Self-Training Domain Adaptation Via Weight Transmission Between Generators.
IEEE International Conference on Acoustics, Speech, and Signal Processing(2024)
摘要
Unsupervised domain adaptation (UDA) aims to transfer knowledge from the labeled source domain to the fully-unlabeled target domain, thus improving the classification performance of the target domain. Recently, self-training has shown its effectiveness on UDA. However, the feature space for generating pseudo-labels contains a large amount of source information, making it challenging for the generator to learn discriminative features of the target domain. In this paper, we propose a self-training domain adaptation model via weight transmission between generators (WTBG). Specifically, we develop a bi-directional transmission structure for generators, using Exponential Moving Average (EMA) as the bridge between two generators. By cyclically transmitting weight parameters between them, alleviate the difficulty of generators in learning target features. And a pseudo-label filter based on cosine similarity is designed to reduce the influence of error pseudo-labels. Extensive experiments conducted on two benchmark UDA datasets show that WTBG has superior classification performance.
更多查看译文
关键词
Domain adaptation,Self-training,Image classification
AI 理解论文
溯源树
样例
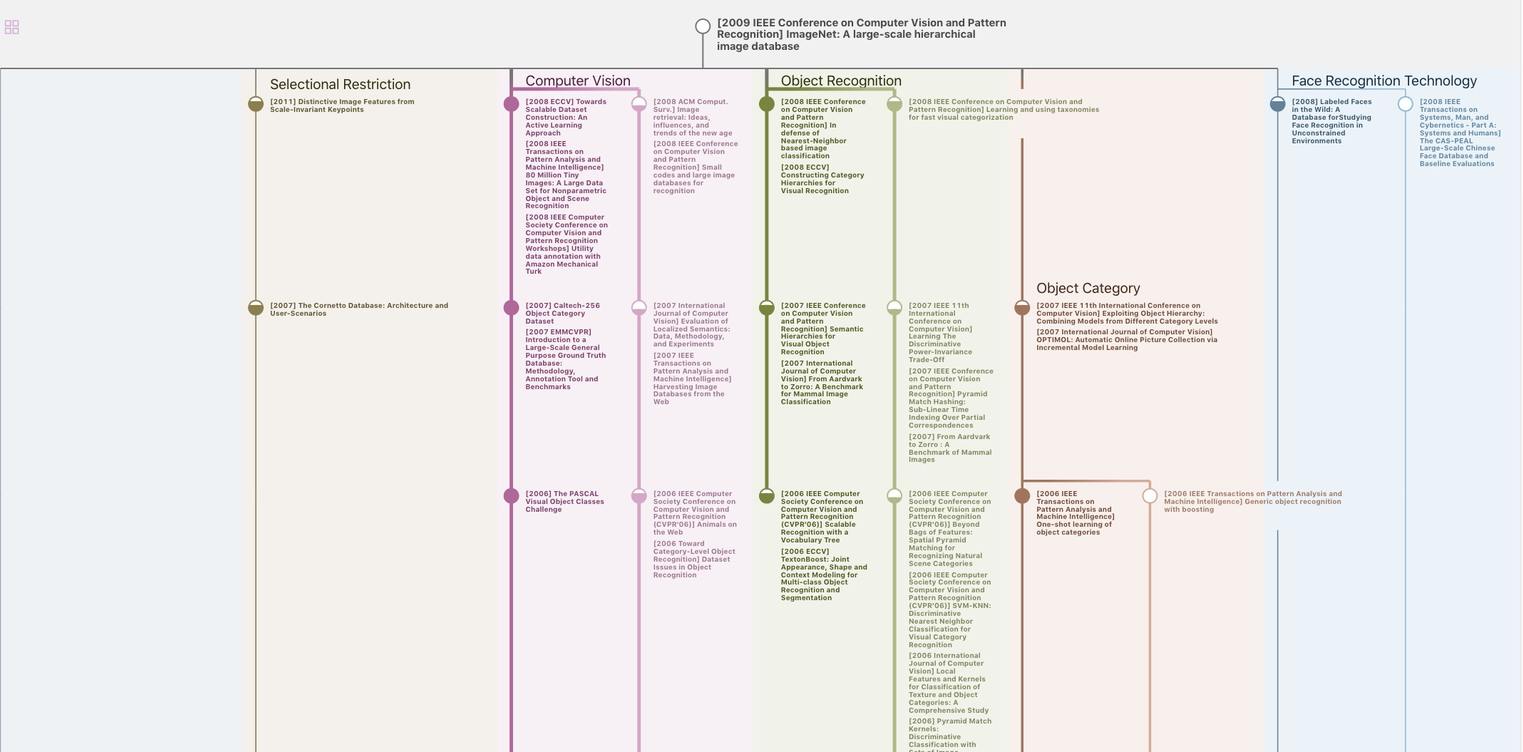
生成溯源树,研究论文发展脉络
Chat Paper
正在生成论文摘要