Modality Re-Balance for Visual Question Answering: A Causal Framework
ICASSP 2024 - 2024 IEEE International Conference on Acoustics, Speech and Signal Processing (ICASSP)(2024)
摘要
Visual Question Answering (VQA) models often prioritize language cues over visual knowledge, leading to the "language prior" phenomenon. To address this, researchers have proposed methods to balance language and image information during training and inference. However, these approaches often struggle to capture important linguistic components due to the excessive exclusion of language information. Inspired by causal inference, we introduce a novel approach called the SyMmetrically Balanced Causal framework (SMBC) that rebalances visual and textual information in VQA tasks. This framework allows for an equal contribution of knowledge from both modalities to inference results. Experimental evaluation shows that SMBC: 1) applies to prevalent VQA models, including those with data augmentation, and 2) consistently improves performance on established benchmarks.
更多查看译文
关键词
visual question answer,causal mediation analysis,language prior
AI 理解论文
溯源树
样例
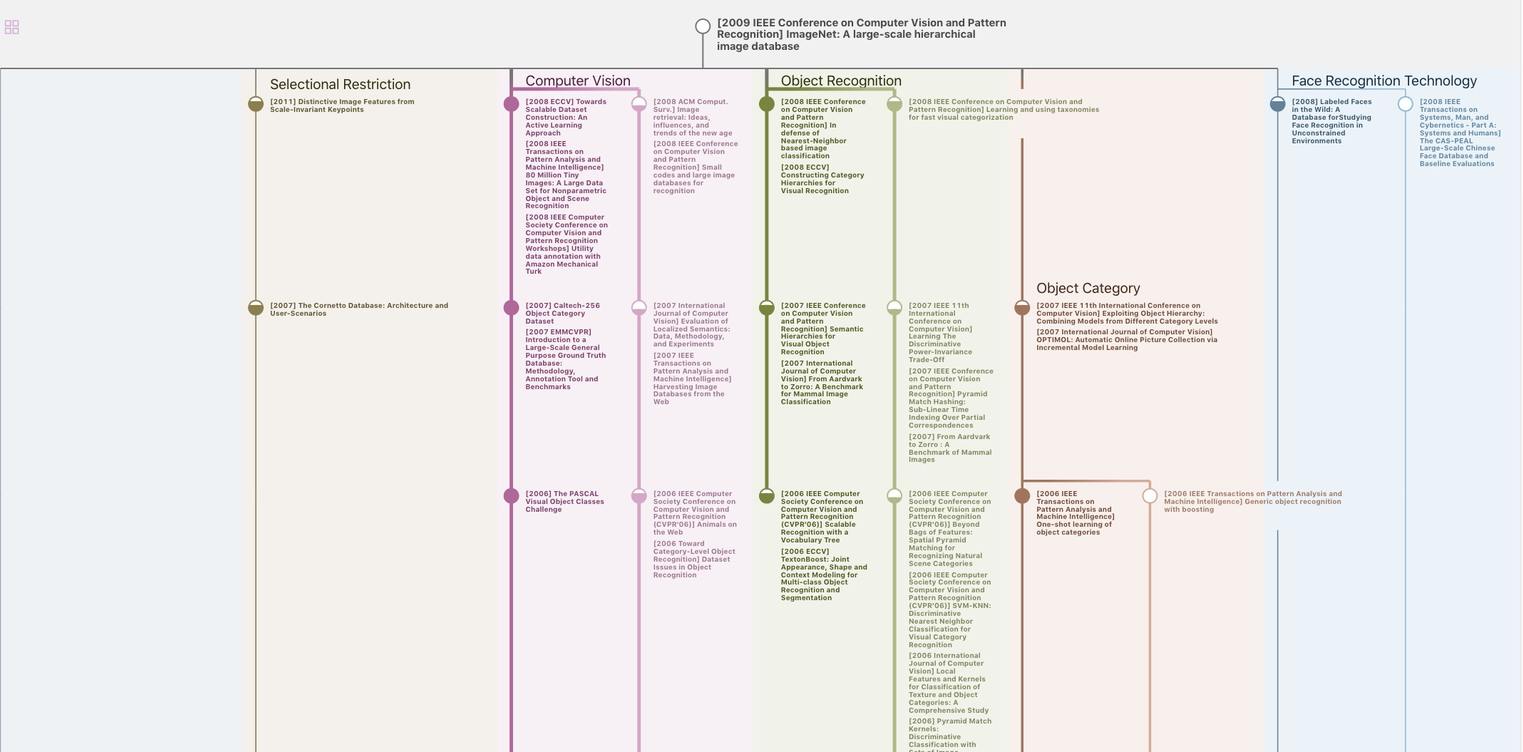
生成溯源树,研究论文发展脉络
Chat Paper
正在生成论文摘要