A high-fidelity digital twin predictive modeling of air-source heat pump using FCPM-SBLS algorithm
Journal of Building Engineering(2024)
摘要
This paper proposes an innovative Digital Twin (DT) model to predict air-source heat pumps' (ASHP) coefficient of performance (COP) using a combination of fuzzy (c + p) means clustering (FCPM) and the stacked broad learning system (SBLS) algorithm (FCPM-SBLS). The SBLS algorithm is adopted to establish the structure of the DT model, considering the imperative of tracking real-time performance changes in ASHP applications. To enhance the fitting and generalization ability of the DT model, FCPM is employed to partition the dataset into subclasses, allowing for the creation of sub-models specifically tailored to each subclass. Moreover, an incremental-full evolution strategy of the DT model is proposed to track changes in ASHP performance. The DT model's validation is conducted using data collected at a railway passenger station. Results demonstrate that the FCPM-SBLS method has commendable predictive performance, with respective mean absolute error (MAE), mean absolute percentage error (MAPE), and root mean square error (RMSE) values of 0.045, 0.012, and 0.252. Additionally, the proposed evolution strategy ensures timely updates for tracking ASHP performance changes. Through analysis of the evolution performance of DT model straddling over years, the updated model shows a 63.1% decrease in MAPE compared to the non-updated model. This study is not only conducive to advancing DT modeling in the field of HVAC systems, but also has practical significance for improving the energy efficiency and performance monitoring of ASHP.
更多查看译文
关键词
Digital twin,Heat pump,Model evolution,Fuzzy clustering
AI 理解论文
溯源树
样例
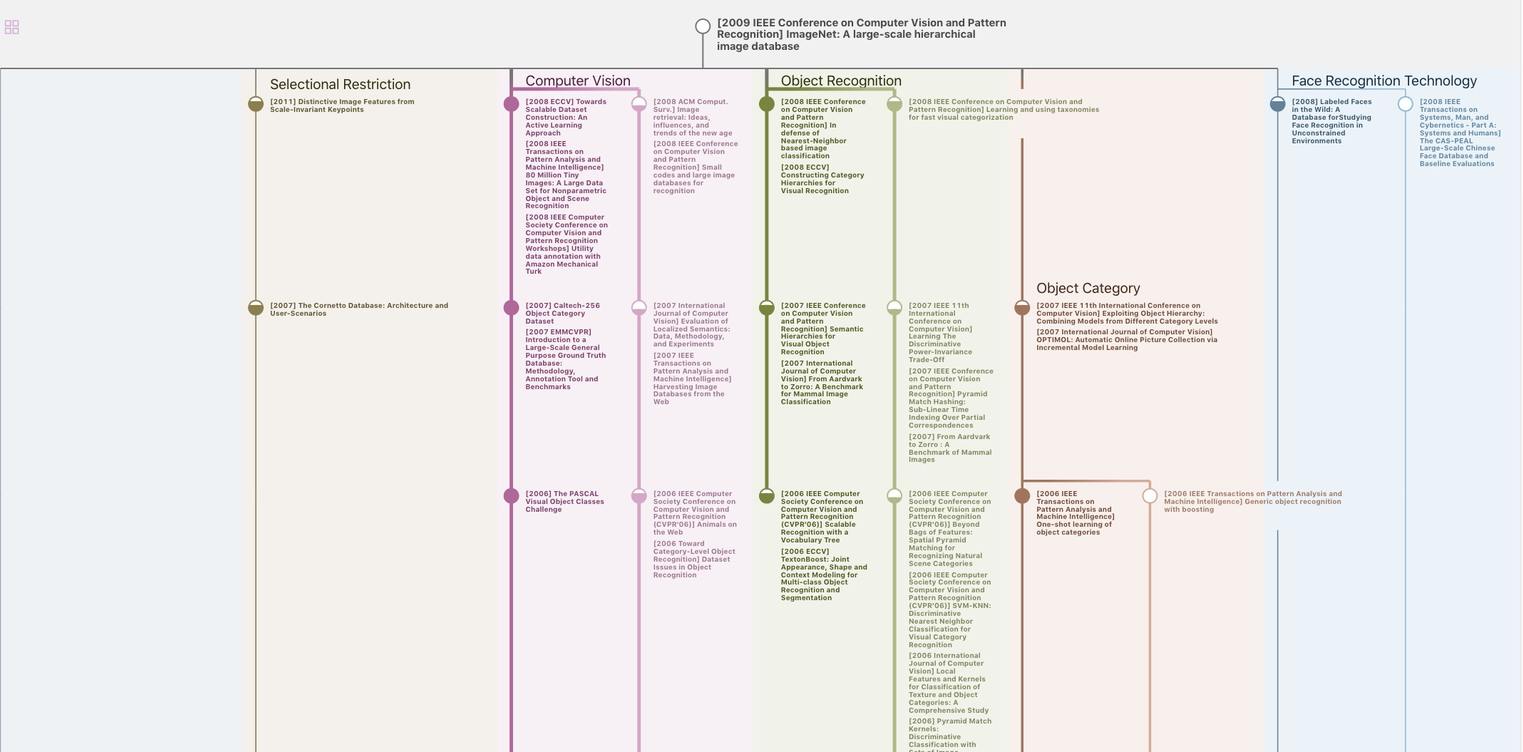
生成溯源树,研究论文发展脉络
Chat Paper
正在生成论文摘要