Surface-Constrained Progressive Feature Preserving Point Cloud Compression.
IEEE International Conference on Acoustics, Speech, and Signal Processing(2024)
摘要
Current point cloud compression methods based on deep learning cannot guarantee that the reconstructed points are constrained to the surface, resulting in low reconstruction quality at low bitrates. Hence, this paper proposes an efficient deep learning-based point cloud geometry compression algorithm. Specifically, by introducing a two-dimensional plane at the decoder, the reconstructed local patch is constrained within a manifold, preserving sufficient surface features. This strategy ensures the decoder can reconstruct high-quality point clouds even at low bitrates. Moreover, we use the anchor features obtained by the neural network to compress the local features at the encoder. The experimental results show that, under the condition of the same restoration quality, the proposed method improves the point-to-plane PSNR by more than 2dB compared to the state-of-the-art methods. The code is available at https://github.com/zbaoye/SurfPCC.
更多查看译文
AI 理解论文
溯源树
样例
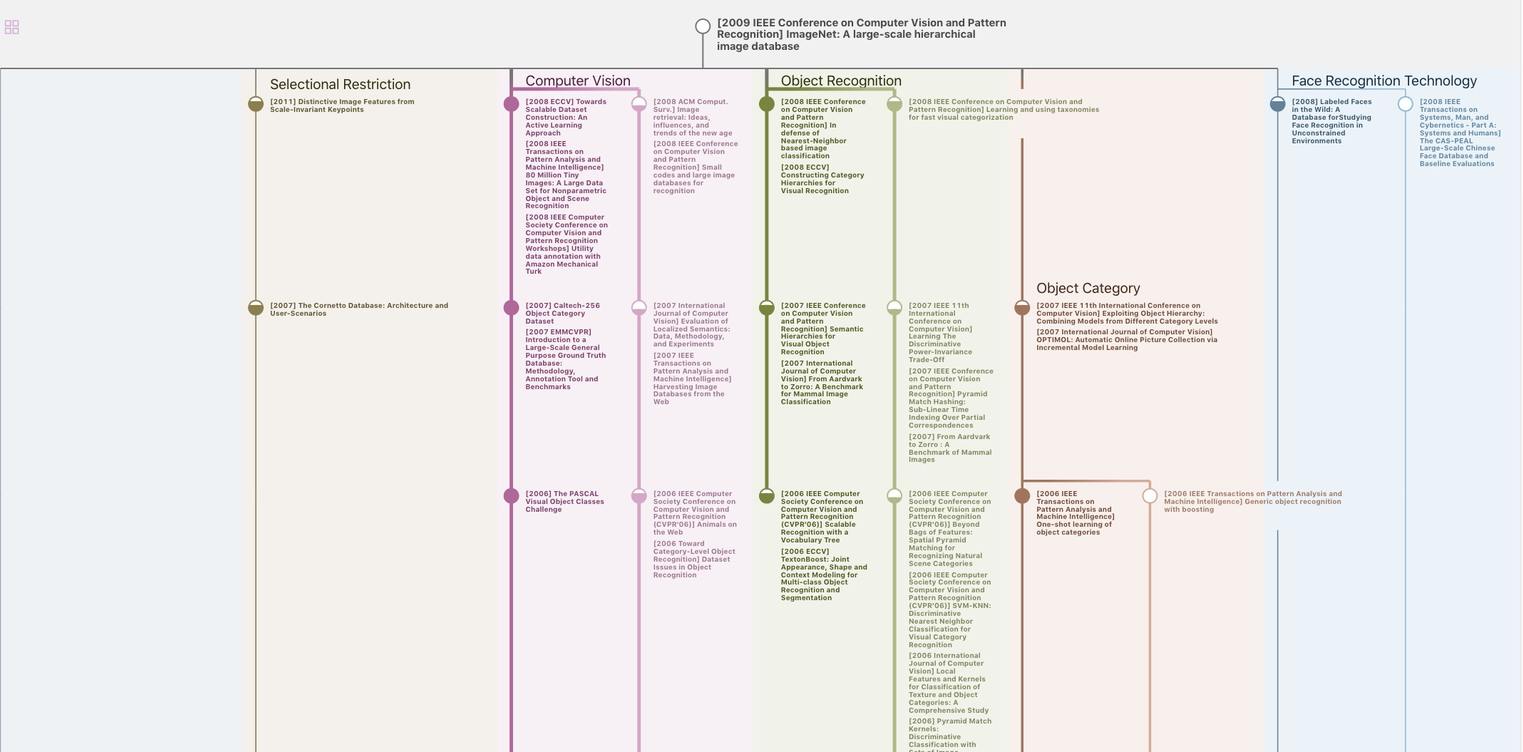
生成溯源树,研究论文发展脉络
Chat Paper
正在生成论文摘要