Facilitating Message Passing with Potential Links for Knowledge Graph Completion
ICASSP 2024 - 2024 IEEE International Conference on Acoustics, Speech and Signal Processing (ICASSP)(2024)
摘要
Knowledge graph completion (KGC) aims at inferring missing links between two entities. Most previous models focus on learning representations for entities and relations via graph neural networks. In this formalism, representations heavily rely on structural information. However, it is common for Knowledge graphs (KGs) to be unconnected due to their inherent incompleteness, resulting in a significant loss of vital structural information. To overcome this issue, this paper proposes to increase the connectedness of KGs to facilitate message passing between entities. Specifically, we first augment KGs with a series of auxiliary triplets derived from outer nodes. Subsequently, a dynamically weighted graph convolutional layer is employed to unequally aggregate the representations of neighboring entities and dynamically combine this aggregated information with information from themselves. Finally, ConvE is utilized to calculate scores for all triplets. Extensive experiments on two benchmark datasets demonstrate the superiority of our method compared to strong baseline models.
更多查看译文
关键词
Knowledge graph completion,knowledge graph augmentation,graph convolutional network
AI 理解论文
溯源树
样例
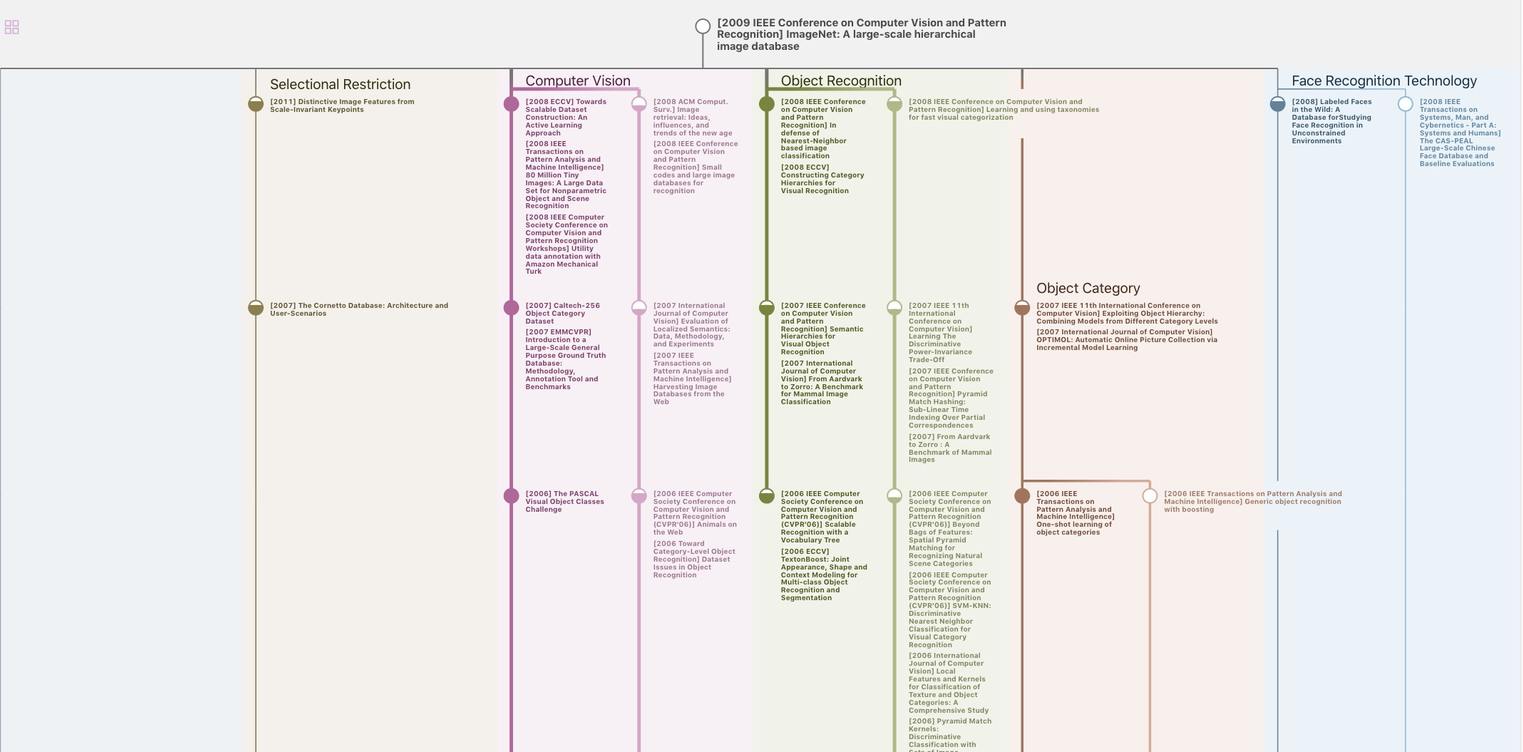
生成溯源树,研究论文发展脉络
Chat Paper
正在生成论文摘要