Enhancing Multi-Task Models For Recommendation with Tensor Trace Norm
ICASSP 2024 - 2024 IEEE International Conference on Acoustics, Speech and Signal Processing (ICASSP)(2024)
摘要
Noise is a pervasive issue in recommendation systems, which can stem from user behaviors that do not align with their intentions. As a result, noise reduction has become a prominent area of research in the field of recommendation systems. However, existing noise reduction techniques in recommendation tend to compromise the performance of certain task objectives. Moreover, they require modifying the structure of the model, which introduces inference latency and additional space cost. In this paper, we propose a straightforward yet powerful approach, Multi-layer Tensor trace Norm (MTN), to address noise-related challenges. Our method achieves this by promoting information sharing across different tasks using tensor trace norms. By leveraging norms, MTN effectively reduces noise without modifying the model’s structure or incurring substantial time and space complexities. Extensive experiments on public datasets and generated noisy datasets demonstrate the effectiveness of MTN on several of the most popular multi-task models.
更多查看译文
关键词
Multi-task learning,recommendation,noise reduction,low-rank
AI 理解论文
溯源树
样例
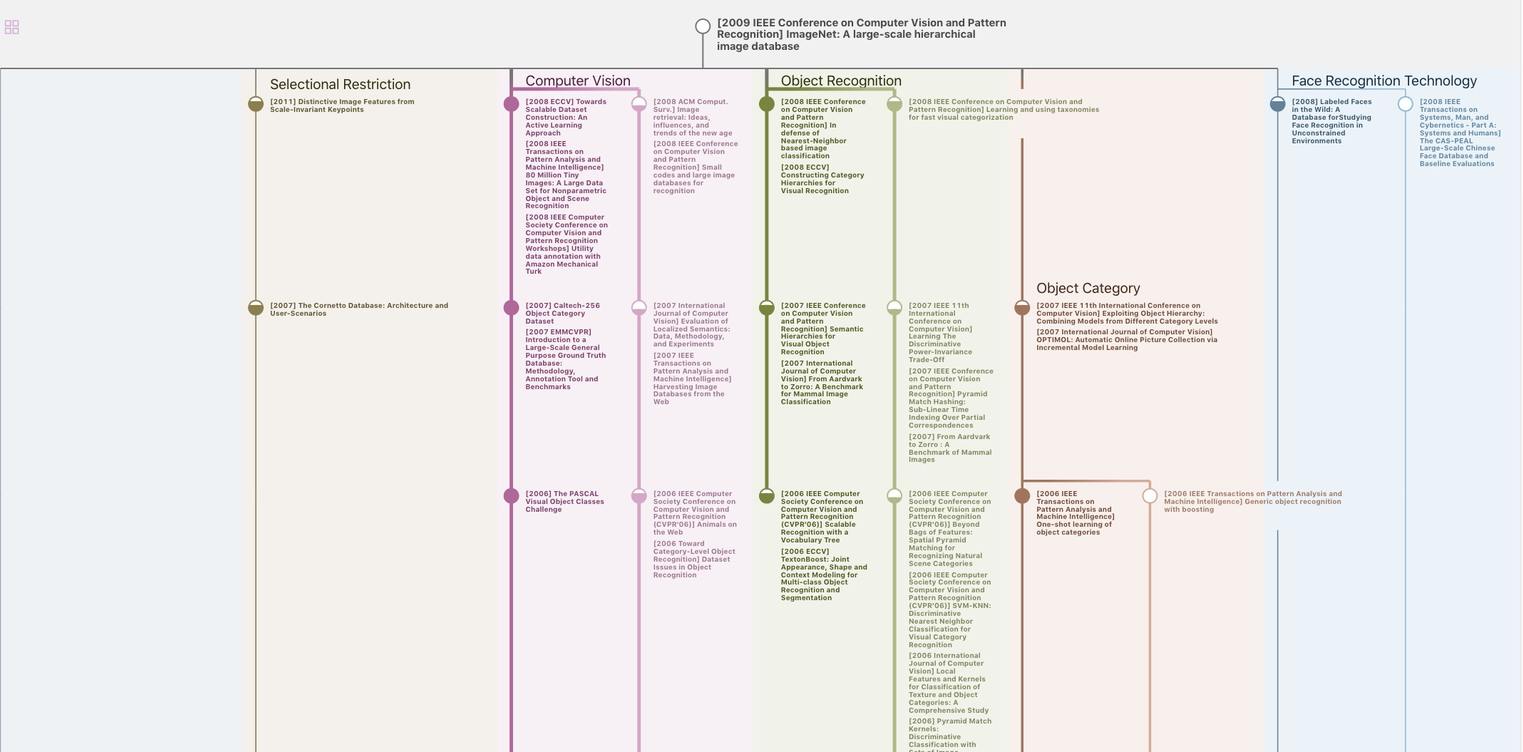
生成溯源树,研究论文发展脉络
Chat Paper
正在生成论文摘要