Diff-HOD: Diffusion Model for Object Detection in Hazy Weather Conditions
ICASSP 2024 - 2024 IEEE International Conference on Acoustics, Speech and Signal Processing (ICASSP)(2024)
摘要
The presence of haze negatively affects the visibility of captured images, posing challenges for general object detection models. We observe that current techniques exhibit three limitations: 1) they typically view image restoration and object detection as separate tasks; 2) they disregard potential details in degraded images that benefit detection; and 3) they lack sufficient recognition ability under haze interference. To this end, we propose a novel Diffusion Model (Diff-HOD) for Object Detection in Hazy weather conditions. Diff-HOD is a multi-task joint learning paradigm that integrates low-level image restoration and high-level object detection. Specifically, to bridge restoration and detection, we present a lightweight restoration module that mitigates the impact of weather-specific information, guiding the shared image encoder to provide high-quality features. We further leverage the excellent modeling ability of diffusion models to enhance the detection capability in hazy conditions. Moreover, we introduce an IoU-aware attention module that utilizes IoU as spatial priors to strengthen relevant features. Extensive experiments demonstrate that our Diff-HOD performs favorably against representative state-of-the-art approaches on both synthetic and natural datasets.
更多查看译文
关键词
Object detection,Diffusion models,Image dehazing,Joint learning
AI 理解论文
溯源树
样例
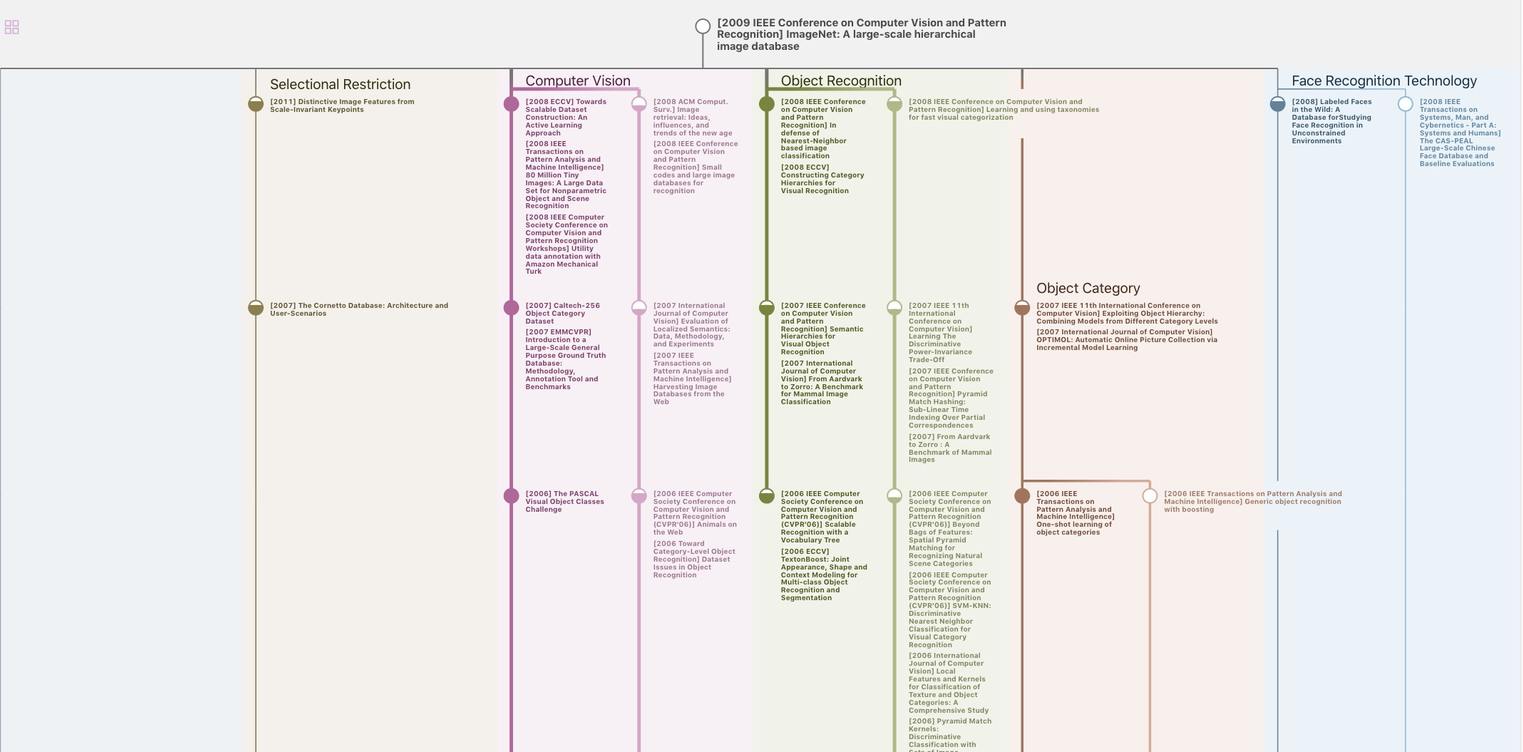
生成溯源树,研究论文发展脉络
Chat Paper
正在生成论文摘要