Max-Margin Transducer Loss: Improving Sequence-Discriminative Training Using a Large-Margin Learning Strategy
ICASSP 2024 - 2024 IEEE International Conference on Acoustics, Speech and Signal Processing (ICASSP)(2024)
摘要
In this work, we propose a novel sequence-discriminative training criterion for automatic speech recognition (ASR) based on the Conformer Transducer. Inspired by the large-margin classifier framework, we separate the "good" and the "bad" hypotheses in an N-best list produced from a pre-trained transducer model by a margin (τ), hence the term, Max-Margin Transducer (MMT) loss. It is observed that fine-tuning with the proposed loss achieves significant improvement over baseline transducer loss but does not outperform the state-of-the-art minimum word error rate (MWER) training. However, combining the proposed MMT loss with MWER surpasses the performance of either losses suggesting the complimentary nature of MWER and MMT losses. With the combined losses, we obtained 7.44% and 7.68% relative WER improvements on Librispeech test-clean and test-other sets, respectively, and up to 8.9% relative improvement on Multi-lingual Librispeech test sets.
更多查看译文
关键词
Max-margin,Conformer,minimum word error rate training,sequence discriminative criterion,end-to-end speech recognition models
AI 理解论文
溯源树
样例
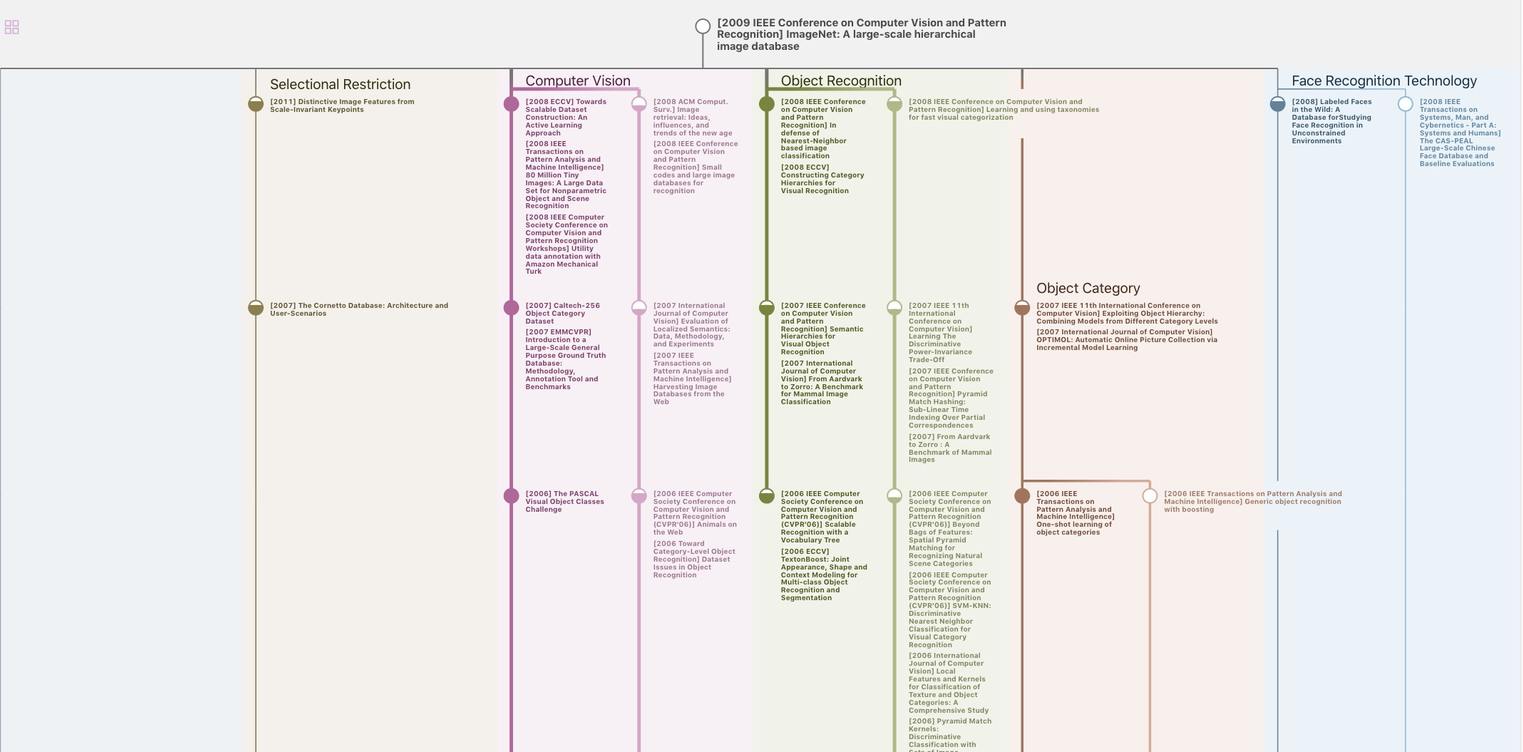
生成溯源树,研究论文发展脉络
Chat Paper
正在生成论文摘要