Label Rectified and Graph Adaptive Semi-Supervised Regression for Electrode Shifted Gesture Recognition
ICASSP 2024 - 2024 IEEE International Conference on Acoustics, Speech and Signal Processing (ICASSP)(2024)
摘要
Surface electromyography (sEMG) noninvasively records muscle activities. It provides valuable information about muscle contractions and enables real-time decoding into hand gestures. Recently many studies have successfully demonstrated this capability. However, the accuracy of gesture recognition decreases significantly due to electrode shifts. Without increasing the density of electrodes which may cause the curse of dimensionality and result in higher costs, we propose a label rectified and graph adaptive semi-supervised regression (LRGASR) model for electrode shifted gesture recognition. LRGASR on one hand learns an optimal graph to characterize the underlying semantic connectionship of both non-shifted and shifted sEMG samples and takes advantage of label rectification to reduce the feature-label inconsistency of shifted ones. Experimental results show that LRGASR achieved the average recognition accuracies 78.20% and 87.28% on the SeNic and ISRMyo sEMG data sets, which outperforms six existing models.
更多查看译文
关键词
sEMG,semi-supervised learning,hand gesture recognition,electrode shifts,noise label
AI 理解论文
溯源树
样例
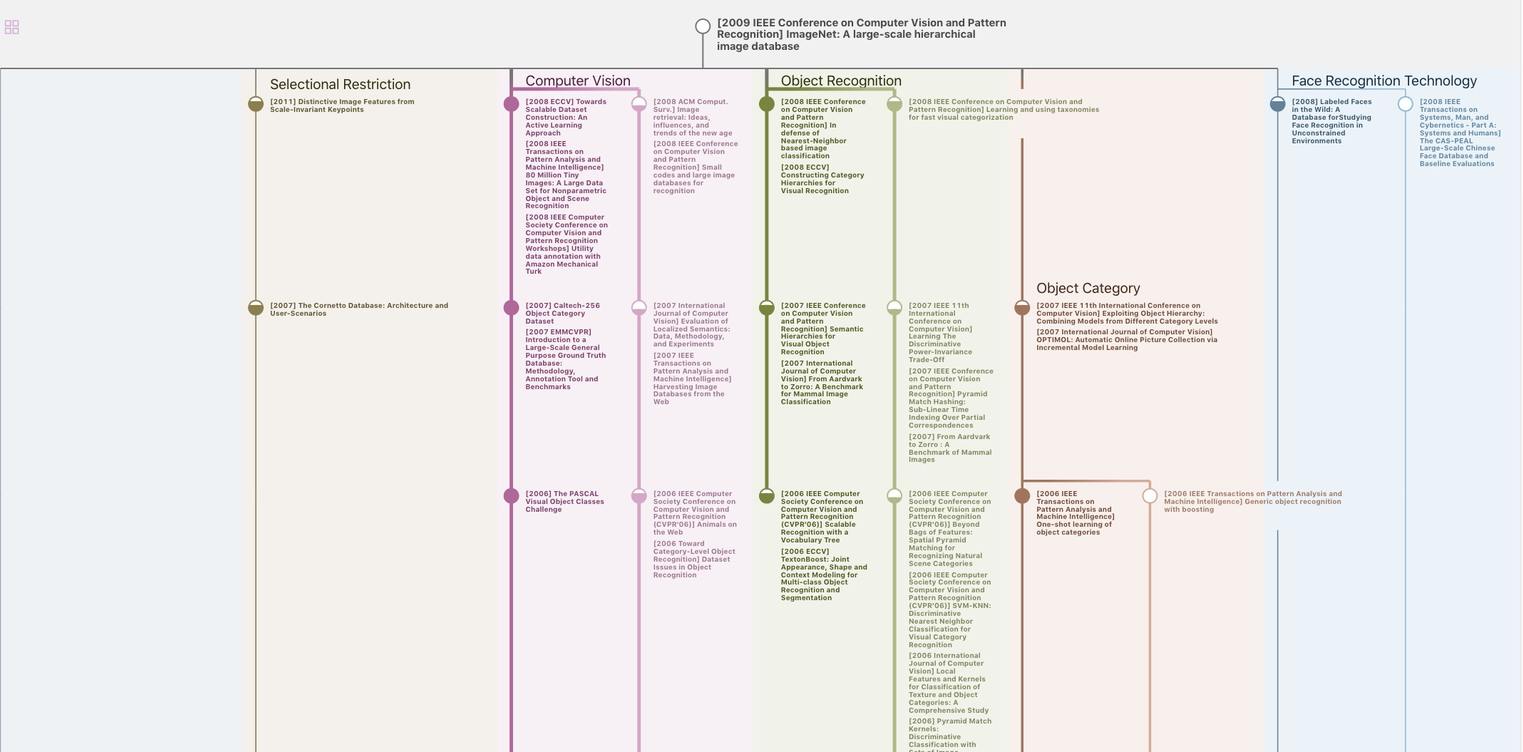
生成溯源树,研究论文发展脉络
Chat Paper
正在生成论文摘要