Accurate and Robust Scene Text Recognition via Adversarial Training
ICASSP 2024 - 2024 IEEE International Conference on Acoustics, Speech and Signal Processing (ICASSP)(2024)
摘要
Adversarial training (AT) is a methodology that utilizes adversarial examples in the training process to enhance a model’s resistance to adversarial attacks and improve generalization. Despite its efficacy in several non-sequential computer vision tasks such as classification and object detection, its effects in the realm of Scene Text Recognition (STR) remain largely unexplored. This paper pioneers an investigation into the implications of AT on STR models and proposes a novel regularization-based AT method to develop an accurate and robust STR model, dynamically generating adversarial examples in the training procedure. Through extensive experiments across seven public real-world datasets, we find that AT not only bolsters the robustness of STR models but also improves overall recognition accuracy. This improvement is particularly significant in low-resolution images - a common challenge in STR. Furthermore, given the diverse nature of real-world text images, developing a robust STR model requires a large dataset. We propose viewing AT as a form of model-based data augmentation technique for STR, compatible with traditional augmentation methods. We hope these encouraging findings catalyze further research into the application of AT for scene text recognition.
更多查看译文
关键词
Scene Text Recognition,OCR,Adversarial Training,Data Augmentation
AI 理解论文
溯源树
样例
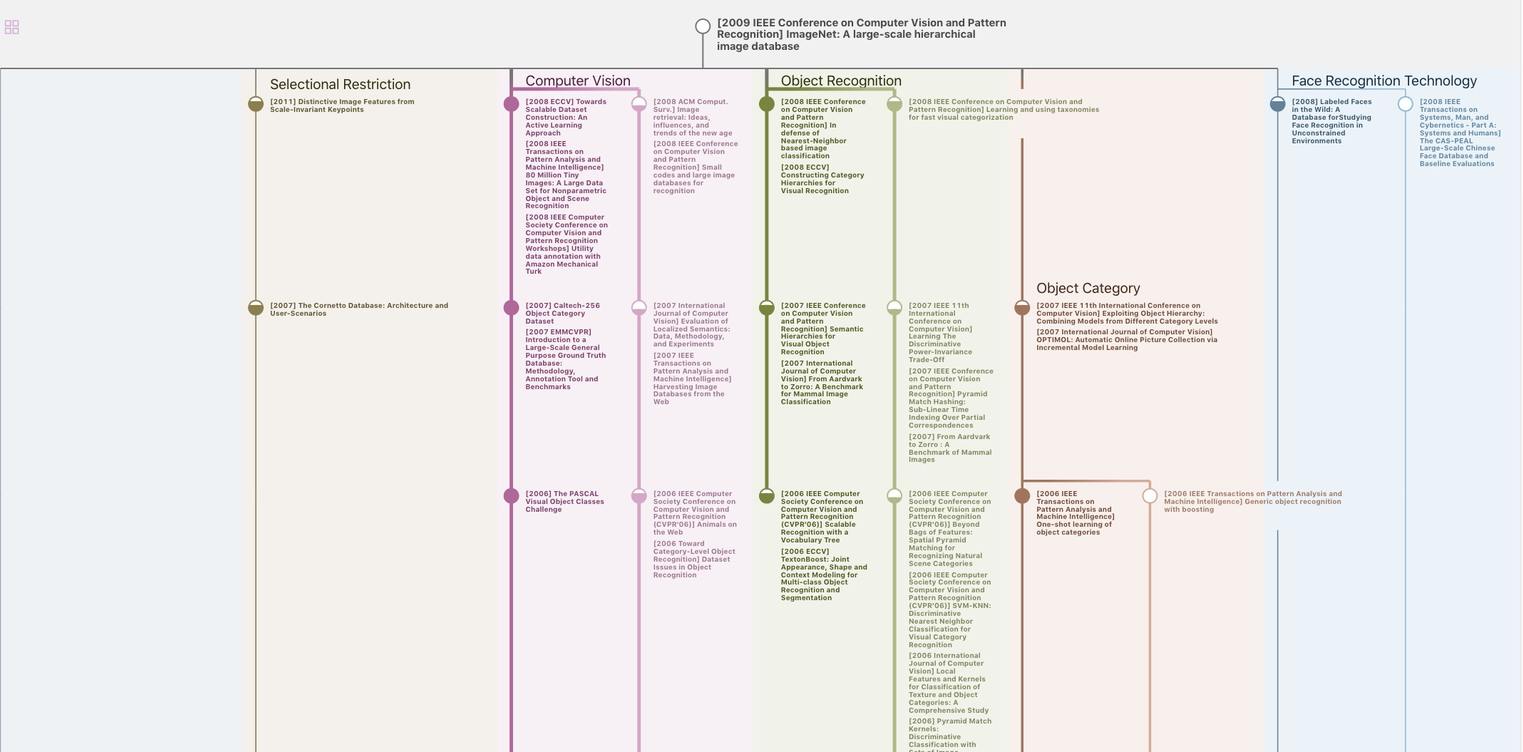
生成溯源树,研究论文发展脉络
Chat Paper
正在生成论文摘要