Sample Adaptive Transfer for Electricity Theft Detection With Distribution Shifts
IEEE Transactions on Power Systems(2024)
Abstract
Recently, there has been a growing interest in using supervised machine learning models to identify electricity thieves. A dominant assumption in most existing studies is that a large number of labeled electricity consumption readings are available for effective model training. However, this assumption does not hold in new cities where labeled electricity consumption readings are scarce. To solve this problem, a novel sample adaptive transfer strategy is proposed to improve the model performance of electricity theft detection in new cities with few labeled electricity consumption readings. In particular, labeled electricity consumption readings from another city are adaptively transferred to the target city, taking into account their distribution shifts affected by consumption behaviors. Extensive investigations are performed on popular machine learning models and multiple datasets to thoroughly test the proposed strategy. Simulation results show that the proposed sample adaptive transfer outperforms baselines with an increase of about 18.83% and 13.29% for mean average precision (MAP) and area under curve (AUC), respectively. Further, the proposed strategy has strong generalizability to a wide range of fraudulent ratios as well as machine learning models in new cities with few labeled samples.
MoreTranslated text
Key words
Electricity theft,smart grid,smart meter,machine learning,transfer learning,distribution shift
AI Read Science
Must-Reading Tree
Example
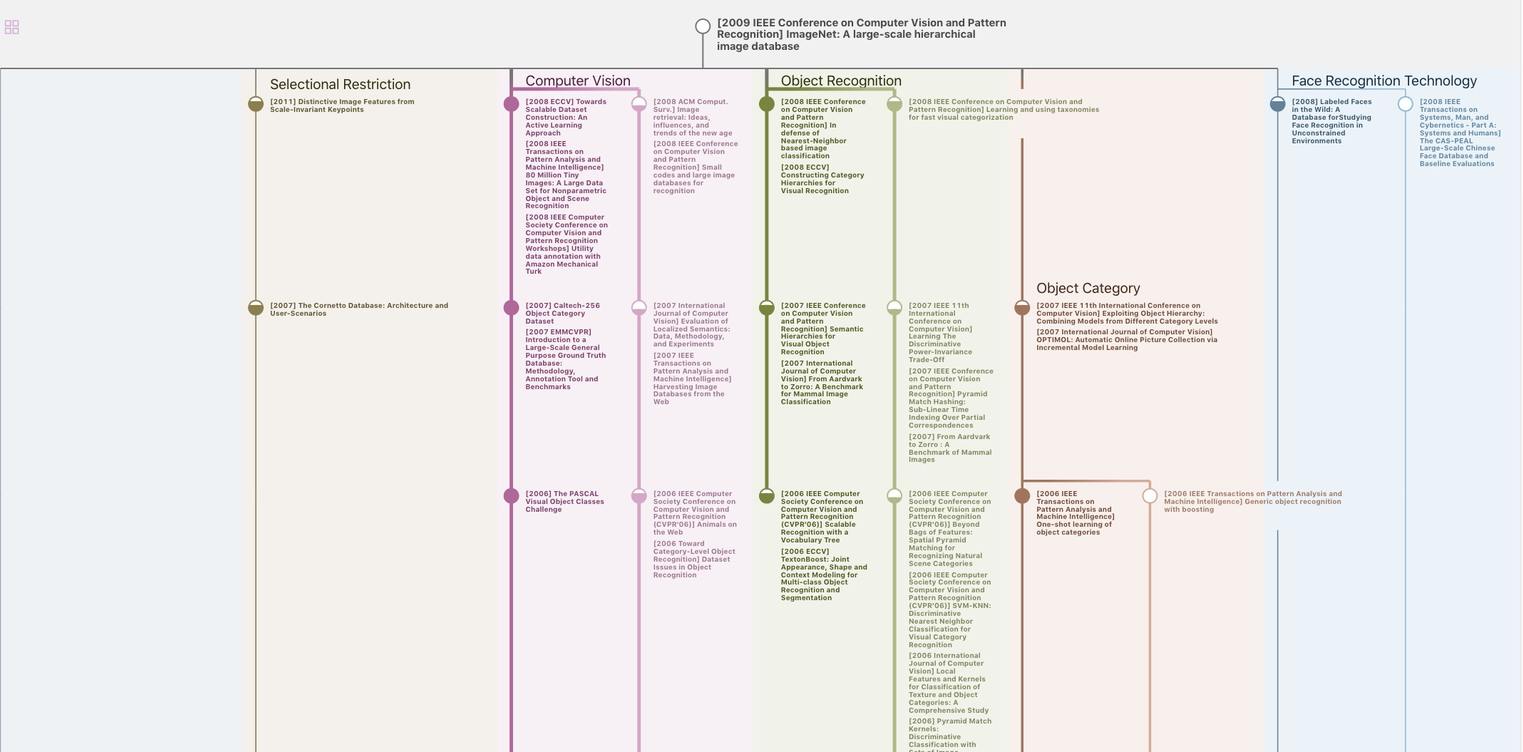
Generate MRT to find the research sequence of this paper
Chat Paper
Summary is being generated by the instructions you defined