Self-Supervised Domain Exploration with an Optimal Transport Regularization for Open Set Cross-Domain Speech Emotion Recognition
ICASSP 2024 - 2024 IEEE International Conference on Acoustics, Speech and Signal Processing (ICASSP)(2024)
摘要
In the tasks of domain adaptation (DA) for speech emotion recognition (SER), self-supervised learning (SSL) algorithms could effectively explore domain and structural information from target domain samples, thereby mitigating domain discrepancies. However, in a general setting, when the target domain contains emotions that are never observed in the source domain, namely in open-set DA, existing SSL-based DA methods cannot maintain the robustness because of the interference of the extra unknown classes. To address this challenge, we propose the self-supervised domain exploration with an optimal transport (OT) regularization (SDEOTR) algorithm. First, we integrate the SSL algorithm into the SER model to mitigate the domain differences. Further, we categorize target domain samples into known and unknown groups based on the network’s prediction confidence. Finally, we employ OT to maximize the global probability distance between the two groups, aiming to decrease the impact of unknown emotions on the SER model. Cross-domain SER experimental results showed that our label-free SDEOTR significantly improved the performance of existing adaptive SER algorithms in open-set scenarios.
更多查看译文
关键词
Speech emotion recognition,open-set domain adaptation,optimal transport,self-supervised learning
AI 理解论文
溯源树
样例
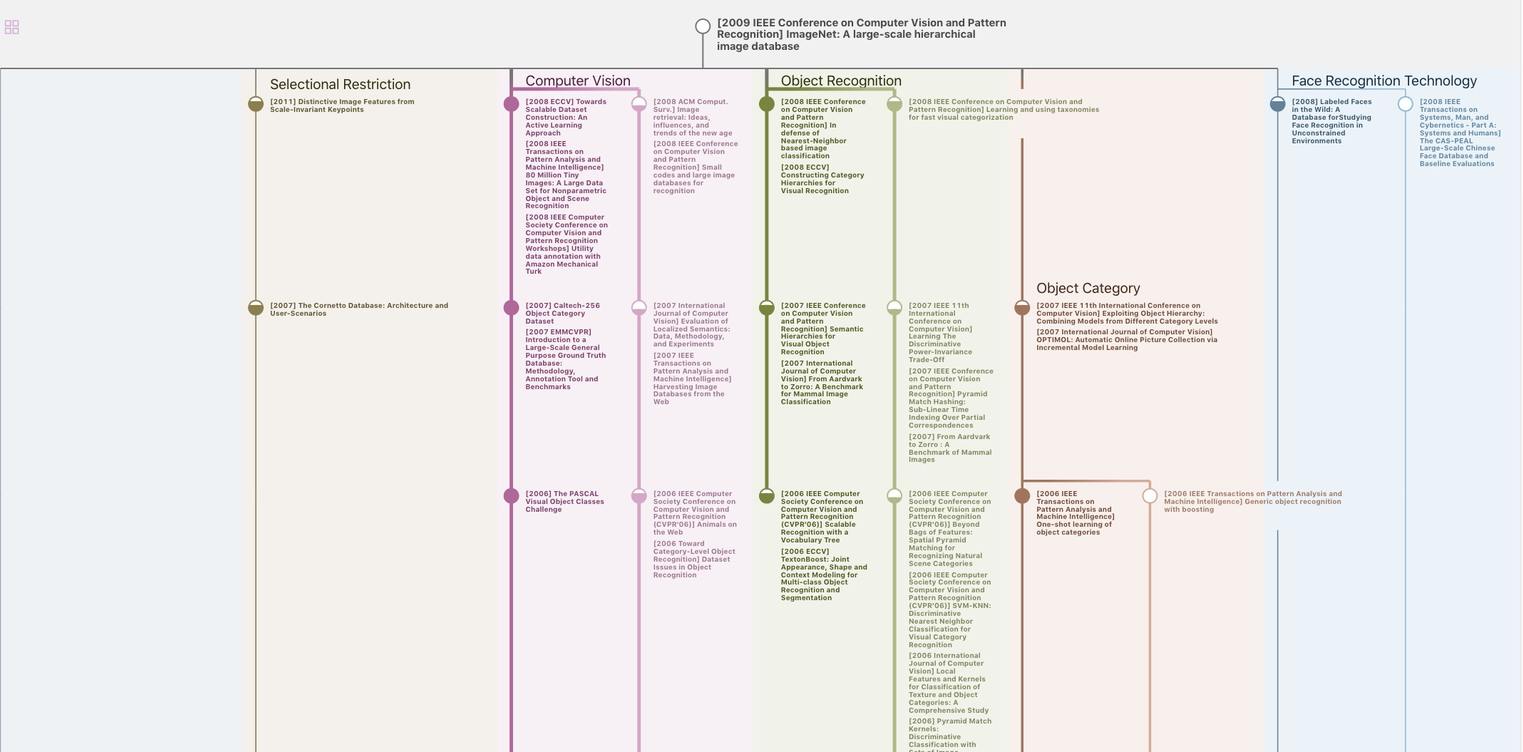
生成溯源树,研究论文发展脉络
Chat Paper
正在生成论文摘要