Efficient Hierarchical Stripe Attention for Lightweight Image Super-Resolution
ICASSP 2024 - 2024 IEEE International Conference on Acoustics, Speech and Signal Processing (ICASSP)(2024)
摘要
Following the successful integration of Transformers in computer vision, several Transformer-based approaches have emerged, surpassing the previously dominant CNN-based techniques. However, it is observed that conventional self-attention involves redundant operations that can be further optimized. In this context, we propose a novel framework for lightweight super-resolution, termed as Hierarchical Stripe-attention Super-Resolution Network (HSSRNet). Specifically, our approach involves the fusion of correlated patches in each layer to access global pixel information. Subsequently, we introduce a stripe intra-patch self-attention (stripe IPSA) mechanism to model long-range dependencies efficiently. To further reduce computational complexity, we approximate the original attention map using two low-rank matrices. Finally, we employ PixelShuffle in conjunction with convolutions for upsampling. Extensive experiments demonstrate the effectiveness of our proposed modules, and the results indicate that our method achieves commendable performance on four benchmark datasets.
更多查看译文
关键词
image SR,stripe attention,lightweight
AI 理解论文
溯源树
样例
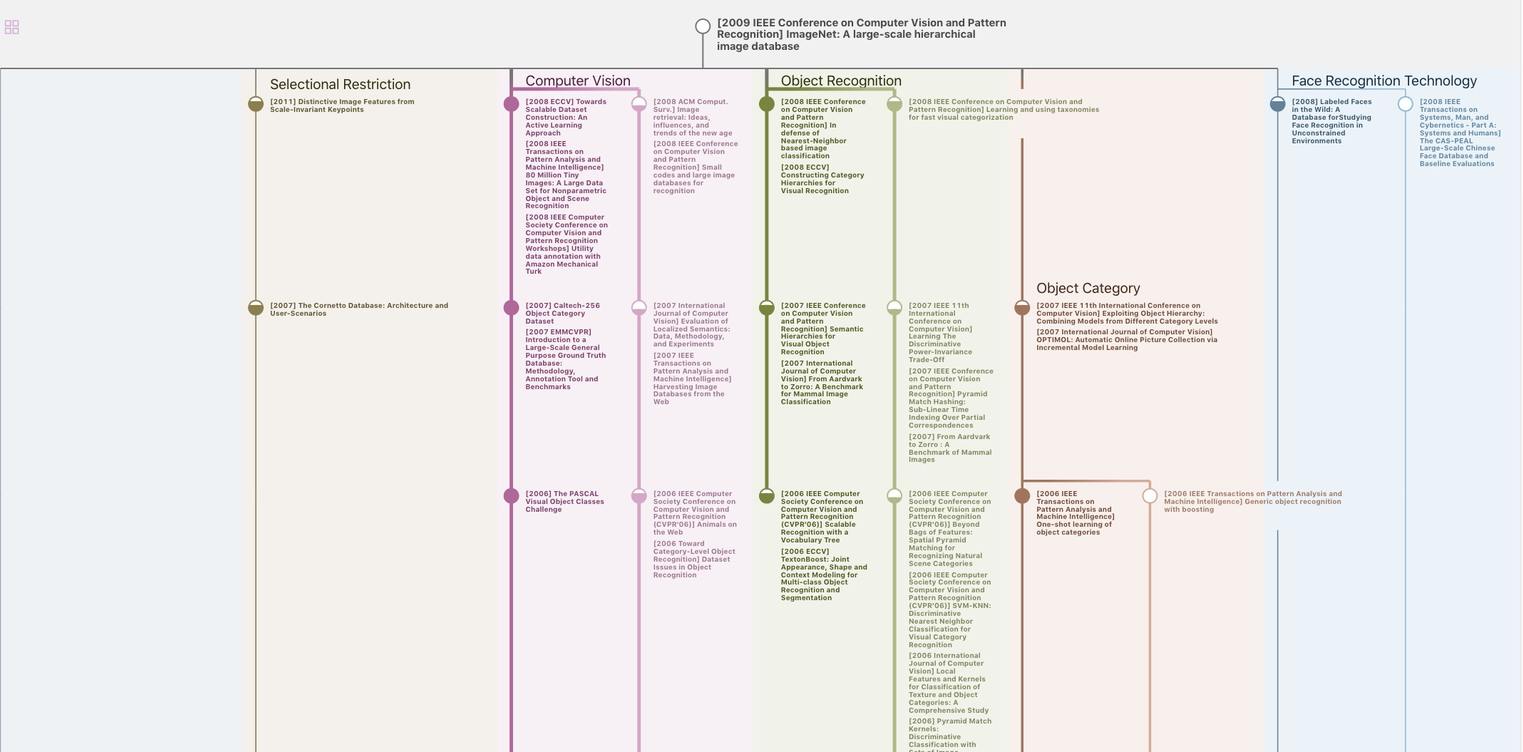
生成溯源树,研究论文发展脉络
Chat Paper
正在生成论文摘要