Generalization of Self-Supervised Learning-Based Representations for Cross-Domain Speech Emotion Recognition
ICASSP 2024 - 2024 IEEE International Conference on Acoustics, Speech and Signal Processing (ICASSP)(2024)
摘要
Self-supervised learning (SSL) from unlabelled speech data has revolutionized speech representation learning. Among them, wavLM, wav2vec2, HuBERT, and Data2vec have produced benchmark performances on automatic speech recognition. However, few studies have explored the generalization of SSL-based representations to different tasks based on paralinguistic information in speech such as emotion recognition. This paper explores the generalization of all four popular SSL models for speech emotion recognition (SER) when trained and tested in different domains. We aim to understand how adaptable these SSL representations are when using simple domain adaptation techniques. The evaluation considers emotional speech databases that deviate in language, recording conditions, and emotional distribution, providing very different target domains. The results reveal the necessity to fine-tune the representations for the SER downstream. As the differences between the source and target domain increase, we observe that the unsupervised domain adaptation techniques are more effective. The analysis in this study provides useful insights to understand the advantages of different representations for domain adaptation in SER.
更多查看译文
关键词
Speech emotion recognition,self-supervised learning,unsupervised domain adaptation
AI 理解论文
溯源树
样例
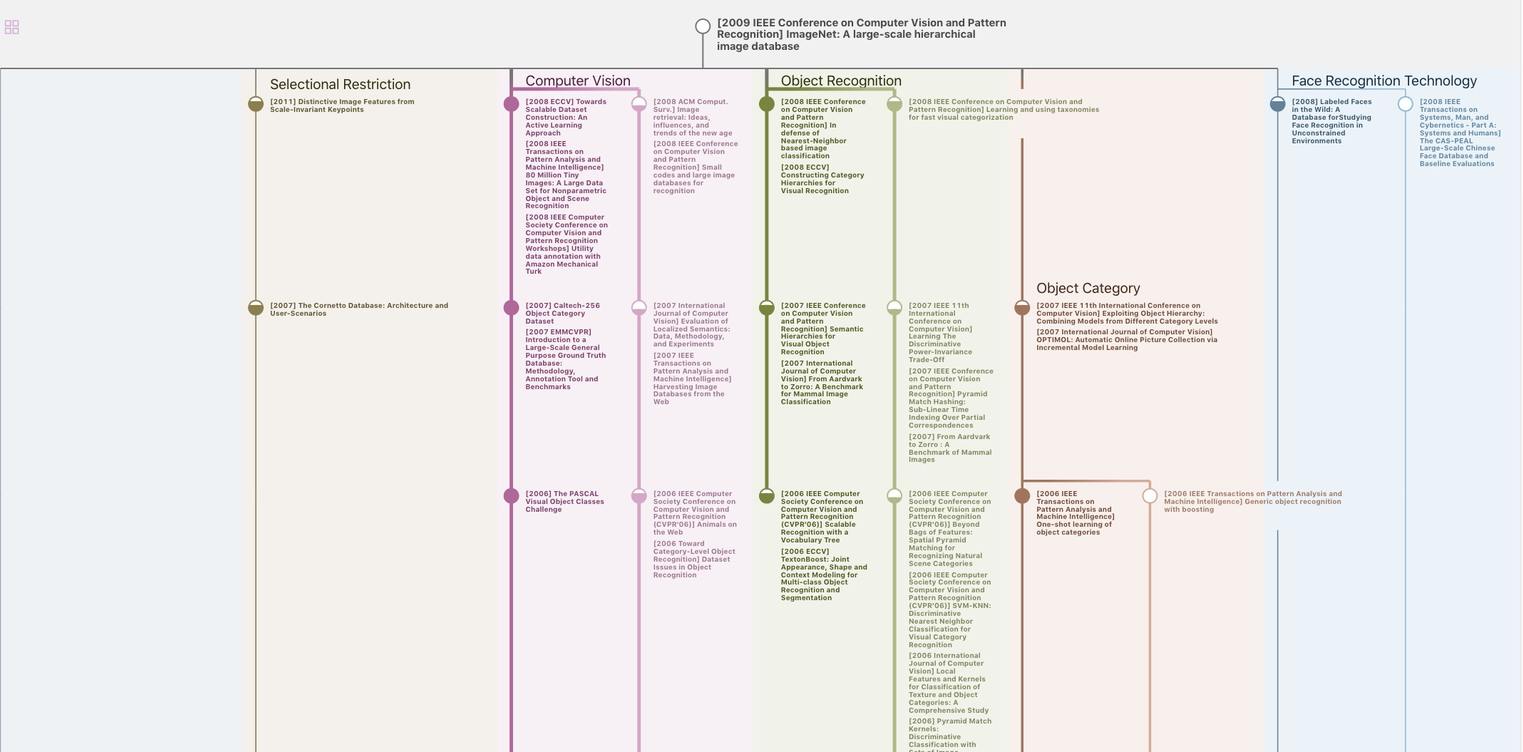
生成溯源树,研究论文发展脉络
Chat Paper
正在生成论文摘要