Yolo-3DMM for Simultaneous Multiple Object Detection and Tracking in Traffic Scenarios
IEEE TRANSACTIONS ON INTELLIGENT TRANSPORTATION SYSTEMS(2024)
摘要
Video-based multiple object tracking (MOT) is a fundamental task in intelligent transportation with applications ranging from automated traffic surveillance to autonomous driving. MOT methods commonly follow a tracking-by-detection paradigm, tracking objects by associating their detections across video frames. However, insofar, these methods have not used the entire vehicle trajectory motion characteristics to perform tracking, which converts the vehicle localization problem into a motion parameter estimation problem. Moreover, MOT methods mainly rely on off-the-shelf detectors. An independently trained detector is sub-optimal for the tracking-by-detection paradigm and adversely affects the overall system performance. In this article, we address these issues by proposing a novel MOT method for moving vehicles in traffic scenarios. Our tracker treats the vehicle tracks as unified 3D spatio-temporal trajectory instances and leverages the power of deep learning to extract vehicle motion from the 3D instances. We propose a new simultaneous detection and tracking network, called YOLO-3D Motion Model Network (Yolo-3DMM) that employs spatio-temporal features of traffic videos for simultaneous vehicle detection and tracking in an end-to-end manner. We adopt a variety of different vehicle tracking datasets to evaluate our method. Moreover, we also propose a tunnel MOT dataset from real highway tunnel surveillance in Guangdong, China to expand the experimental scenarios. To establish the efficacy of our method, we evaluate it on 100 different roadside traffic scenarios. Our method shows excellent performance on UA-DETRAC and Omni-MOT datasets. It achieves a PR-MOTA score of 29.40% on UA-DETRAC and gets a 69.7% MOTA score on the Omni-MOT dataset.
更多查看译文
关键词
Multi-object tracking,simultaneous detection and tracking,tracking by detection,motion-based prediction
AI 理解论文
溯源树
样例
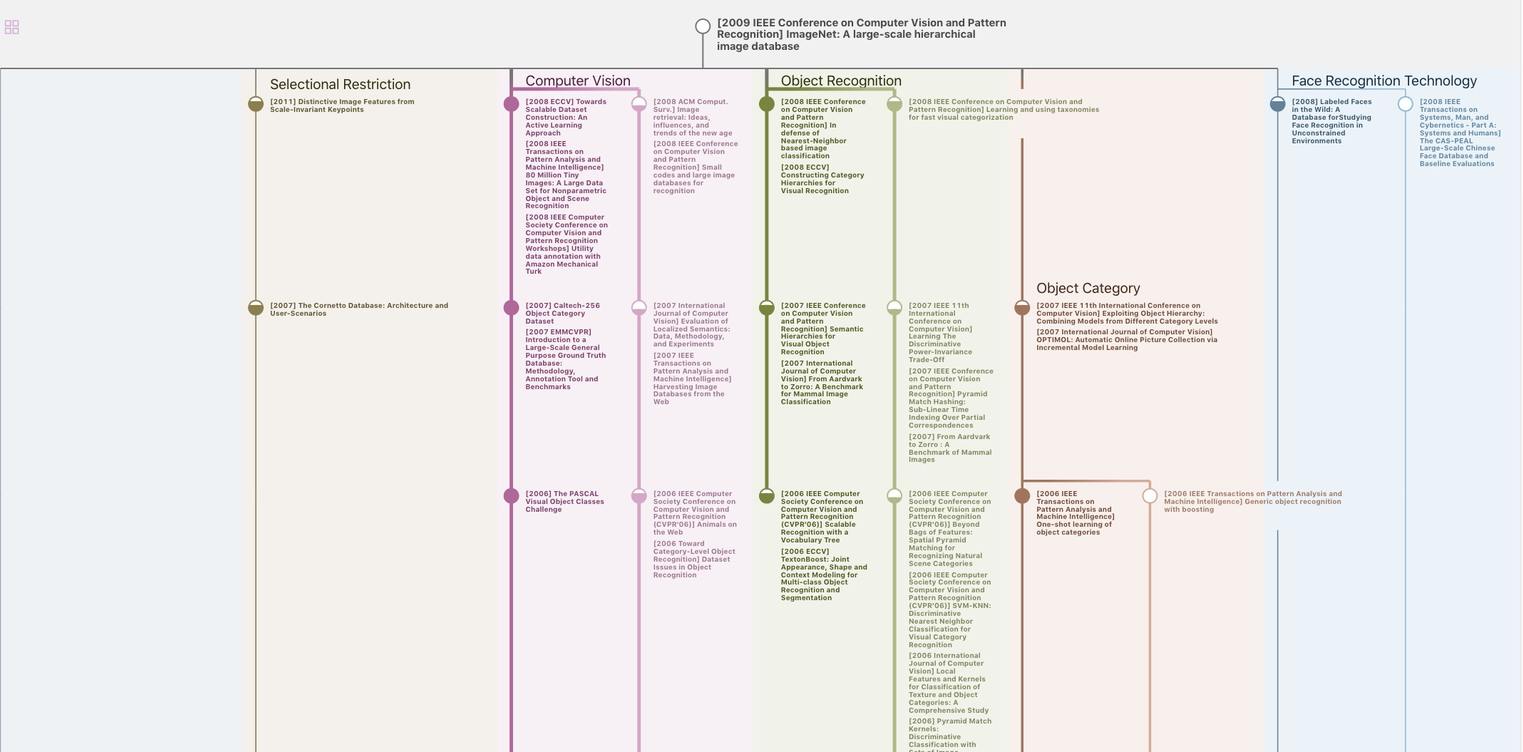
生成溯源树,研究论文发展脉络
Chat Paper
正在生成论文摘要