A Protein Language Model for Exploring Viral Fitness Landscapes
biorxiv(2024)
摘要
Successively emerging SARS-CoV-2 variants lead to repeated epidemic surges through escalated spreading potential (i.e., fitness). Modeling genotype–fitness relationship enables us to pinpoint the mutations boosting viral fitness and flag high-risk variants immediately after their detection. Here, we introduce CoVFit, a protein language model able to predict the fitness of variants based solely on their spike protein sequences. CoVFit was trained with genotype–fitness data derived from viral genome surveillance and functional mutation data related to immune evasion. When limited to only data available before the emergence of XBB, CoVFit successfully predicted the higher fitness of the XBB lineage. Fully-trained CoVFit identified 549 fitness elevation events throughout SARS-CoV-2 evolution until late 2023. Furthermore, a CoVFit-based simulation was able to predict the higher fitness of JN.1 subvariants before their detection. Our study provides both insight into the SARS-CoV-2 fitness landscape and a novel tool potentially transforming viral genome surveillance.
### Competing Interest Statement
Jumpei Ito has consulting fees and honoraria for lectures from Takeda Pharmaceutical Co. Ltd. Spyros Lytras has consulting fees from EcoHealth Alliance. Kei Sato has consulting fees from Moderna Japan Co., Ltd. and Takeda Pharmaceutical Co. Ltd. and honoraria for lectures from Gilead Sciences, Inc., Moderna Japan Co., Ltd., and Shionogi & Co., Ltd. The other authors declare no competing interests. Conflicts that the editors consider relevant to the content of the manuscript have been disclosed.
更多查看译文
AI 理解论文
溯源树
样例
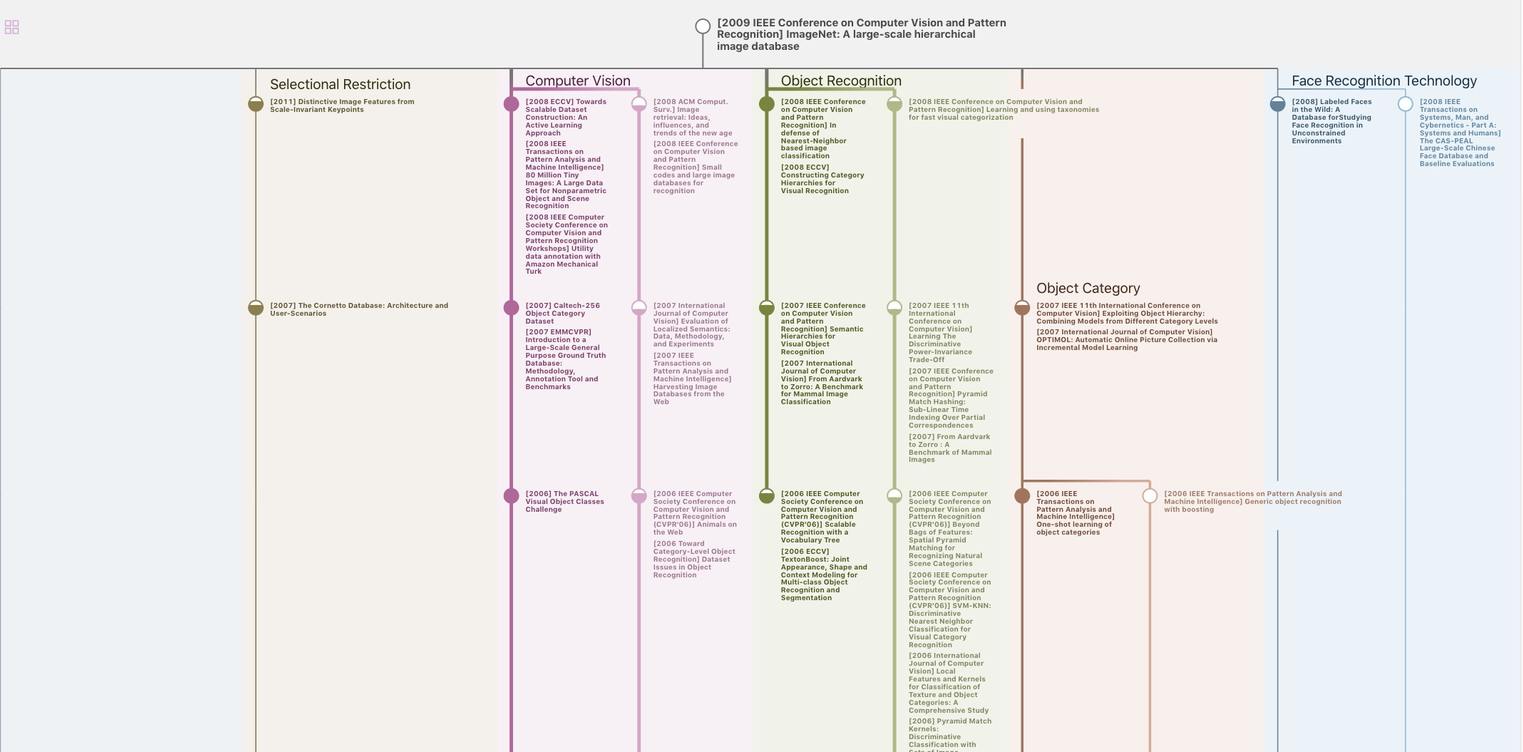
生成溯源树,研究论文发展脉络
Chat Paper
正在生成论文摘要