Incomplete Multi-View Representation Learning Through Anchor Graph-Based GCN and Information Bottleneck
ICASSP 2024 - 2024 IEEE International Conference on Acoustics, Speech and Signal Processing (ICASSP)(2024)
摘要
Real-world data often contain incomplete views with varying degrees of missing information. While there are existing methods for learning representations from such data, effectively utilizing all incomplete view data and ensuring robustness to different levels of completeness remains a challenging task. To address this problem, we propose a novel framework named IMRL-AGI. IMRL-AGI combines the anchor graph-based Graph Convolutional Network (GCN) and information bottleneck. Specifically, the framework starts by constructing an anchor graph to effectively captures the nonlinear information between instances. Next, an anchor graph-based GCN is designed to extract feature information from various views. IMRL-AGI maximizes the mutual information between the views obtained by the common representation and the anchor-graph-based GCN, ensuring the accurate extraction of view information. Furthermore, the minimization of mutual information is applied to promote diversity and reduce redundancy in the multi-view representation. Extensive experiments are conducted on several real-world datasets, and the results demonstrate the superiority of IMRL-AGI.
更多查看译文
关键词
Incomplete View Data,Information Bottleneck,Anchor Graph,Graph Convolutional Network
AI 理解论文
溯源树
样例
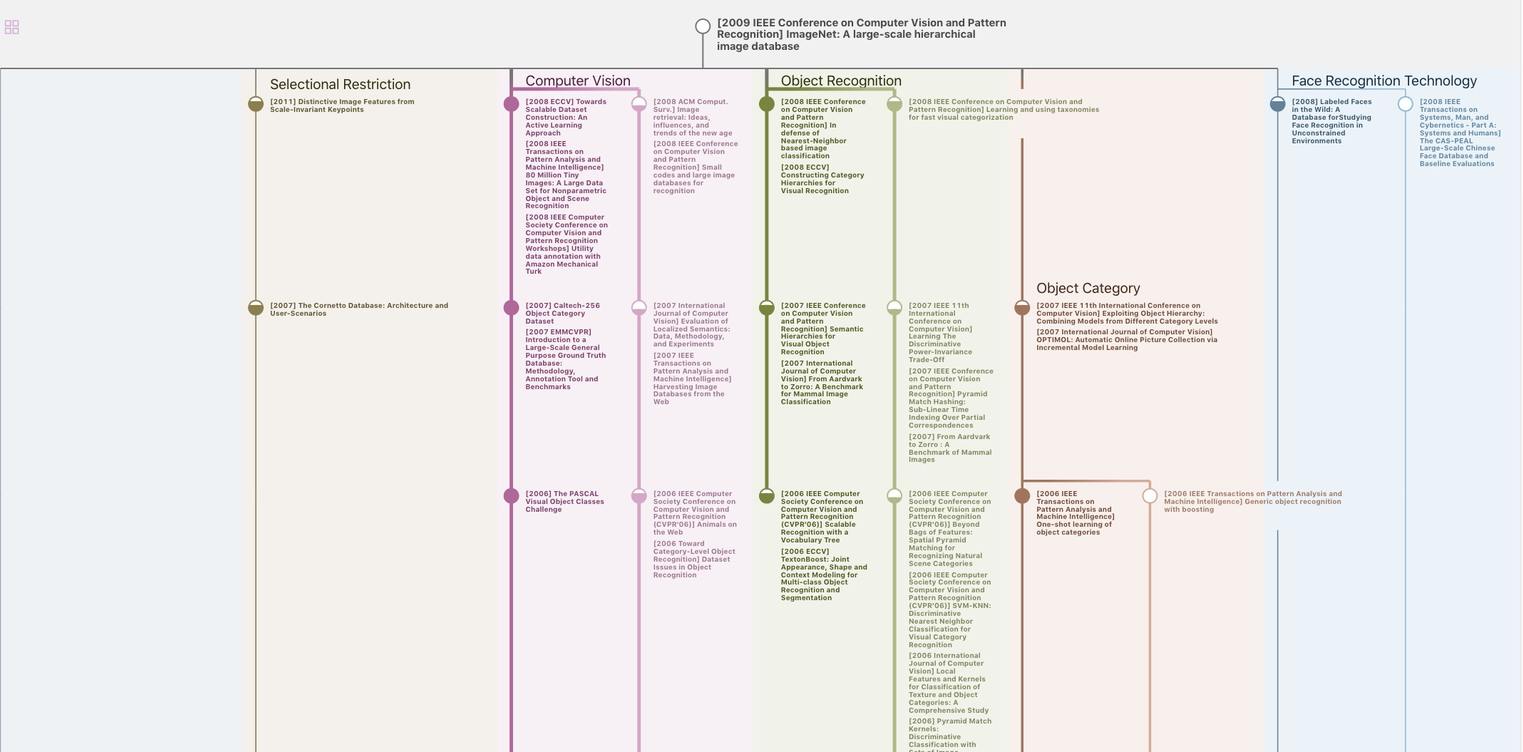
生成溯源树,研究论文发展脉络
Chat Paper
正在生成论文摘要