A Method for Bilevel Optimization with Convex Lower-Level Problem
ICASSP 2024 - 2024 IEEE International Conference on Acoustics, Speech and Signal Processing (ICASSP)(2024)
摘要
Gradient-based bilevel optimization methods have been applied to a wide range of applications including hyper-parameter optimization, meta-learning, and model pruning. However, it is known that the bilevel optimization problem is difficult to solve, and the finite-time guarantee has only been established for simpler bilevel problems with a strongly-convex lower-level problem. In this work, we propose an iterative bilevel optimization method that sequentially solves simple approximate problems of the original problem. Despite the lack of strong convexity in the lower level, we show that the proposed method converges to an ϵ-stationary-point with an iteration complexity of $\mathcal{O}\left( {{\varepsilon ^{ - 1}}} \right)$. Experiments have verified the effectiveness of the method.
更多查看译文
关键词
Bilevel optimization,difference-of-convex,convex-concave procedure,hyperparameter tunning
AI 理解论文
溯源树
样例
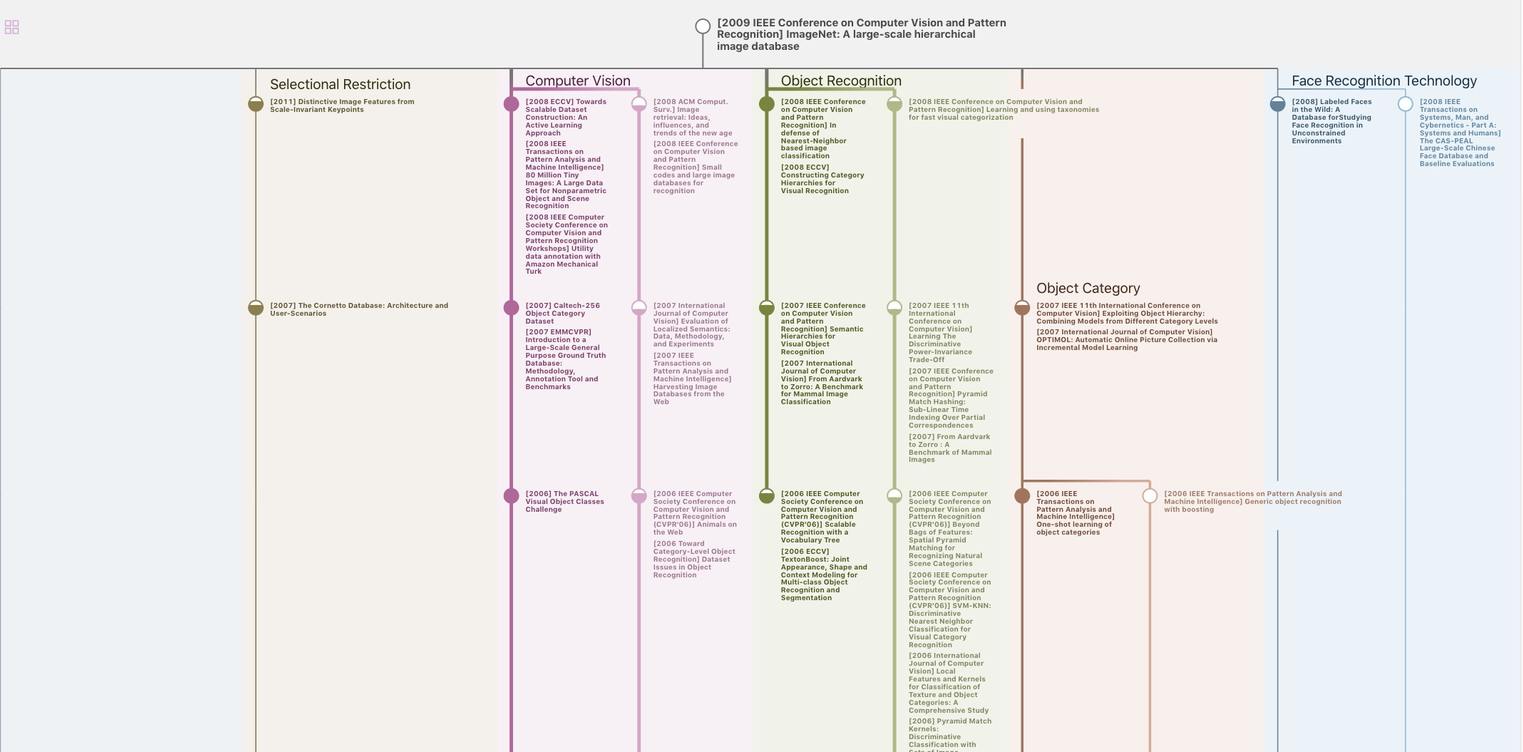
生成溯源树,研究论文发展脉络
Chat Paper
正在生成论文摘要