Enhancing the prediction of symptomatic radiation pneumonitis for locally advanced non-small-cell lung cancer by combining 3D deep learning-derived imaging features with dose–volume metrics: a two-center study
Strahlentherapie und Onkologie(2024)
摘要
This study aims to examine the ability of deep learning (DL)-derived imaging features for the prediction of radiation pneumonitis (RP) in locally advanced non-small-cell lung cancer (LA-NSCLC) patients. The study cohort consisted of 90 patients from the Fudan University Shanghai Cancer Center and 59 patients from the Affiliated Hospital of Jiangnan University. Occurrences of RP were used as the endpoint event. A total of 512 3D DL-derived features were extracted from two regions of interest (lung–PTV and PTV–GTV) delineated on the pre-radiotherapy planning CT. Feature selection was done using LASSO regression, and the classification models were built using the multilayered perceptron method. Performances of the developed models were evaluated by receiver operating characteristic curve analysis. In addition, the developed models were supplemented with clinical variables and dose–volume metrics of relevance to search for increased predictive value. The predictive model using DL features derived from lung–PTV outperformed the one based on features extracted from PTV–GTV, with AUCs of 0.921 and 0.892, respectively, in the internal test dataset. Furthermore, incorporating the dose–volume metric V30Gy into the predictive model using features from lung–PTV resulted in an improvement of AUCs from 0.835 to 0.881 for the training data and from 0.690 to 0.746 for the validation data, respectively (DeLong p < 0.05). Imaging features extracted from pre-radiotherapy planning CT using 3D DL networks could predict radiation pneumonitis and may be of clinical value for risk stratification and toxicity management in LA-NSCLC patients. Integrating DL-derived features with dose–volume metrics provides a promising noninvasive method to predict radiation pneumonitis in LA-NSCLC lung cancer radiotherapy, thus improving individualized treatment and patient outcomes.
更多查看译文
关键词
Radiation therapy,Radiation pneumonitis prediction,Deep learning-based radiomics,Machine learning,Dosimetry
AI 理解论文
溯源树
样例
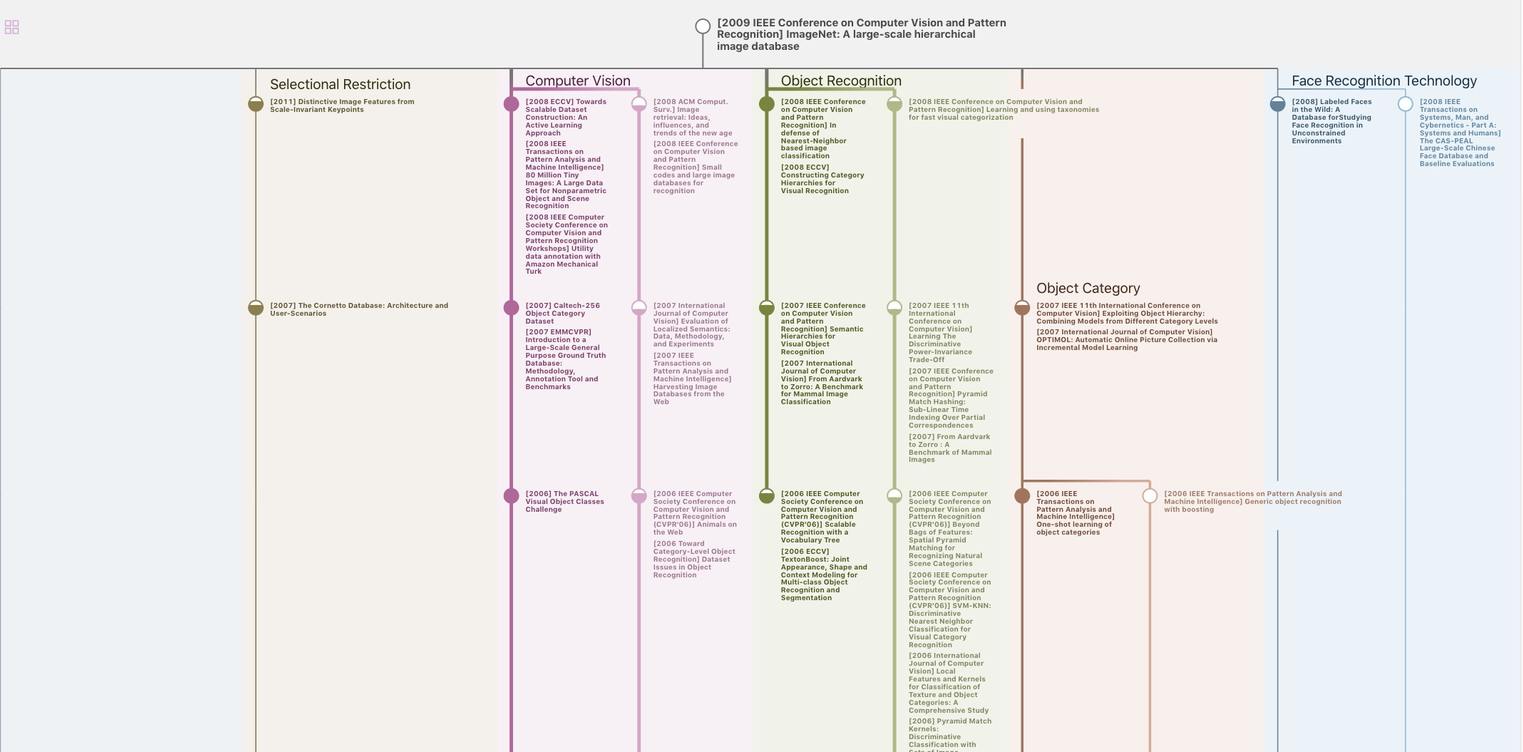
生成溯源树,研究论文发展脉络
Chat Paper
正在生成论文摘要