Enhanced Event-Based Video Reconstruction with Motion Compensation
arxiv(2024)
摘要
Deep neural networks for event-based video reconstruction often suffer from a
lack of interpretability and have high memory demands. A lightweight network
called CISTA-LSTC has recently been introduced showing that high-quality
reconstruction can be achieved through the systematic design of its
architecture. However, its modelling assumption that input signals and output
reconstructed frame share the same sparse representation neglects the
displacement caused by motion. To address this, we propose warping the input
intensity frames and sparse codes to enhance reconstruction quality. A
CISTA-Flow network is constructed by integrating a flow network with CISTA-LSTC
for motion compensation. The system relies solely on events, in which predicted
flow aids in reconstruction and then reconstructed frames are used to
facilitate flow estimation. We also introduce an iterative training framework
for this combined system. Results demonstrate that our approach achieves
state-of-the-art reconstruction accuracy and simultaneously provides reliable
dense flow estimation. Furthermore, our model exhibits flexibility in that it
can integrate different flow networks, suggesting its potential for further
performance enhancement.
更多查看译文
AI 理解论文
溯源树
样例
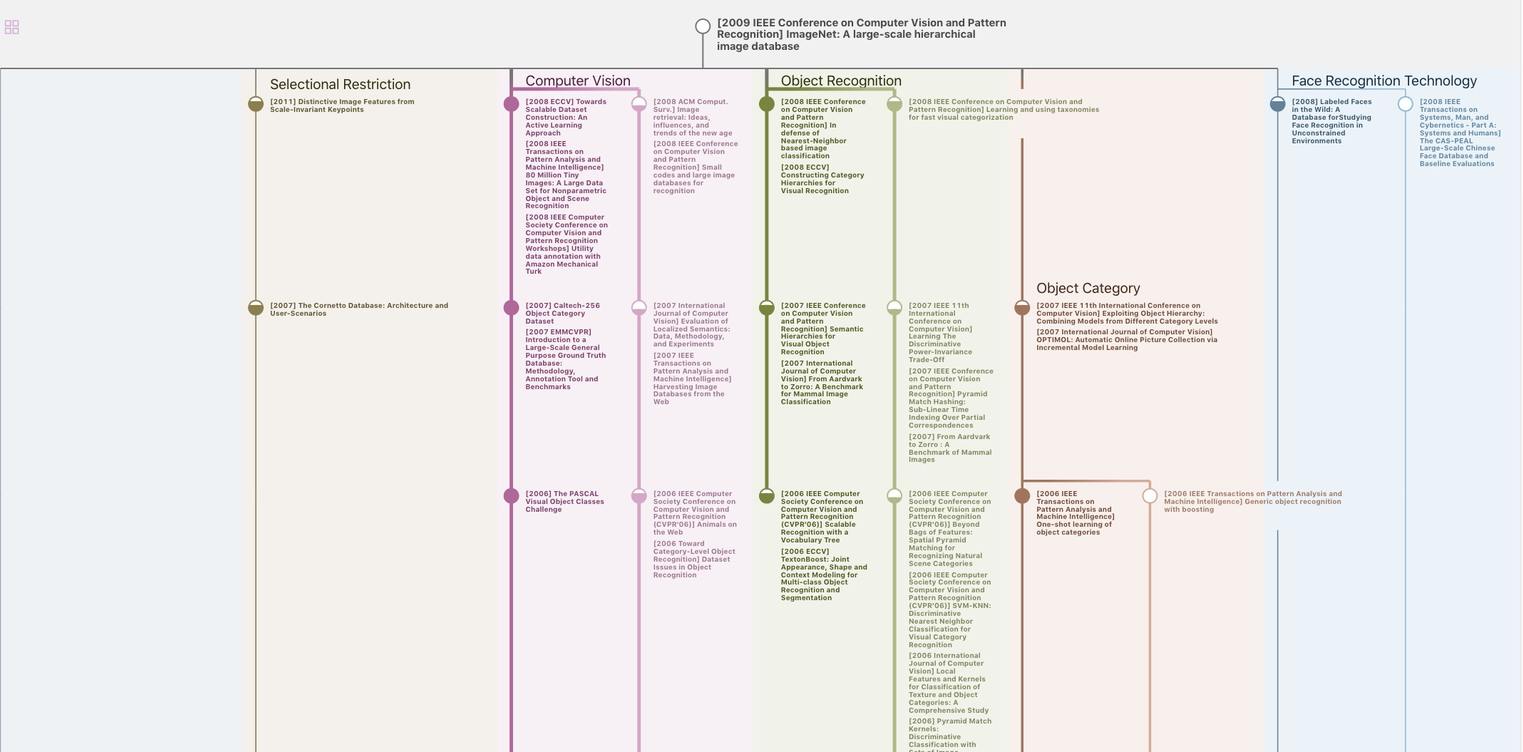
生成溯源树,研究论文发展脉络
Chat Paper
正在生成论文摘要