A generative adversarial network using residual feature fusion for JPEG restoration and anti-forensics
crossref(2024)
摘要
Abstract JPEG image recovery involves eliminating artifacts introduced by JPEG compression, while anti-forensics specifically targets the removal of image processing traces caused by compression. Despite recent improvements in removing compression artifacts and anti-forensics using (generative adversarial networks) GANs, the high number of parameters makes it computationally complex and often overlooks certain hierarchical features. To address these issues, we propose a novel Residual Feature Fusion (RFF) framework as the backbone network of the generator. This framework has two structures that fuse residuals, made up of multiple modules. It adds skip connections to directly incorporate forward features in each local remaining branch. Furthermore, to enhance the capabilities of the RFF framework, we introduce an Improved Spatial Attention (ISA) block. The ISA block enhances the focus of the remaining features on the critical spatial content. Our final generator network is constructed by applying the proposed RFF framework and ISA blocks. Experimental results show that our proposed method outperforms existing JPEG anti-forensics techniques in terms of image quality while maintaining comparable anti-forensics performance.
更多查看译文
AI 理解论文
溯源树
样例
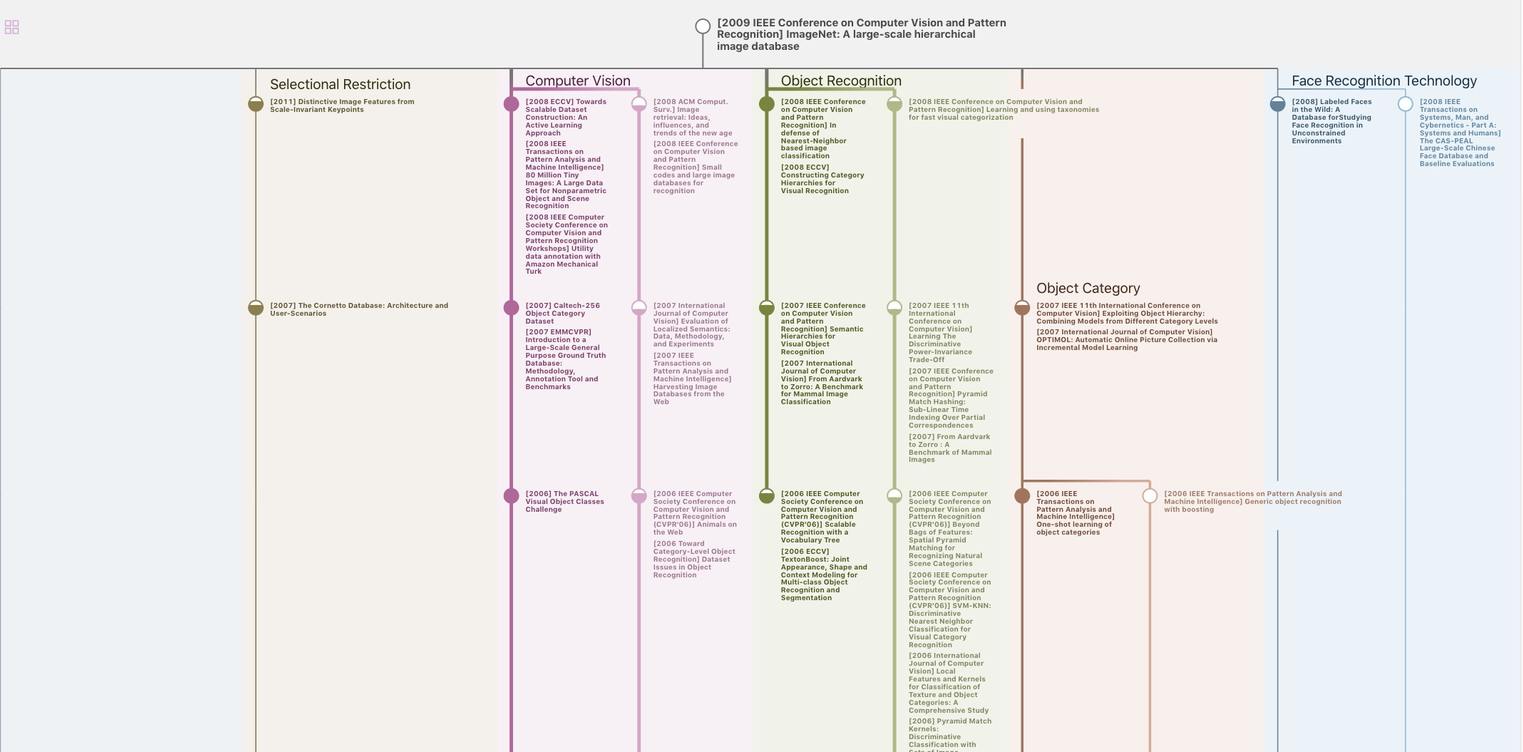
生成溯源树,研究论文发展脉络
Chat Paper
正在生成论文摘要