Predicting the rotational dependence of line broadening using machine learning
Journal of Molecular Spectroscopy(2024)
摘要
Correct pressure broadening is essential for modelling radiative transfer in atmospheres, however data are lacking for the many exotic molecules expected in exoplanetary atmospheres. Here we explore modern machine learning methods to mass produce pressure broadening parameters for a large number of molecules in the ExoMol data base. To this end, state-of-the-art machine learning models are used to fit to existing, empirical air-broadening data from the HITRAN database. A computationally cheap method for large-scale production of pressure broadening parameters is developed, which is shown to be reasonably (69%) accurate for unseen active molecules. This method has been used to augment the previously insufficient ExoMol line broadening diet, providing air-broadening data for all ExoMol molecules, so that the ExoMol database has a full and more accurate treatment of line broadening. Suggestions are made for improved air-broadening parameters for species present in atmospheric databases.
更多查看译文
关键词
Machine learning,Line broadening
AI 理解论文
溯源树
样例
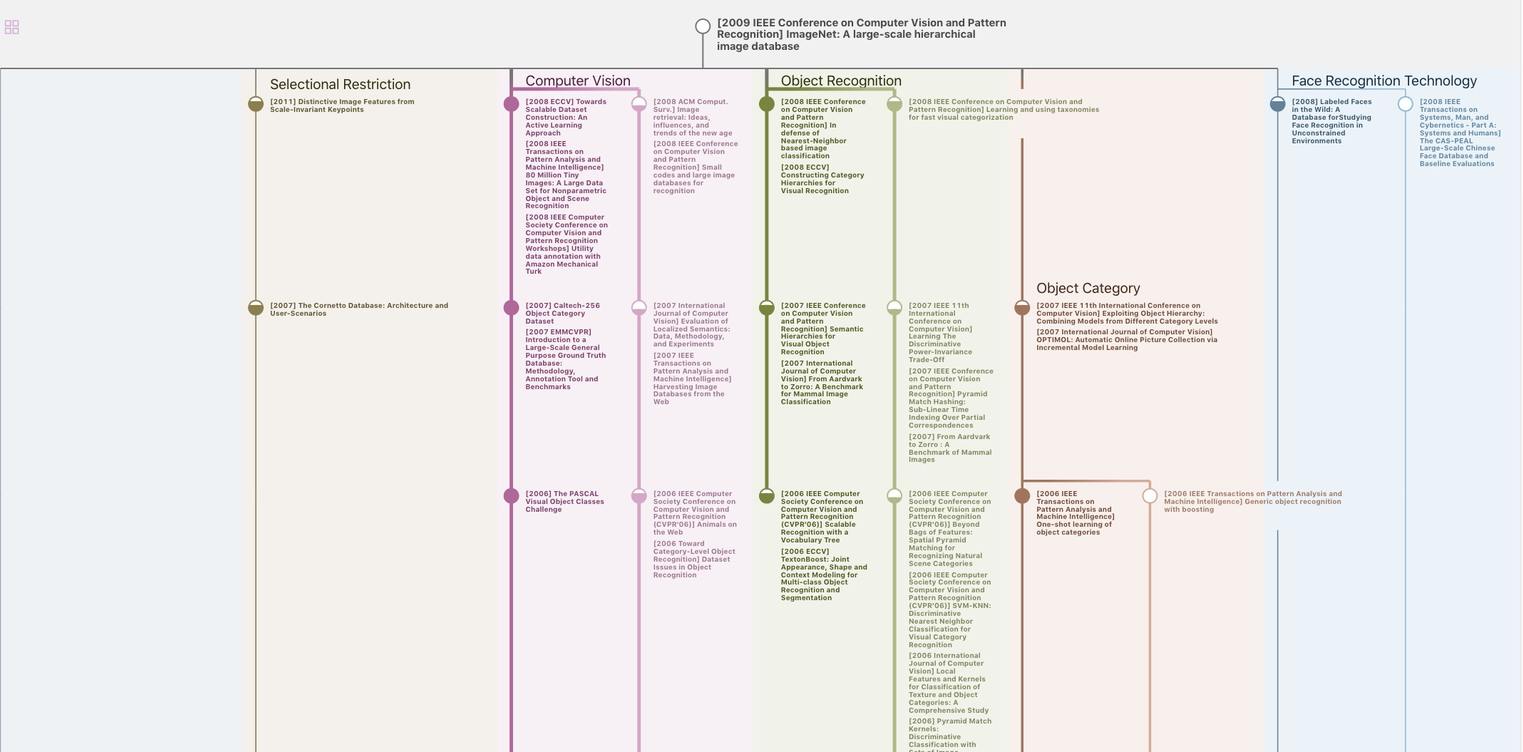
生成溯源树,研究论文发展脉络
Chat Paper
正在生成论文摘要