Social Learning with Adaptive Models
ICASSP 2024 - 2024 IEEE International Conference on Acoustics, Speech and Signal Processing (ICASSP)(2024)
摘要
In social learning, a network of agents assigns probability scores (beliefs) to some hypotheses of interest, based on the observation of streaming data. First, each agent updates locally its belief with the information extracted from the current data through a suitable likelihood model. Then, these beliefs are diffused across the network, and the agents aggregate the beliefs received from their neighbors by means of a pooling rule. This work studies social learning in the context of fully online problems, where the true hypothesis and the likelihood models can drift over time. Traditional social learning fails to address both cases. To overcome this limitation, we propose the doubly adaptive social learning (A
2
SL) strategy, which infuses traditional social learning with the necessary adaptation capabilities to face drifts in the hypotheses and/or models. The A
2
SL strategy achieves this goal by employing two adaptation stages, and we show that all agents learn well (i.e., they end up placing full belief mass on the correct hypothesis) in the regime of small adaptation parameters.
更多查看译文
关键词
Social learning,adaptation and learning,belief and opinion formation,online learning,model drift
AI 理解论文
溯源树
样例
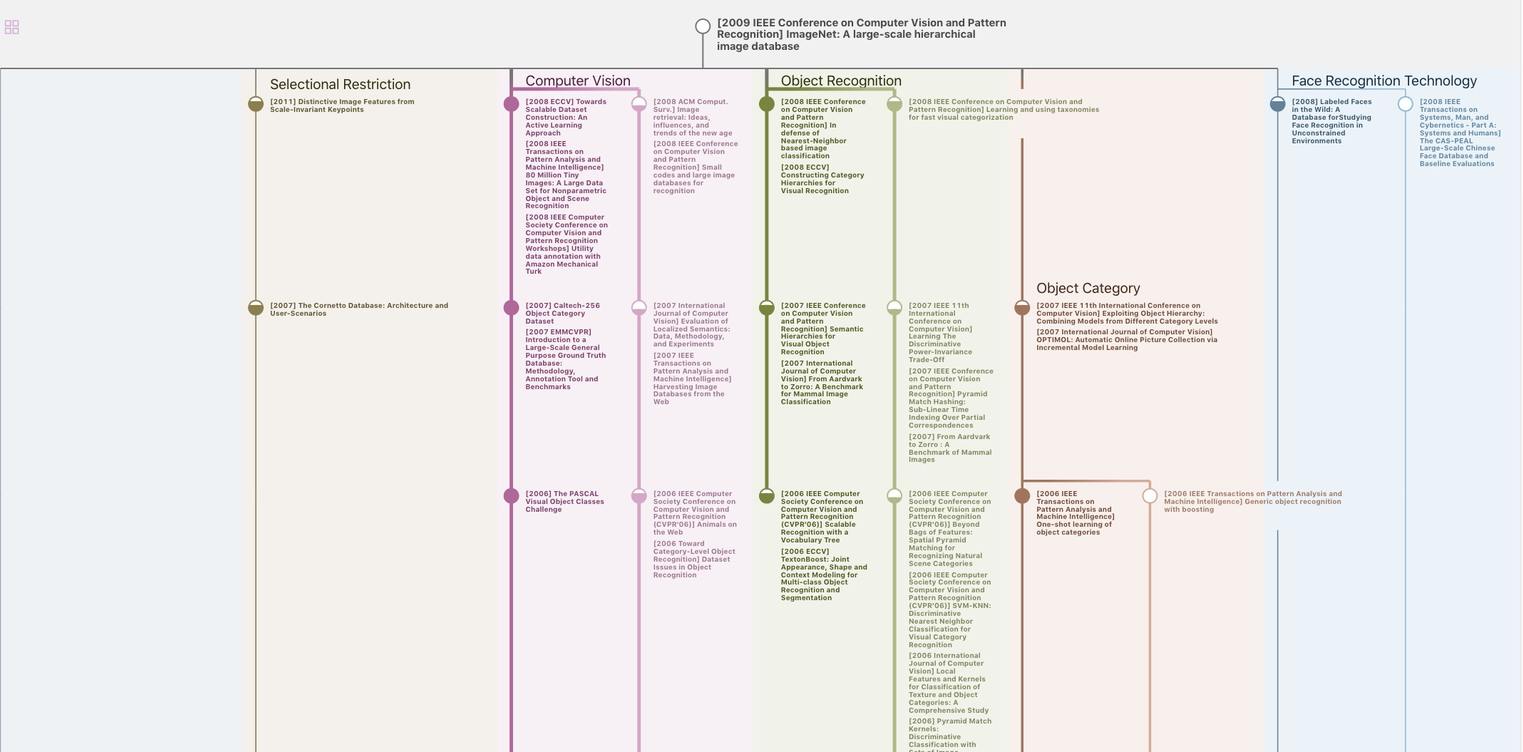
生成溯源树,研究论文发展脉络
Chat Paper
正在生成论文摘要