Edge-Oriented Point Cloud Compression by Moving Object Detection for Realtime Smart Monitoring.
Consumer Communications and Networking Conference(2024)
摘要
Smart traffic monitoring at intersections which exploits three-dimensional light detection and ranging (LiDAR) sensor networks is a promising technique for achieving a safe and secure society. One challenge for widely spreading these systems is to effectively aggregate massive point cloud data generated by multiple LiDAR sensors installed at every corner of intersections with a limited cost and a limited communication bandwidth. To this end, this paper proposes a lightweight point cloud compression method for real-time smart traffic monitoring. The proposed method enables tiny low-cost processors installed at every LiDAR sensor to detect moving parts of a point cloud from point could data in real time. By sending compressed data of moving parts of a point cloud only to edge servers, the communication bandwidth is saved, which helps edge servers to analyze them for preventing traffic accidents in real time. Experimental results using the KoPER intersection dataset show that the average detection rate over 1,200 frames of data is around 95%. The processing time per frame is about 5.4 ms with a commercial edge-oriented processor, which is less than typical frame rates of modern LiDAR sensors. In addition, point could compression ratio of the proposed method is approximately 3.5 times better than that without the moving part detection technique.
更多查看译文
关键词
Smart monitoring,LiDAR,Point cloud compression
AI 理解论文
溯源树
样例
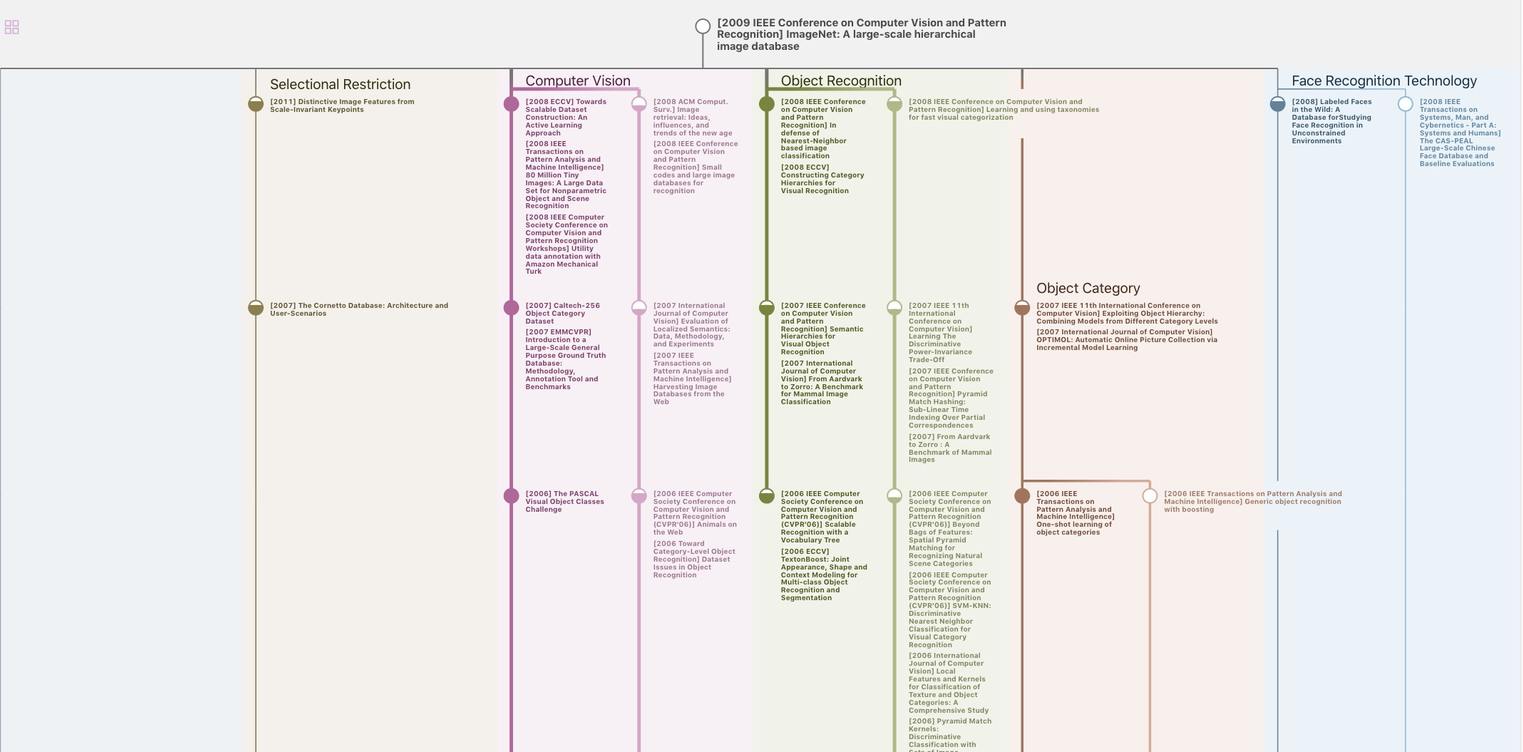
生成溯源树,研究论文发展脉络
Chat Paper
正在生成论文摘要