Patch Inherent Feature Guided Mask Selection for Image Compression
ICASSP 2024 - 2024 IEEE International Conference on Acoustics, Speech and Signal Processing (ICASSP)(2024)
摘要
Recent advances in Learned Image Compression (LIC) utilize the powerful Masked Image Modeling (MIM) framework (e.g., MAE). However, the random masking treats all patches equally, which could lead (i) missing critical information and (ii) poor robustness, since different patches may have different contributions to image reconstruction. The principle experiment shows that the patch-level inherent feature evaluation is highly correlated with the quality of the reconstructed image. Based on this key insight, we propose a simple mask selection approach based on the patch’s inherent feature. Specifically, we design plug-and-play frequency and spatial inherent feature modules to select masks, enhancing various MIMs based on the MAE model and achieving high-quality image reconstruction and strong robustness at high mask rates for data-intensive compression tasks.
更多查看译文
关键词
Image Compression,Masked Image Modeling,Masked Autoencoder
AI 理解论文
溯源树
样例
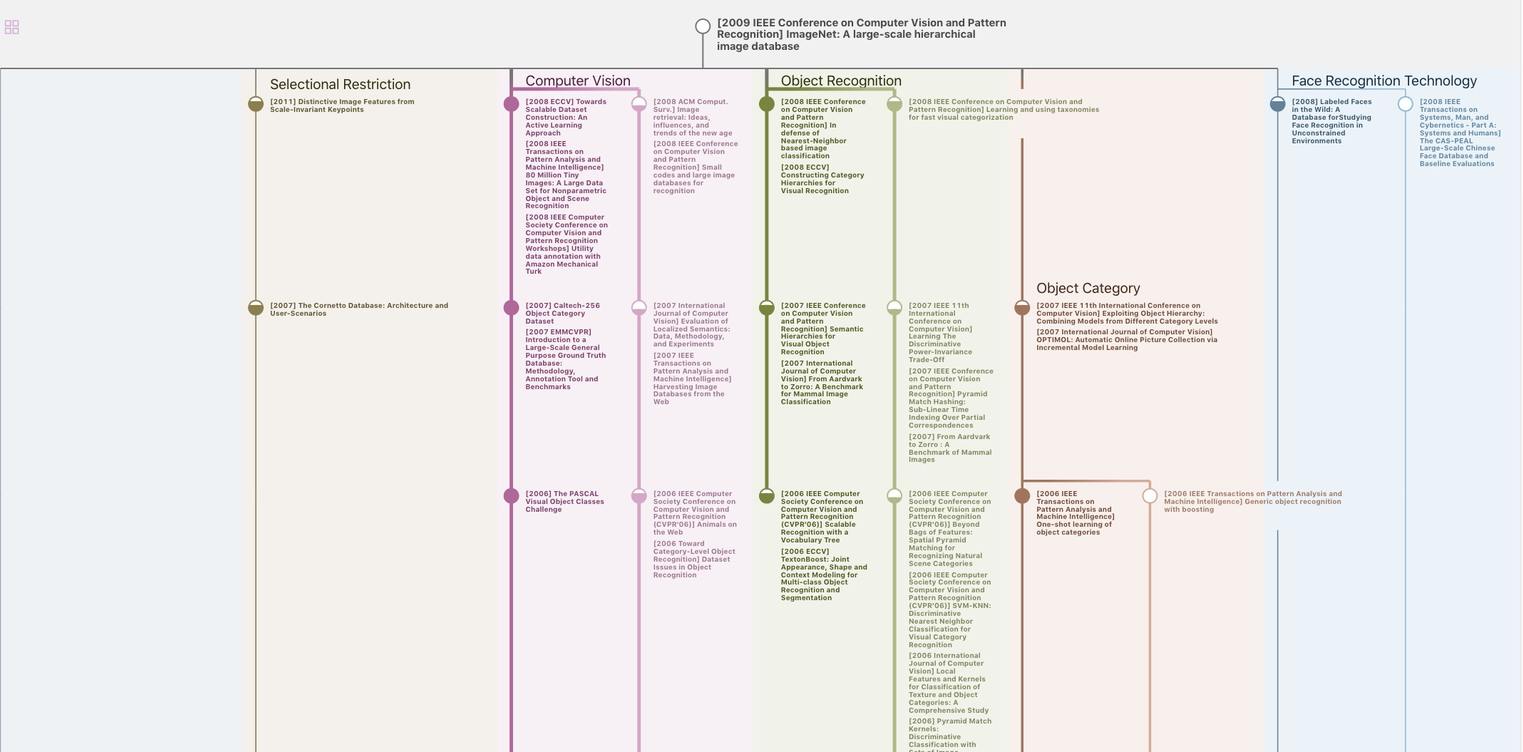
生成溯源树,研究论文发展脉络
Chat Paper
正在生成论文摘要