Temporal Pattern Mining for Knowledge Discovery in the Early Prediction of Septic Shock
Pattern Recognition(2024)
Abstract
Temporal pattern mining can be employed to detect patterns and trends in a patient's health status as it evolves over time. However, these methods often produce an overwhelming number of patterns, impeding knowledge discovery and practical implementation in acute care settings. To address this, we propose a framework that focuses on identifying a concise set of relevant temporal patterns and static variables from electronic health records for the early prediction of septic shock. Sepsis is caused by an adverse immune response to infection that triggers widespread inflammation throughout the body, which can progress to septic shock and ultimately result in death if not treated promptly. The analysis of health state patterns in sepsis patients over time offers the potential to predict septic shock prior to its onset, enabling proactive healthcare interventions. Our framework incorporates a temporal pattern mining method and four feature selection techniques. We discover that selecting features based on a model-based wrapper approach yields the highest prediction performance among these techniques. On the other hand, the use of information value identifies more multi-state patterns with abnormal health states, providing healthcare providers with valuable indicators of patient deterioration.
MoreTranslated text
Key words
Temporal Pattern Mining,Feature Selection,Electronic Health Records,Knowledge Discovery,Sepsis
AI Read Science
Must-Reading Tree
Example
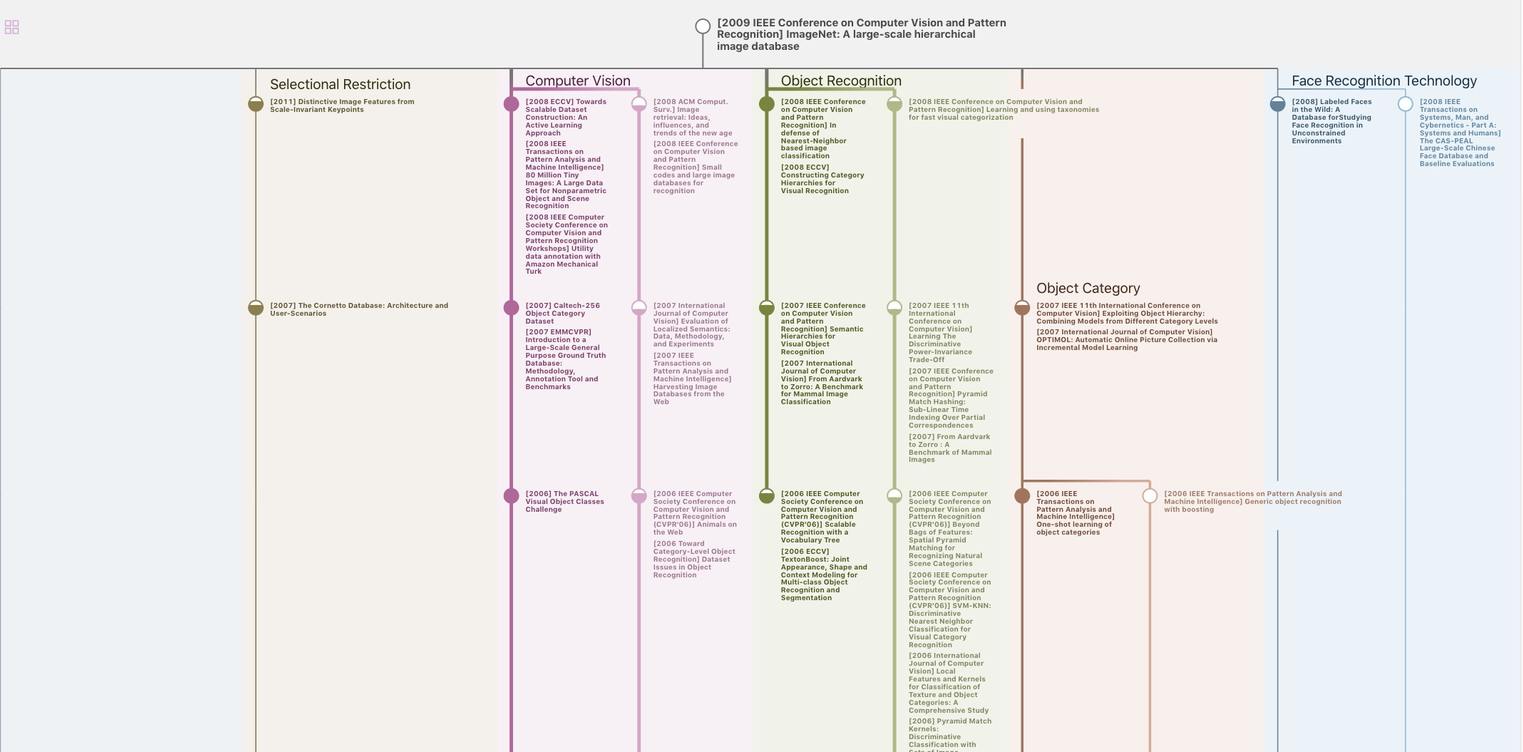
Generate MRT to find the research sequence of this paper
Chat Paper
Summary is being generated by the instructions you defined