Single-Agent Actor Critic for Decentralized Cooperative Driving
arxiv(2024)
摘要
Active traffic management incorporating autonomous vehicles (AVs) promises a
future with diminished congestion and enhanced traffic flow. However,
developing algorithms for real-world application requires addressing the
challenges posed by continuous traffic flow and partial observability. To
bridge this gap and advance the field of active traffic management towards
greater decentralization, we introduce a novel asymmetric actor-critic model
aimed at learning decentralized cooperative driving policies for autonomous
vehicles using single-agent reinforcement learning. Our approach employs
attention neural networks with masking to handle the dynamic nature of
real-world traffic flow and partial observability. Through extensive
evaluations against baseline controllers across various traffic scenarios, our
model shows great potential for improving traffic flow at diverse bottleneck
locations within the road system. Additionally, we explore the challenge
associated with the conservative driving behaviors of autonomous vehicles that
adhere strictly to traffic regulations. The experiment results illustrate that
our proposed cooperative policy can mitigate potential traffic slowdowns
without compromising safety.
更多查看译文
AI 理解论文
溯源树
样例
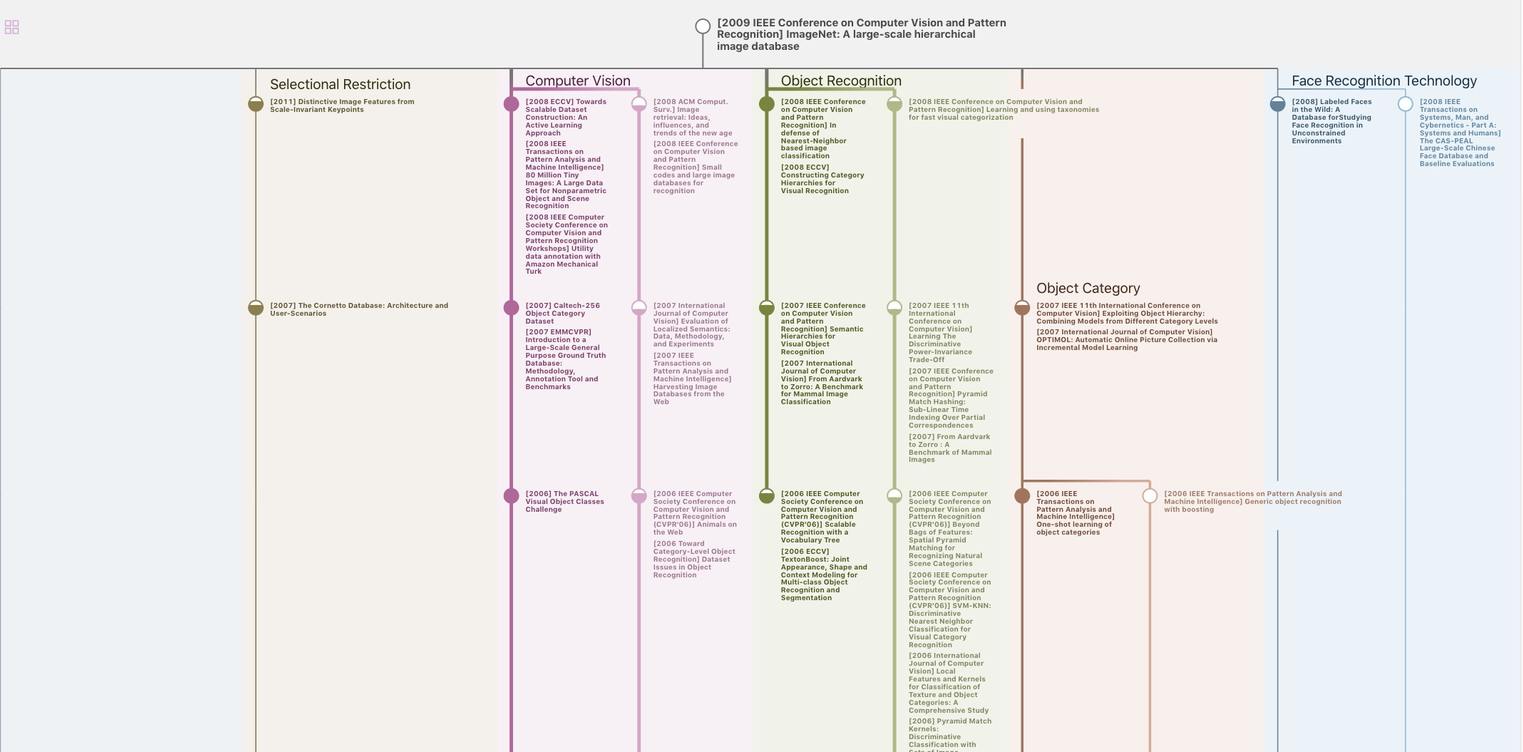
生成溯源树,研究论文发展脉络
Chat Paper
正在生成论文摘要