Boosting demonstrates robust performance at 99.76%, AdaBoost exhibits an accuracy of 90.00%, Decision Tree boasts a noteworthy accuracy of 99.80%, and Extremely Randomized Trees demonstrate substantial proficiency with an accuracy of 99.86%. This empirical exploration enriches our comprehension of these algorithms and offers critical insights to enhance the security of computer systems.
更多查看译文
关键词
Network Intrusion Detection Systems,Machine Learning Algorithms,AI,Algorithm Performance,Cybersecurity Enhancement
AI 理解论文
溯源树
样例
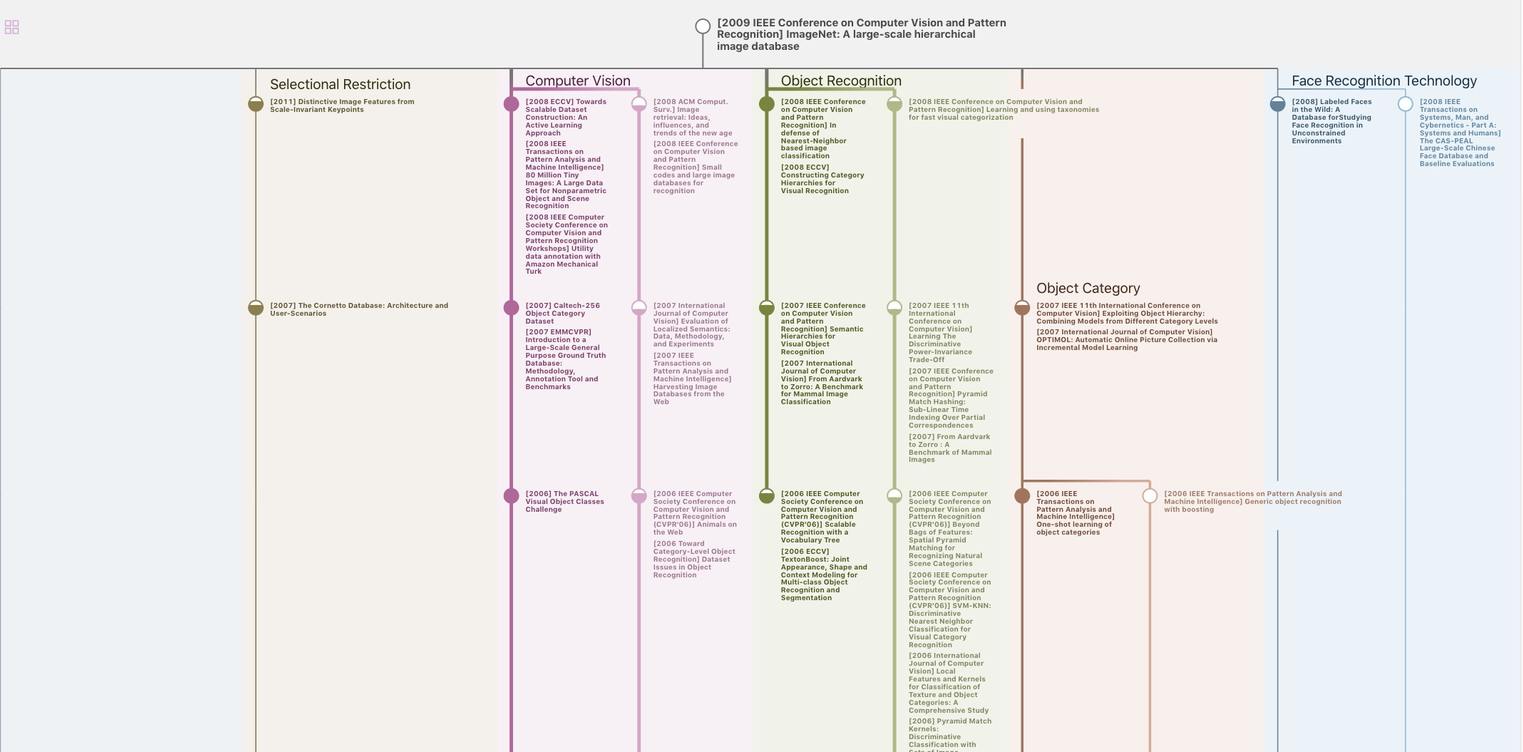
生成溯源树,研究论文发展脉络
Chat Paper
正在生成论文摘要