Differentially Private Federated Frank-Wolfe
ICASSP 2024 - 2024 IEEE International Conference on Acoustics, Speech and Signal Processing (ICASSP)(2024)
Abstract
In this paper, we propose DP-FedFW, a novel Frank-Wolfe based federated learning algorithm with local (ϵ,δ)-differential privacy (DP) guarantees in a constrained learning setting. In DP-FedFW, we perturb local models to ensure privacy while communicating with the server, and each client performs several Frank-Wolfe steps to arrive at a local model. The proposed method guarantees (ϵ,δ)-DP for each client and has a sublinear convergence of $\mathcal{O}$(1/k) for smooth convex objective functions, where k is the number of communication rounds and an asymptotic convergence for smooth non-convex objective functions. The theoretical analysis shows that given an (ϵ,δ)-DP requirement, the proposed algorithm’s performance improves with the number of clients and the batch size. We empirically validate the efficacy of the proposed method on several constrained machine learning tasks.
MoreTranslated text
Key words
Constrained learning,differential privacy,federated learning,Frank-Wolfe
AI Read Science
Must-Reading Tree
Example
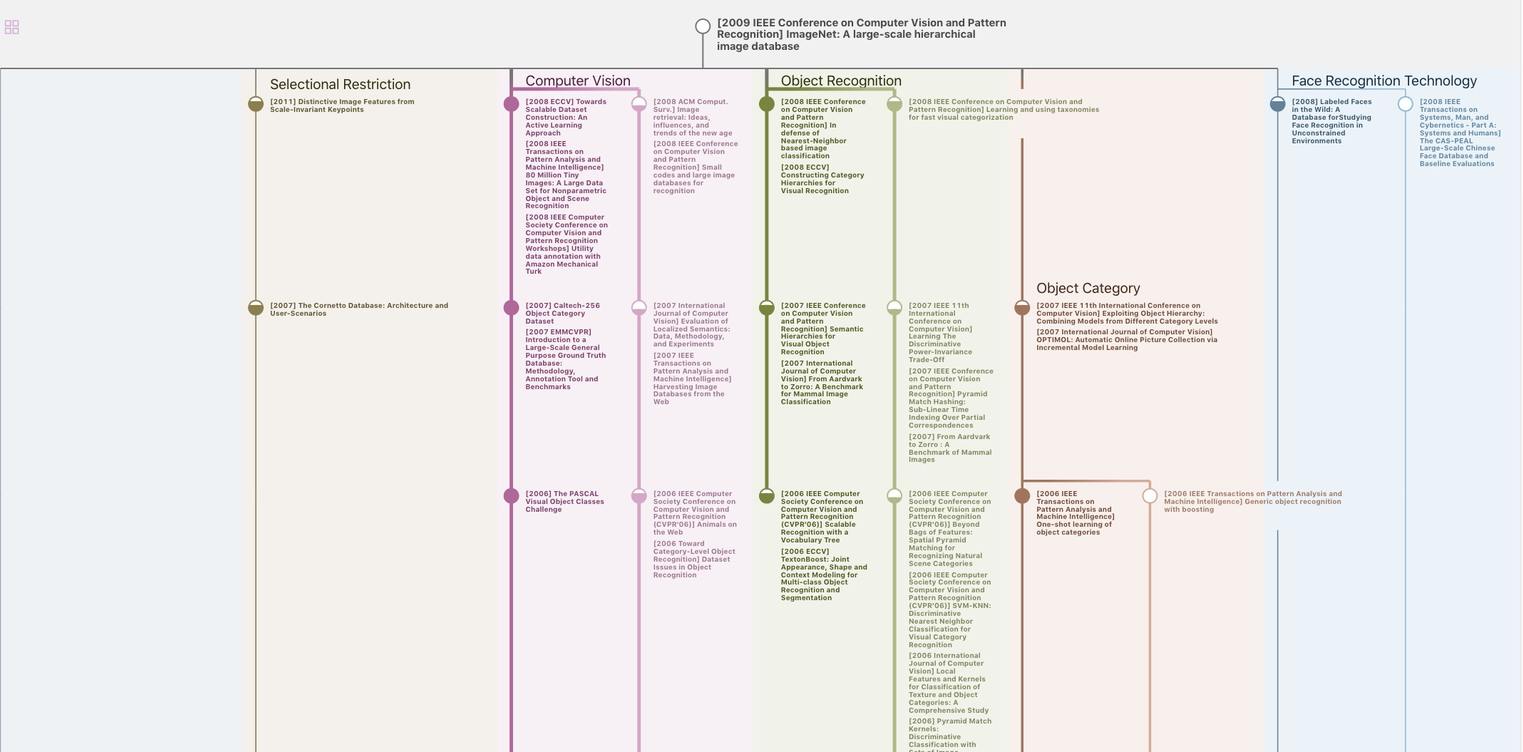
Generate MRT to find the research sequence of this paper
Chat Paper
Summary is being generated by the instructions you defined